Proper Scoring Loss Functions Are Simple and Effective for Uncertainty Quantification of White Matter Hyperintensities
UNCERTAINTY FOR SAFE UTILIZATION OF MACHINE LEARNING IN MEDICAL IMAGING, UNSURE 2023(2023)
摘要
Uncertainty quantification is an important tool for improving the trustworthiness and clinical usefulness of medical imaging segmentation models, and many techniques exist for quantifying segmentation uncertainty. However, popular segmentation losses such as Dice loss lead to poorly calibrated models and silent failure in uncertainty maps. We compare common proper scoring rule based losses, which encourage well-calibrated models, to Dice loss and calibrated Dice loss variants, for white matter hyperintensity (WMH) segmentation in FLAIR and T1w MRI. We show that scoring rules yield strong performance (e.g., Spherical-TopK: Dice of 0.763, vs 0.717 for Dice loss) and low WMH instance detection failure rate in axial slices (Logarithmic yields 11% missing instances in the uncertainty map vs 28% for Dice). Furthermore, proper scoring rule methods do not exhibit the performance degradation in calibration error and WMH burden prediction of Dice loss in low WMH burden patients. Finally, we show temperature scaling is insufficient to overcome the drawbacks of Dice loss.
更多查看译文
关键词
Scoring Functions,Uncertainty Quantification,White Matter Hyperintensities
AI 理解论文
溯源树
样例
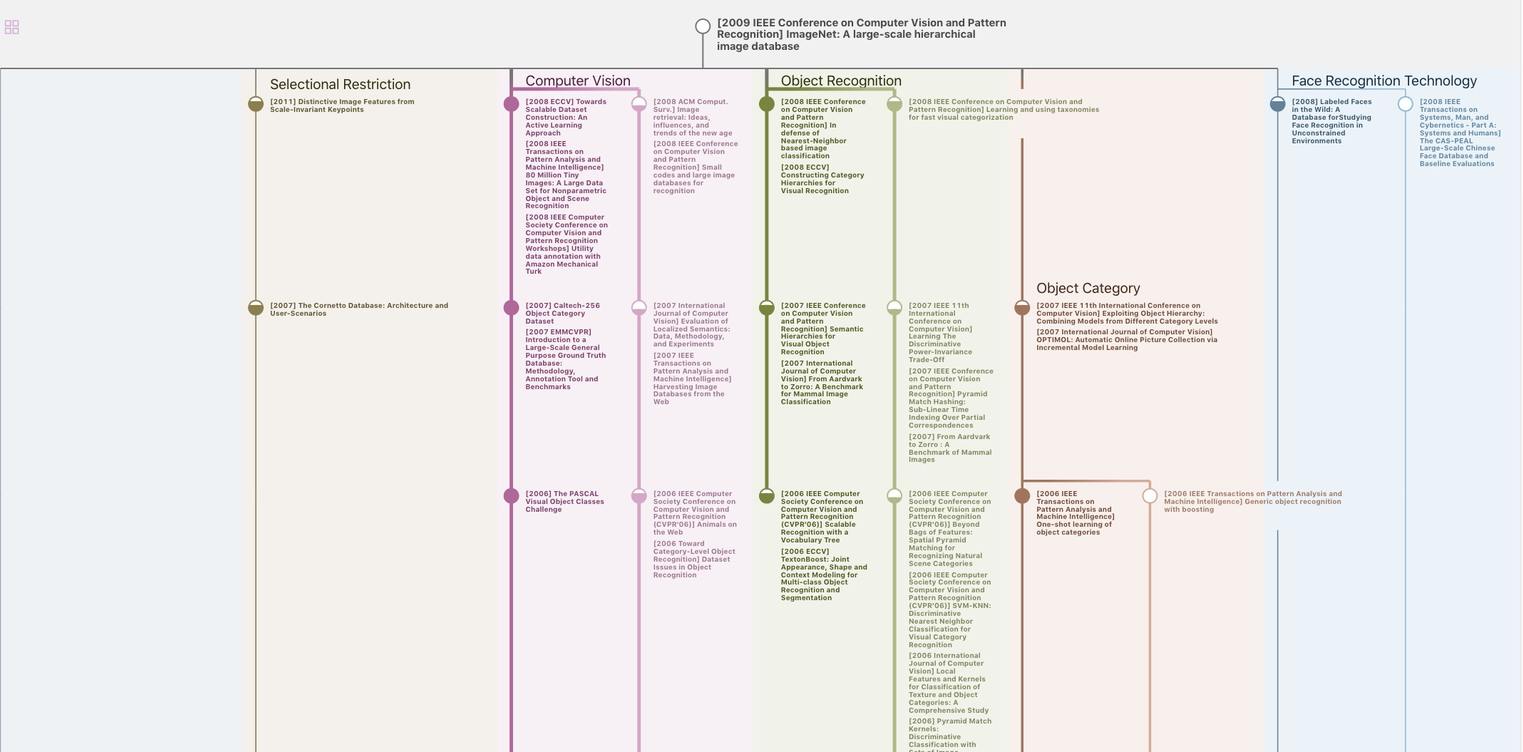
生成溯源树,研究论文发展脉络
Chat Paper
正在生成论文摘要