Federated Condition Generalization on Low-dose CT Reconstruction via Cross-domain Learning
MEDICAL IMAGE COMPUTING AND COMPUTER ASSISTED INTERVENTION, MICCAI 2023, PT III(2023)
摘要
The harmful radiation dose associated with CT imaging is a major concern because it can cause genetic diseases. Acquiring CT data at low radiation doses has become a pressing goal. Deep learning (DL)-based methods have proven to suppress noise-induced artifacts and promote image quality in low-dose CT imaging. However, it should be noted that most of the DL-based methods are constructed based on the CT data from a specific condition, i.e., specific imaging geometry and specific dose level. Then these methods might generalize poorly to the other conditions, i.e., different imaging geometries and other radiation doses, due to the big data heterogeneity. In this study, to address this issue, we propose a condition generalization method under a federated learning framework (FedCG) to reconstruct CT images on two conditions: three different dose levels and different sampling shcemes at three different geometries. Specifically, the proposed FedCG method leverages a cross-domain learning approach: individual-client sinogram learning and cross-client image reconstruction for condition generalization. In each individual client, the sinogram at each condition is processed similarly to that in the iRadonMAP. Then the CT images at each client are learned via a condition generalization network in the server which considers latent common characteristics in the CT images at all conditions and preserves the client-specific characteristics in each condition. Experiments show that the proposed FedCG outperforms the other competing methods on two imaging conditions in terms of qualitative and quantitative assessments.
更多查看译文
关键词
low-dose CT,image reconstruction,federal learning,generalization,generalization
AI 理解论文
溯源树
样例
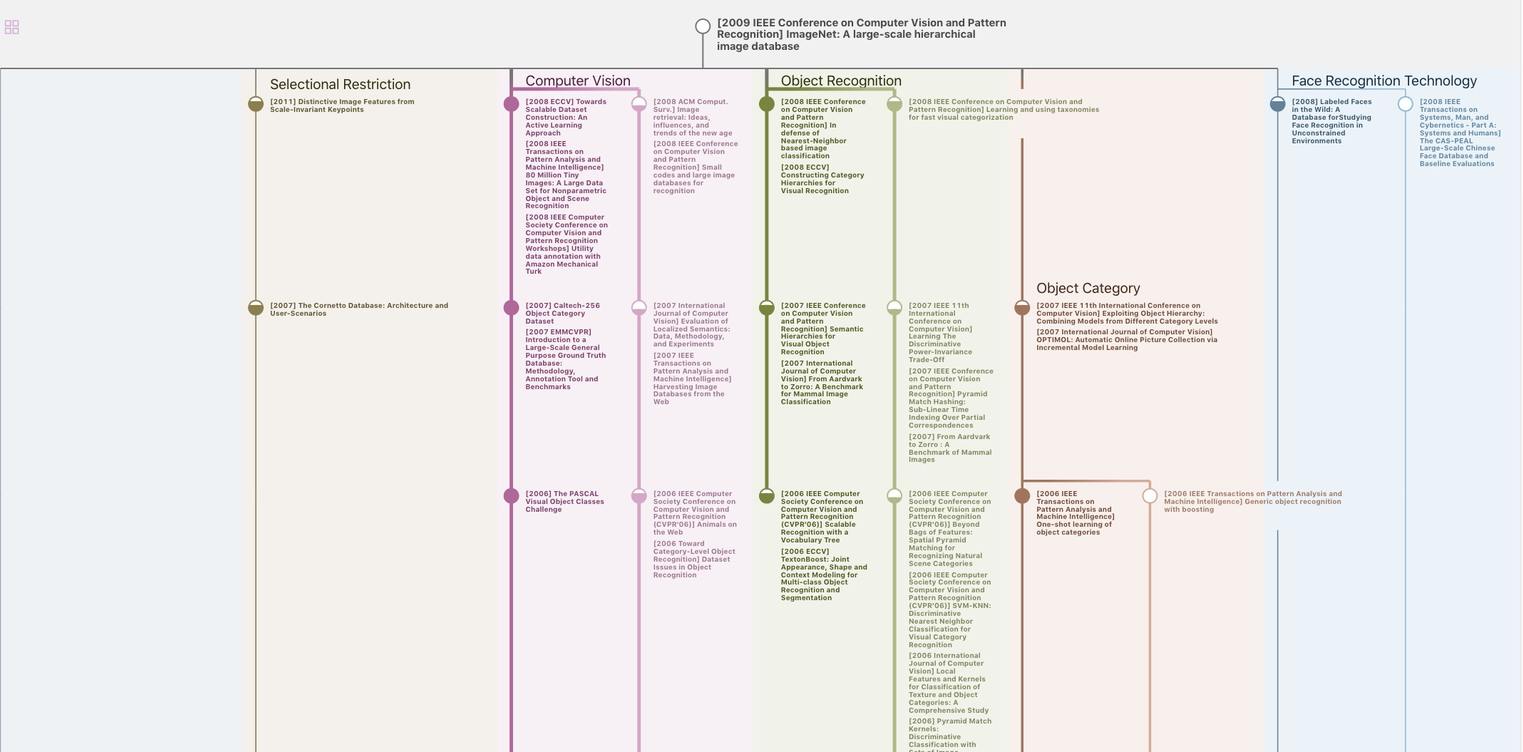
生成溯源树,研究论文发展脉络
Chat Paper
正在生成论文摘要