Neural architecture search with interpretable meta-features and fast predictors.
Inf. Sci.(2023)
摘要
Neural Architecture Search (NAS) is well-known for automatizing neural architecture design and finding better architectures. Although NAS methods have shown substantial progress over the years, most still suffer from data inefficiency, high model complexity, and lack of interpretability. This paper proposes a solution for these problems by introducing a Prediction-based and interpretable Meta-Learning method called MbML-NAS, capable of generalizing to different search spaces and datasets using less data than several state-of-the-art NAS methods. The proposal uses interpretable meta-features extracted from neural architectures and regression models as meta-predictors to infer Convolutional Networks performances. Experiments compared MbMLNAS with a graph-based Neural Predictor, state-of-the-art NAS methods, a lower bound, and an upper bound Oracle baseline. Furthermore, an interpretability analysis of meta-features and meta-predictors is presented. As a result, using at least 172 examples representing 0.04% and 1.1% of the popular NAS-Bench-101 and NAS-Bench-201 search spaces, MbML-NAS find architectures with better or comparable performances than most baselines, including the Oracle. Moreover, the results showed the potential for using simple meta-features to generalize across NAS search spaces and datasets, encoding neural architectures so that even linear models can accurately predict their performances. Additionally, novel meta-datasets suitable for NAS are proposed to facilitate research on NAS.
更多查看译文
关键词
Neural architecture search,Meta-learning,Prediction-based NAS,Interpretability,Image classification
AI 理解论文
溯源树
样例
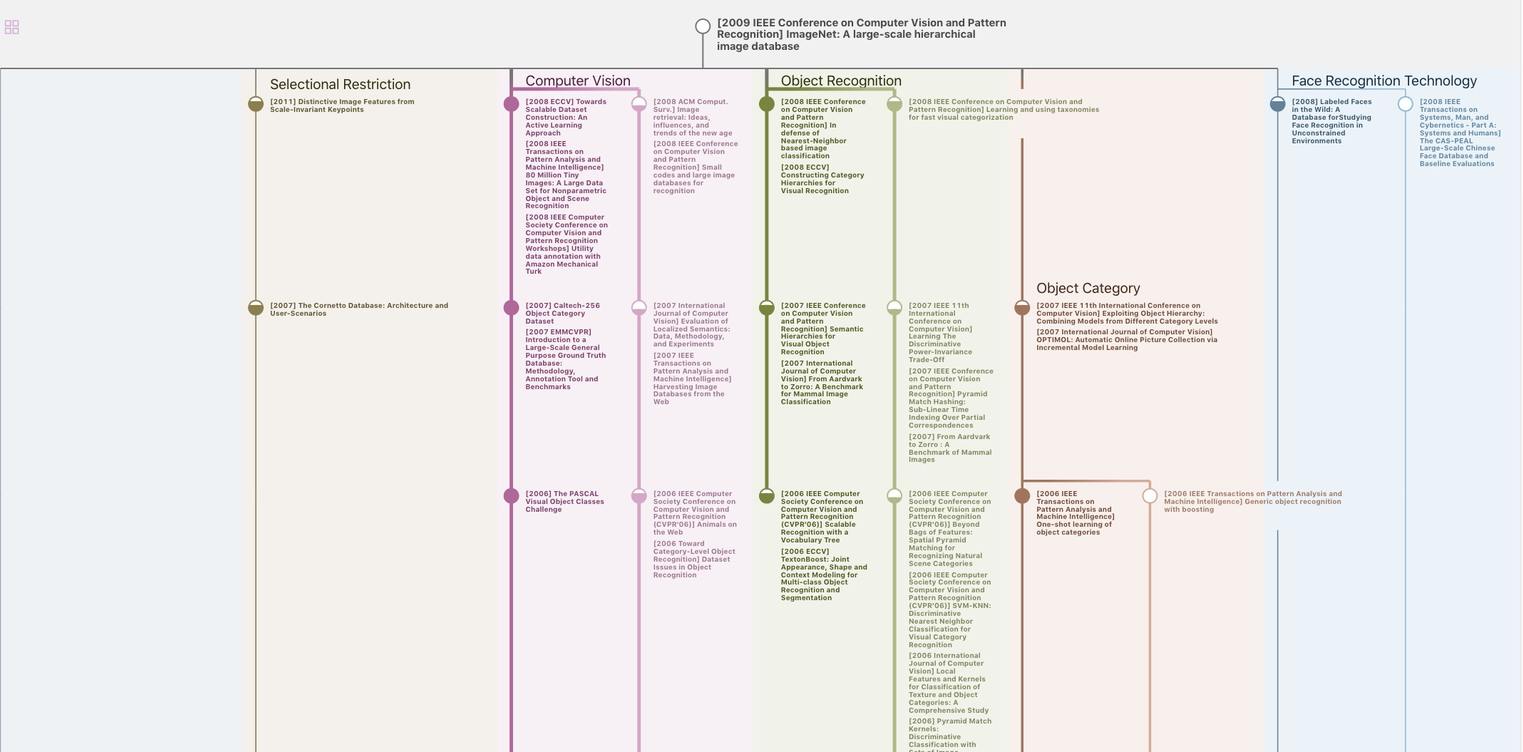
生成溯源树,研究论文发展脉络
Chat Paper
正在生成论文摘要