PLD-AL: Pseudo-label Divergence-Based Active Learning in Carotid Intima-Media Segmentation for Ultrasound Images
MEDICAL IMAGE COMPUTING AND COMPUTER ASSISTED INTERVENTION, MICCAI 2023, PT II(2023)
摘要
Segmentation of the carotid intima-media (CIM) offers more precise morphological evidence for obesity and atherosclerotic disease compared to the method that measures its thickness and roughness during routine ultrasound scans. Although advanced deep learning technology has shown promise in enabling automatic and accurate medical image segmentation, the lack of a large quantity of high-quality CIM labels may hinder the model training process. Active learning (AL) tackles this issue by iteratively annotating the subset whose labels contribute the most to the training performance at each iteration. However, this approach substantially relies on the expert's experience, particularly when addressing ambiguous CIM boundaries that may be present in real-world ultrasound images. Our proposed approach, called pseudo-label divergence-based active learning (PLD-AL), aims to train segmentation models using a gradually enlarged and refined labeled pool. The approach has an outer and an inner loops: The outer loop calculates the Kullback-Leibler (KL) divergence of predictive pseudo-labels related to two consecutive AL iterations. It determines which portion of the unlabeled pool should be annotated by an expert. The inner loop trains two networks: The student network is fully trained on the current labeled pool, while the teacher network is weighted upon itself and the student one, ultimately refining the labeled pool. We evaluated our approach using both the Carotid Ultrasound Boundary Study dataset and an in-house dataset from Children's Hospital, Zhejiang University School of Medicine. Our results demonstrate that our approach outperforms state-of-the-art AL approaches. Furthermore, the visualization results show that our approach less overestimates the CIM area than the rest methods, especially for severely ambiguous ultrasound images at the thickness direction.
更多查看译文
关键词
Carotid intima-media complex,active learning,image segmentation
AI 理解论文
溯源树
样例
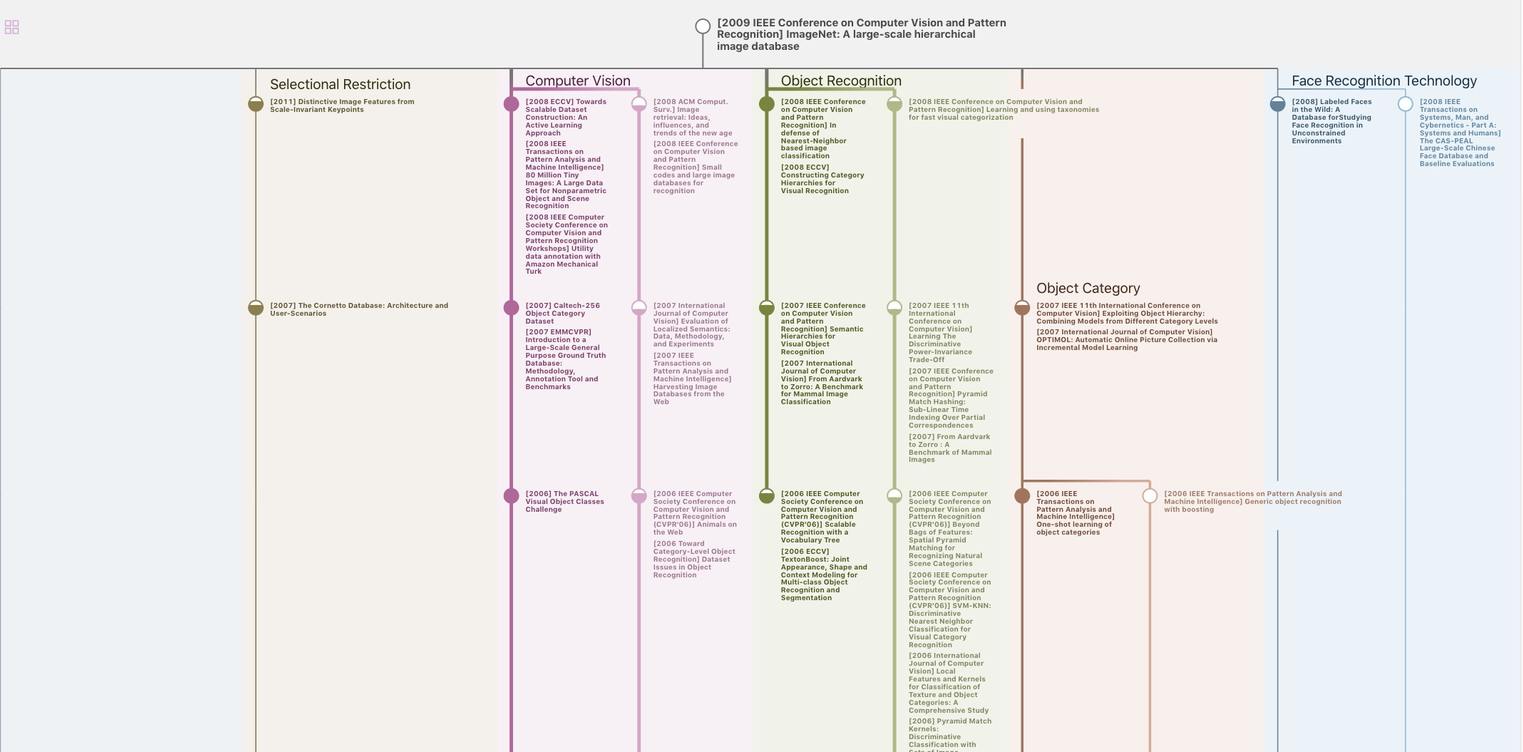
生成溯源树,研究论文发展脉络
Chat Paper
正在生成论文摘要