Physics-guided Graph Diffusion Network for Combining Heterogeneous Simulated Data: An Application in Predicting Stream Water Temperature.
SDM(2023)
摘要
This paper introduces a new method for combining simulated data over different types of nodes in heterogeneous graphs to facilitate predictive learning. Simulation has been widely used in scientific domains to mitigate the need for a large number of observation samples. However, simulated data are often created separately for each type of physical systems while interactions amongst different types of systems remain unexplored. Our method is developed in the context of predicting water temperature in stream networks, which is critical for decision making in water management. In particular, we first develop a graph diffusion network (GDN) to model the interactions amongst stream segments and reservoirs in a heterogeneous graph. We use the GDN model to combine simulated data for both streams and reservoirs in the graph, and use the obtained composite simulations to train the GDN model in a semi-supervised manner. Then the GDN model is further fine-tuned using true observations. Since observation data are often sparse and localized, we further leverage the information from simulations to build a reweighting strategy so as to migitage the discrepancy between training and testing data. Our evaluations in the Delaware River Basin have shown the superiority of the proposed method over multiple baselines using either sparse or localized training data. The proposed GDN model also creates a better composite simulation dataset for heterogeneous graphs.
更多查看译文
关键词
graph diffusion network,heterogeneous simulated data,physics-guided
AI 理解论文
溯源树
样例
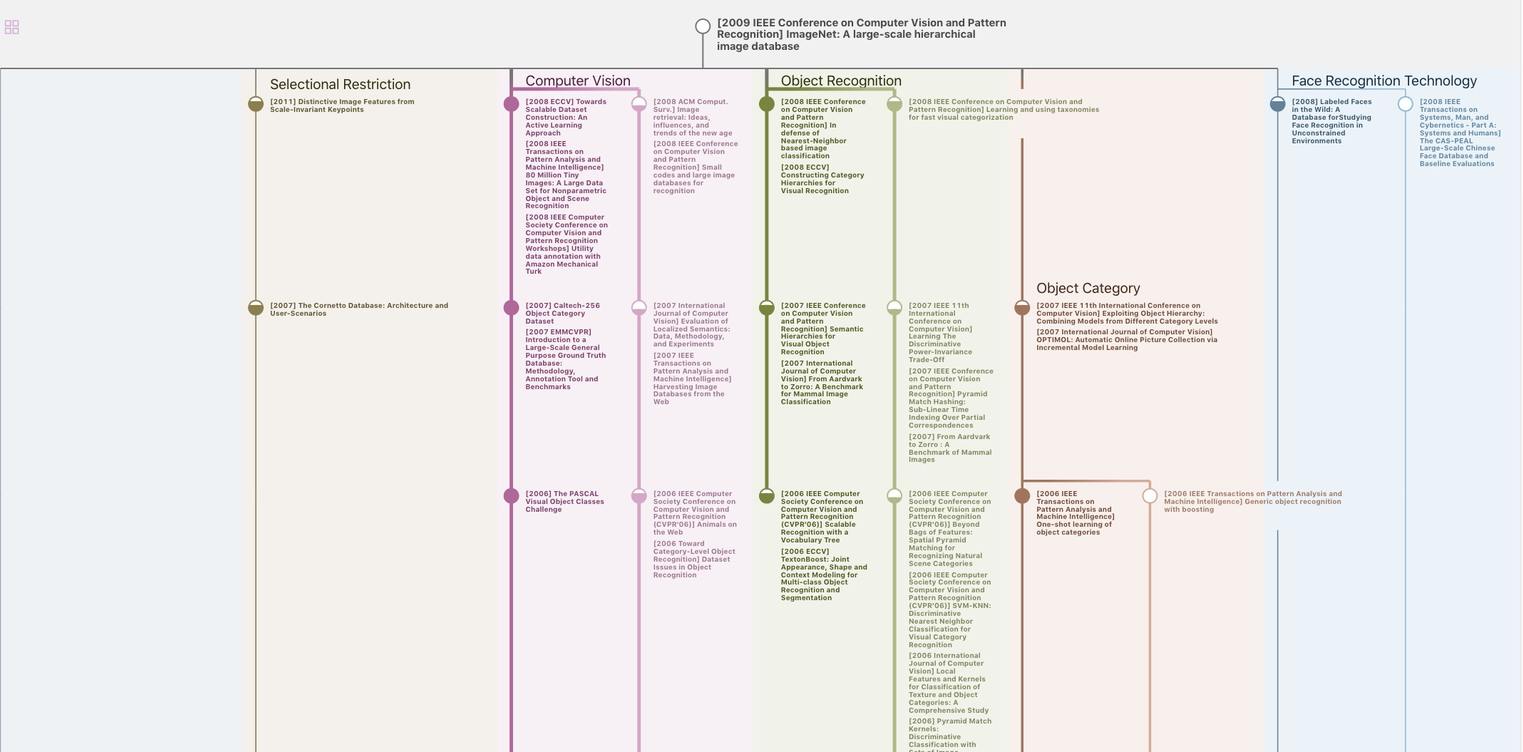
生成溯源树,研究论文发展脉络
Chat Paper
正在生成论文摘要