Scalable Multi-Agent Reinforcement Learning-Based Distributed Channel Access.
ICC 2023-IEEE INTERNATIONAL CONFERENCE ON COMMUNICATIONS(2023)
摘要
With the rapid development of smart devices, the next generation wireless networks (NGWNs) are expected to achieve high access efficiency in a high-dynamic scenario. To tackle the above challenges in NGWNs, this paper proposes a new MAC protocol, MAAC-advanced Listen-Before-Talk (MLBT), which employs multi-agent reinforcement learning (MARL) algorithm. As a MARL paradigm, centralized training with decentralized execution (CTDE) is confronted with the scalability issue. To address it, we design a scalable neural network architecture based on the attention mechanism, which can cope with the varying number of stations. Moreover, a novel reward function is designed to achieve the max-min fairness and maximum aggregate network throughput simultaneously. Extensive simulation experiments are provided to show that MLBT approaches the optimal performance and accelerates the centralized training process when stations join or leave the network.
更多查看译文
关键词
centralized training process,decentralized execution,high access efficiency,high-dynamic scenario,MAAC-advanced Listen-Before-Talk,MAC protocol,MARL paradigm,maximum aggregate network,MLBT,multiagent reinforcement learning algorithm,NGWNs,scalability issue,scalable multiagent reinforcement learning,scalable neural network architecture,smart devices
AI 理解论文
溯源树
样例
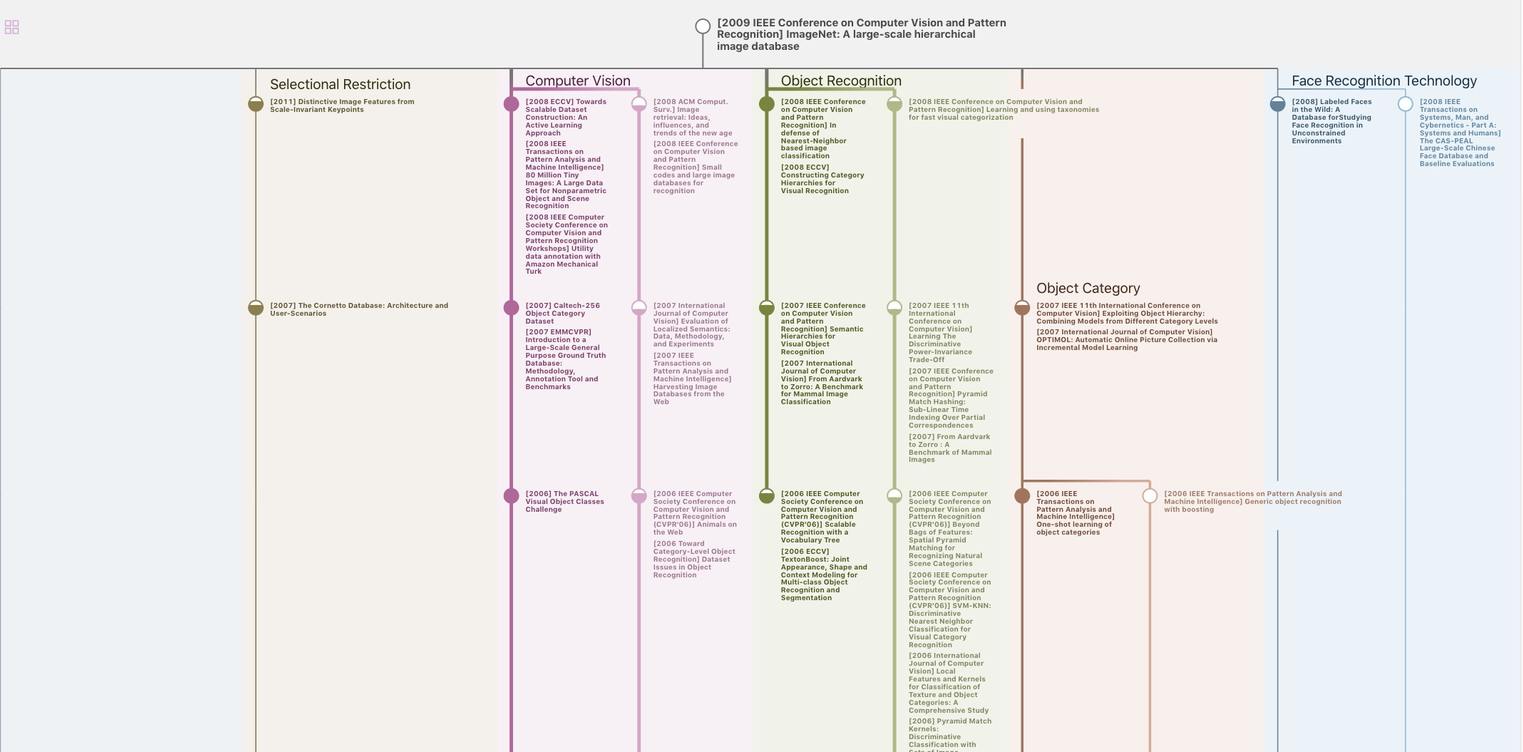
生成溯源树,研究论文发展脉络
Chat Paper
正在生成论文摘要