Social Networks based Robust Federated Learning for Encrypted Traffic Classification
ICC 2023 - IEEE INTERNATIONAL CONFERENCE ON COMMUNICATIONS(2023)
摘要
The encrypted traffic classification based on federated learning has become one of the key concerns since it can effectively provide expansion and privacy protection for traffic dataset. However, existing classification models suffer from low robustness and slow convergence in the presence of abnormal traffic data on the client side. We note that the clients participating in the training are operated by humans in social networks, and their communication with each other generate social traffic. By introducing the traffic data into the federated learning classification model, the correlation between the respective small model parameters of the clients can be increased, which can be leveraged to quickly detect abnormal clients and improve the model performance. The effectiveness of the scheme is verified on a classical public dataset and the results show that this WS network structure converges the fastest and the degree distribution has an overall linear relationship with the convergence speed. Our scheme is still highly robust with abnormal data and the model convergence speed is significantly better than other methods. Compared with the existing method, the model of social networks based classification of federated encrypted traffic has 2.5% higher accuracy, 6.1% higher recall, and more than 39.3% fewer communication rounds, respectively.
更多查看译文
关键词
social networks,encrypted traffic classification,federated learning,robustness
AI 理解论文
溯源树
样例
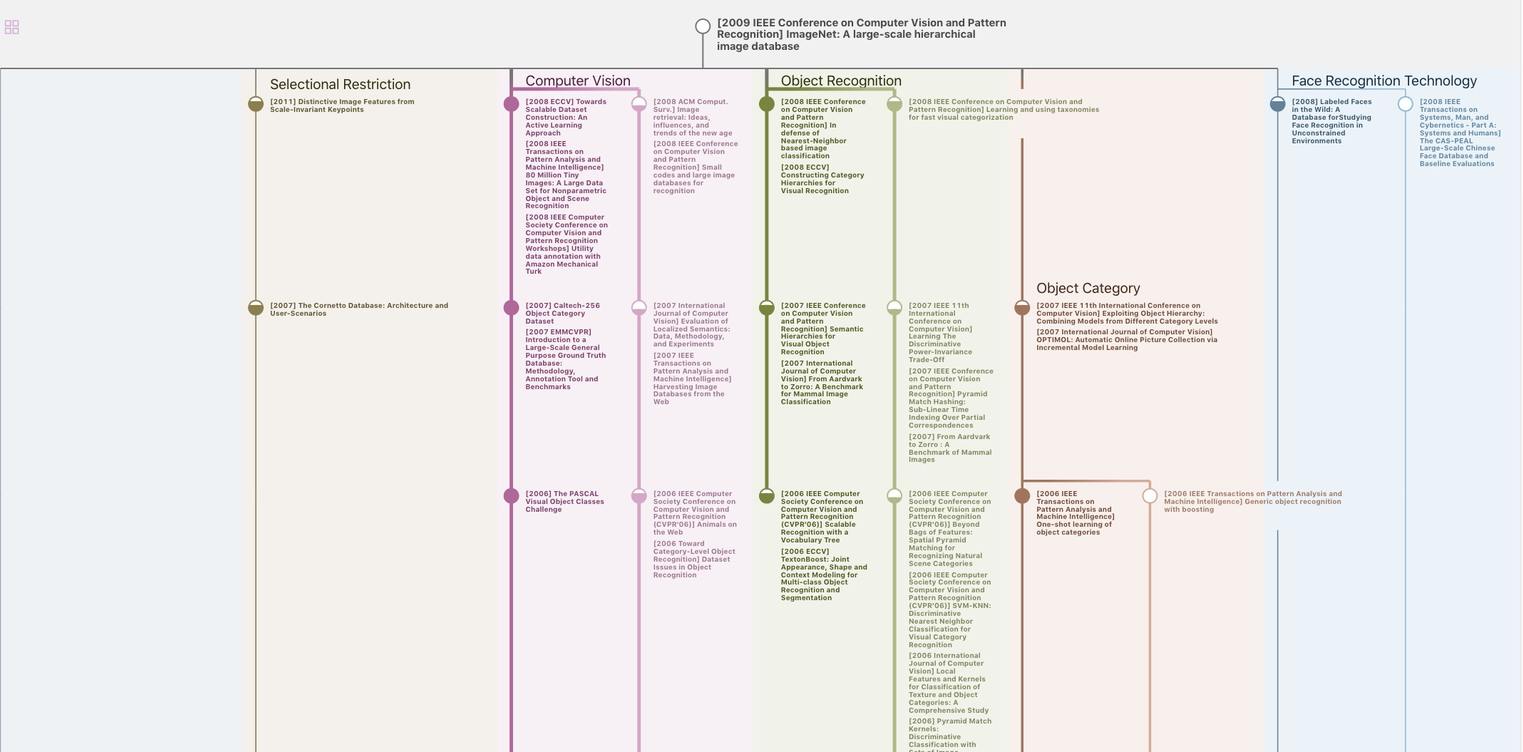
生成溯源树,研究论文发展脉络
Chat Paper
正在生成论文摘要