Hierarchical Vision Transformers for Disease Progression Detection in Chest X-Ray Images
MEDICAL IMAGE COMPUTING AND COMPUTER ASSISTED INTERVENTION, MICCAI 2023, PT V(2023)
摘要
Chest radiography is a commonly used diagnostic imaging exam for monitoring disease progression and treatment effectiveness. While machine learning has made significant strides in tasks such as image segmentation, disease diagnosis, and automatic report generation, more intricate tasks such as disease progression monitoring remain fairly underexplored. This task presents a formidable challenge because of the complex and intricate nature of disease appearances on chest X-ray images, which makes distinguishing significant changes from irrelevant variations between images challenging. Motivated by these challenges, this work proposes CheXRelFormer, an end-to-end siamese Transformer disease progression model that takes a pair of images as input and detects whether the patient's condition has improved, worsened, or remained unchanged. The model comprises two hierarchical Transformer encoders, a difference module that compares feature differences across images, and a final classification layer that predicts the change in the patient's condition. Experimental results demonstrate that CheXRelFormer outperforms previous counterparts. Code is available at https://github.com/PLAN-Lab/CheXRelFormer.
更多查看译文
关键词
Vision Transformers,Disease Progression,Chest X-Ray Comparison Relations,Longitudinal CXR Relationships
AI 理解论文
溯源树
样例
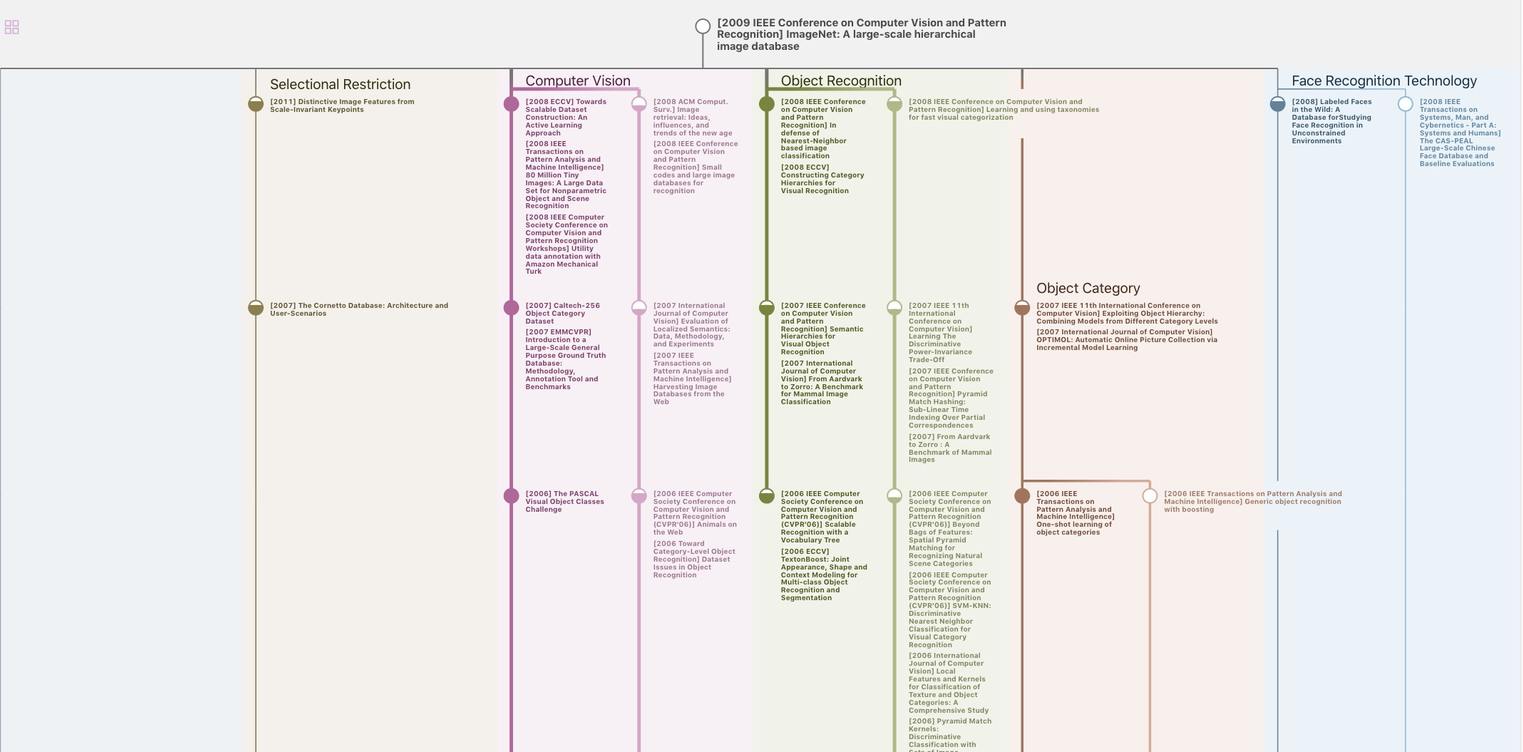
生成溯源树,研究论文发展脉络
Chat Paper
正在生成论文摘要