Boosting Knowledge Distillation via Random Fourier Features for Prostate Cancer Grading in Histopathology Images
DOMAIN ADAPTATION AND REPRESENTATION TRANSFER, DART 2023(2024)
摘要
There has been a growing number of pathology image datasets, in particular for cancer diagnosis. Although these datasets permit easy access and development of computational pathology tools, the current computational models still struggle to handle unseen datasets due to various reasons. Transfer learning and fine-tuning are standard techniques to adapt an existing model that was trained on one dataset to another. However, this approach does not fully exploit the existing model and the target dataset. Inspired by knowledge distillation, we propose a student-teacher strategy that distills knowledge from a well-trained teacher model, generally trained on a larger dataset, to a student model to be tested on a small dataset. To facilitate efficient and effective knowledge distillation and transfer, we employ contrastive learning and non-parameterized random Fourier features for compressed feature mapping into a lower-dimensional space. We evaluated our proposed method using three prostate cancer datasets, including a teacher dataset, a target student dataset, and an independent test dataset. The experimental results demonstrate that the proposed approach outperforms other transfer learning and state-of-the-art knowledge distillation methods. Code is available at: https://github.com/trinhvg/KD_CoRFF.
更多查看译文
关键词
knowledge distillation,random Fourier features,contrastive learning,cancer grading,digital pathology
AI 理解论文
溯源树
样例
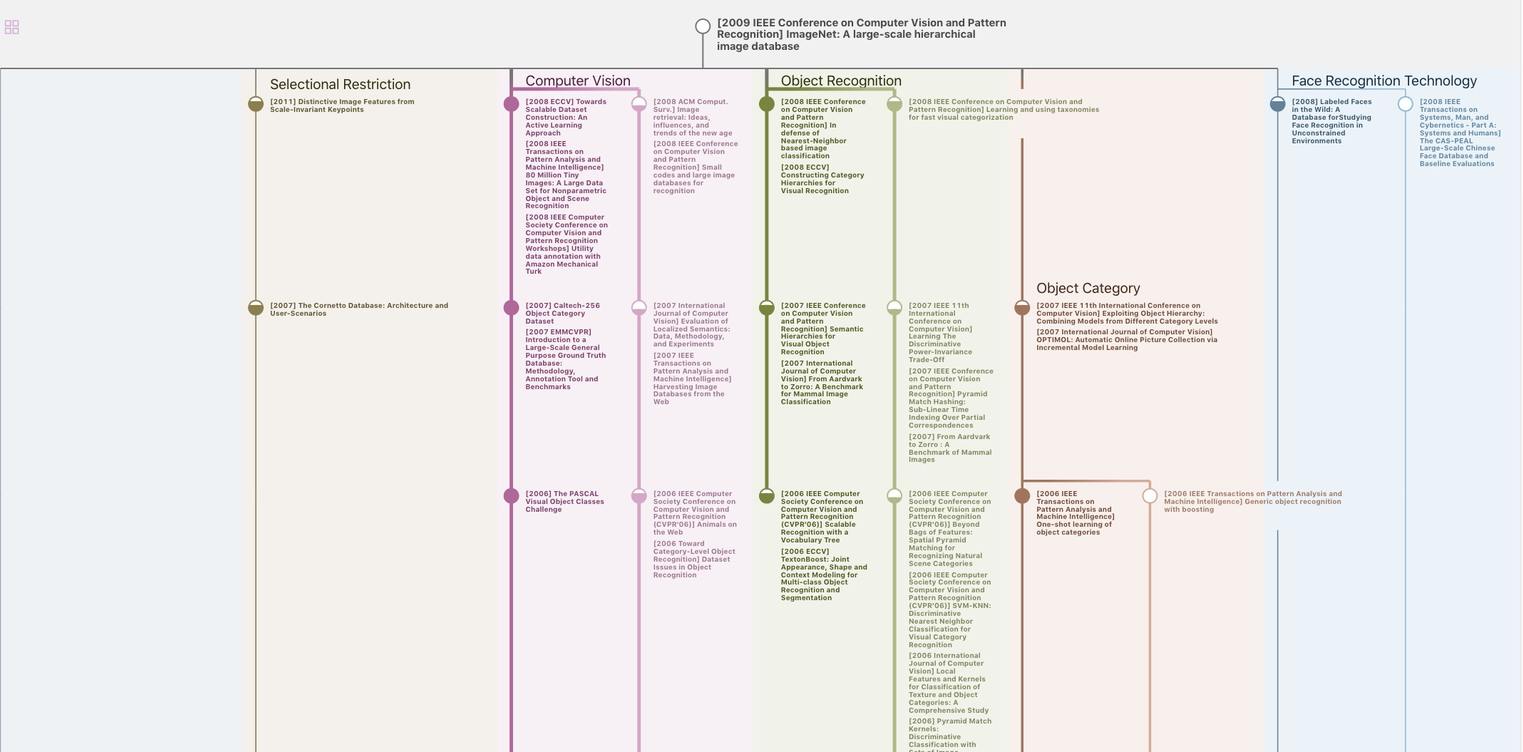
生成溯源树,研究论文发展脉络
Chat Paper
正在生成论文摘要