Energy Minimization with Secrecy Provisioning in Federated Learning-assisted Marine Digital Twin Networks
ICC 2023 - IEEE INTERNATIONAL CONFERENCE ON COMMUNICATIONS(2023)
摘要
Digital twin has been emerging as a promising paradigm that connects the physical entities and digital space, and continuously evolves and optimizes the physical systems. In this paper, we focus on studying the efficient data communication and computation when constructing the marine digital twin network with secrecy provisioning. Specifically, we leverage the federated learning (FL) to train the digital twin model. In the process of FL, all unmanned surface vehicles (USVs) deliver the trained models with non-orthogonal multiple access (NOMA) to the high altitude platform (HAP) for the global model aggregation. Considering the possible eavesdropping on the HAP, we utilize the chaotic sequences to spread the model information during the global model broadcasting. In this framework, we further want to minimize the total energy consumption of completing the digital twin training by jointly optimizing the global accuracy, local accuracy, HAP's transmission power, and model uploading duration subject to the secrecy provisioning and latency constraint. Despite the non-convexity, we propose a low-complexity search algorithm (LCS-Algorithm) to solve this joint optimization problem. Finally, the numerical results validate the performance of the proposed algorithm in terms of optimality and time efficiency.
更多查看译文
AI 理解论文
溯源树
样例
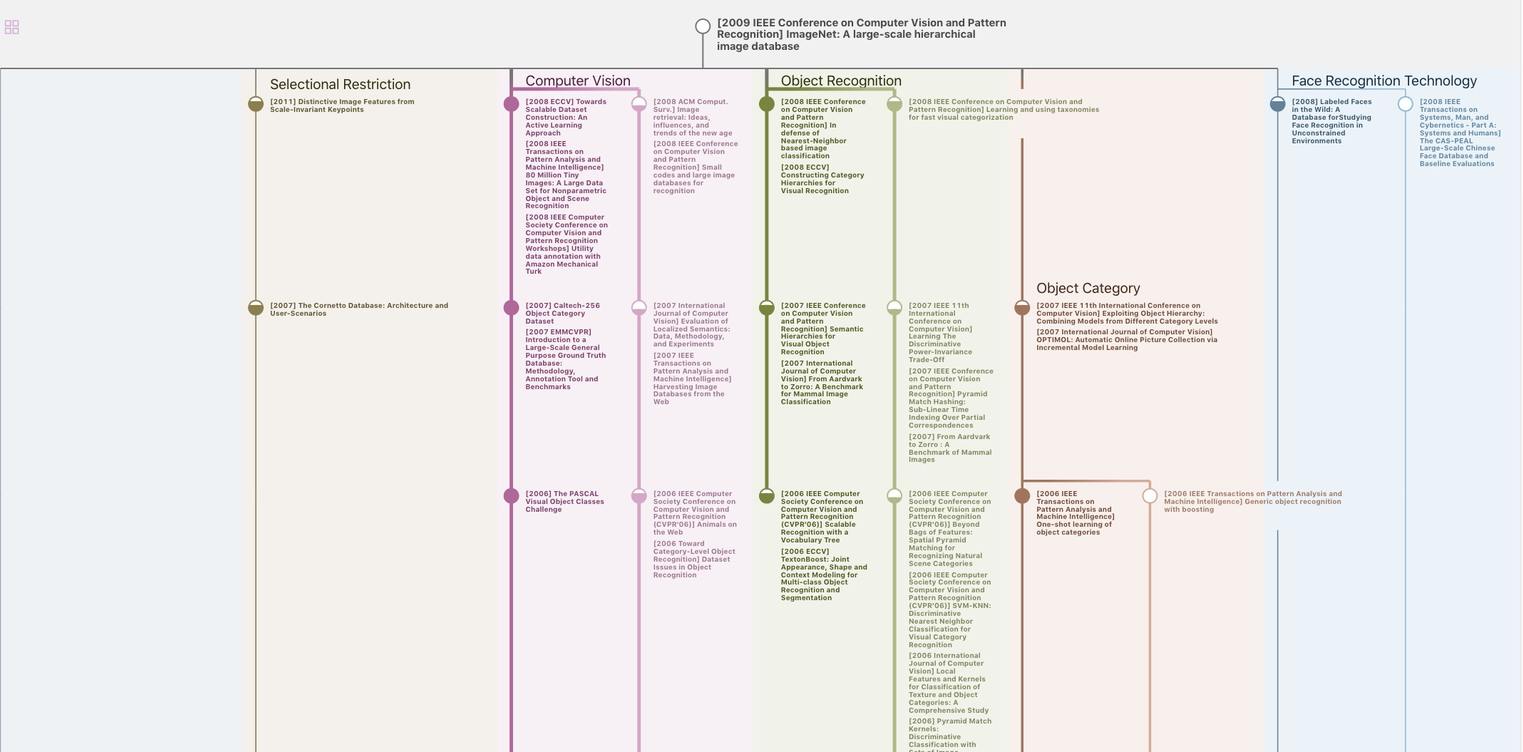
生成溯源树,研究论文发展脉络
Chat Paper
正在生成论文摘要