Image-to-Image Translation Between Tau Pathology and Neuronal Metabolism PET in Alzheimer Disease with Multi-domain Contrastive Learning
MACHINE LEARNING IN CLINICAL NEUROIMAGING, MLCN 2023(2023)
摘要
Alzheimer Disease (AD) is delineated by the presence of amyloid (A) and tau (T) pathology, with variable levels of neurodegeneration (N). While the relationship between tau and neuronal hypometabolism with positron emission tomography (PET) has been studied by T/N regression models, there has been limited application of image-to-image translation to compare between AD biomarker domains. We optimize a contrastive learning (CL) generative adversarial network for translation between paired tau and 18F-Fluorodeoxyglucose (18F-FDG) images from 172 older adults in an AD study. By comparing output domain query patches with positive and negative patches from input and target domains in latent space, the multi-domain CL loss approximates a cross entropy between latent output and a mixture of both original data distributions. Aligned with theory, CL model performance varies empirically based on use of dual vs. single domain CL loss, diversity of training data and negative patches, and CL temperature. Translation mapping yields realistic and accurate T and N images while reconstruction error validates regional relationships of T/N decoupling observed in regression models. Critically, biomarkers of non-AD pathology correlate with real and translated N images more than with input T images, suggesting our model imparts knowledge of non-AD influences on T/N relationships. Collectively, we present a proof-of-principle multi-domain CL approach to translate between clinical tau and 18F-FDG PET, study mismatch between T and cellular responses and reveal patterns in AD vs. non-AD heterogeneity.
更多查看译文
关键词
Image Translation,Contrastive Learning,PET,Alzheimer Disease
AI 理解论文
溯源树
样例
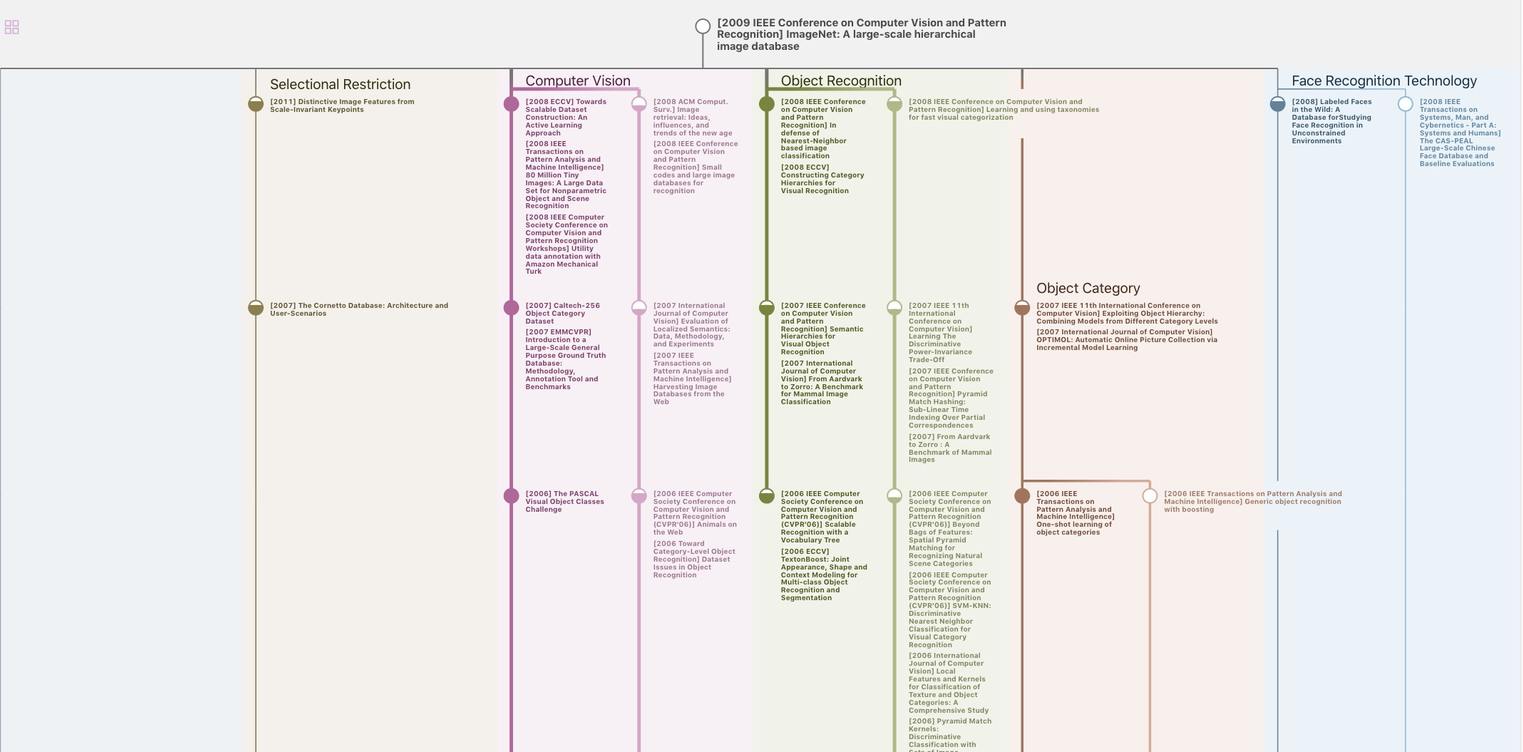
生成溯源树,研究论文发展脉络
Chat Paper
正在生成论文摘要