Deep Cellular Embeddings: An Explainable Plug and Play Improvement for Feature Representation in Histopathology
MEDICAL IMAGE COMPUTING AND COMPUTER ASSISTED INTERVENTION, MICCAI 2023, PT VI(2023)
摘要
Weakly supervised classification of whole slide images (WSIs) in digital pathology typically involves making slide-level predictions by aggregating predictions from embeddings extracted from multiple individual tiles. However, these embeddings can fail to capture valuable information contained within the individual cells in each tile. Here we describe an embedding extraction method that combines tile-level embeddings with a cell-level embedding summary. We validated the method using four hematoxylin and eosin stained WSI classification tasks: human epidermal growth factor receptor 2 status and estrogen receptor status in primary breast cancer, breast cancer metastasis in lymph node tissue, and cell of origin classification in diffuse large B-cell lymphoma. For all tasks, the new method outperformed embedding extraction methods that did not include cell-level representations. Using the publicly available HEROHE Challenge data set, the method achieved a state-of-the-art performance of 90% area under the receiver operating characteristic curve. Additionally, we present a novel model explainability method that could identify cells associated with different classification groups, thus providing supplementary validation of the classification model. This deep learning approach has the potential to provide morphological insights that may improve understanding of complex underlying tumor pathologies.
更多查看译文
关键词
Deep Learning,Whole Slide Images,Hematoxylin and Eosin
AI 理解论文
溯源树
样例
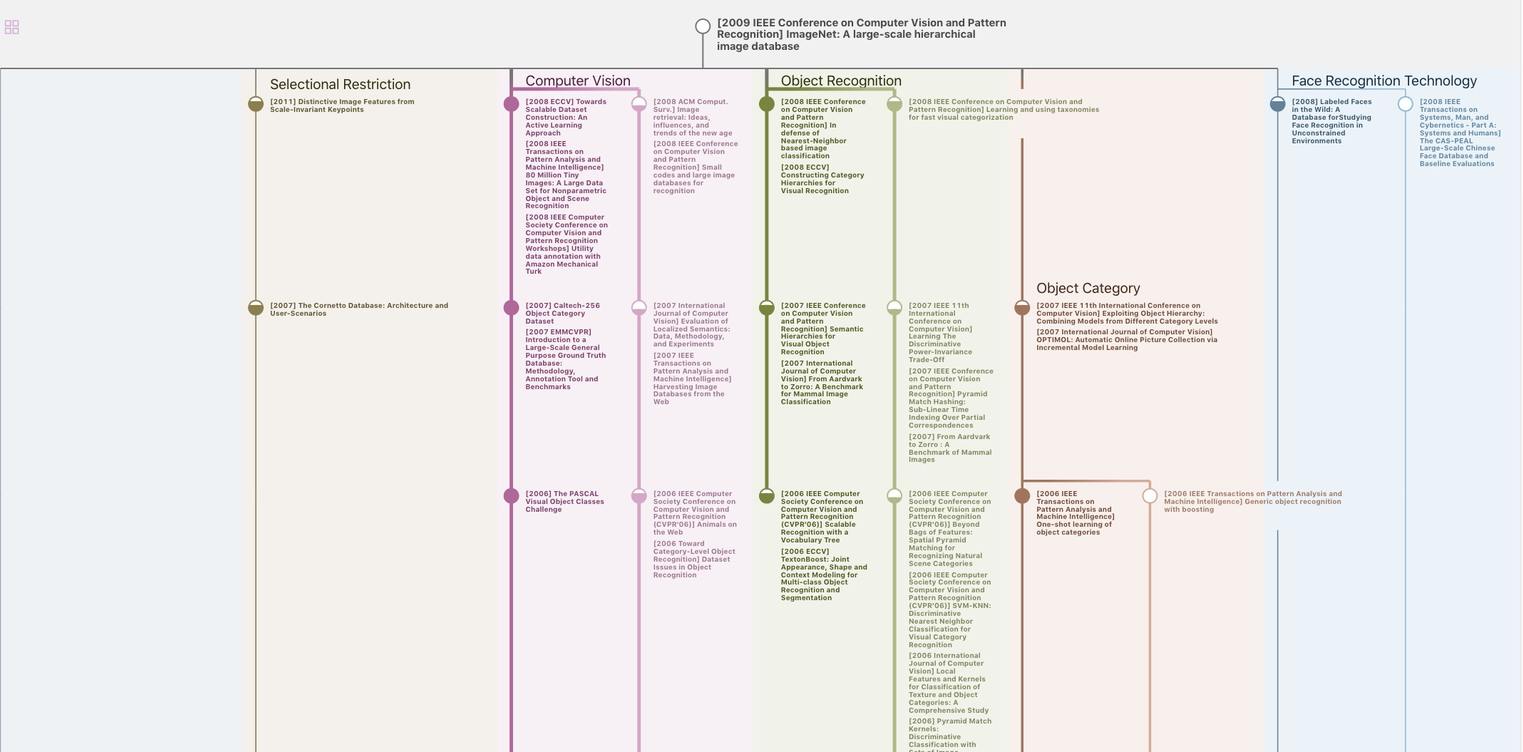
生成溯源树,研究论文发展脉络
Chat Paper
正在生成论文摘要