Confidence Intervals and Hypothesis Testing for High-dimensional Quantile Regression: Convolution Smoothing and Debiasing
JOURNAL OF MACHINE LEARNING RESEARCH(2023)
摘要
1-penalized pound quantile regression (1-QR) pound is a useful tool for modeling the relation-ship between input and output variables when detecting heterogeneous effects in the high-dimensional setting. Hypothesis tests can then be formulated based on the debiased 1-QR pound estimator that reduces the bias induced by Lasso penalty. However, the non-smoothness of the quantile loss brings great challenges to the computation, especially when the data dimension is high. Recently, the convolution-type smoothed quantile regression (SQR) model has been proposed to overcome such shortcoming, and people developed theory of estimation and variable selection therein. In this work, we combine the debiased method with SQR model and come up with the debiased 1-SQR pound estimator, based on which we then establish confidence intervals and hypothesis testing in the high-dimensional setup. Theoretically, we provide the non-asymptotic Bahadur representation for our proposed es-timator and also the Berry-Esseen bound, which implies the empirical coverage rates for the studentized confidence intervals. Furthermore, we build up the theory of hypothesis testing on both a single variable and a group of variables. Finally, we exhibit extensive numerical experiments on both simulated and real data to demonstrate the good performance of our method.
更多查看译文
关键词
High-dimensional quantile regression,convolution-based smoothing,debiased method,hypothesis testing,non-asymptotic statistics
AI 理解论文
溯源树
样例
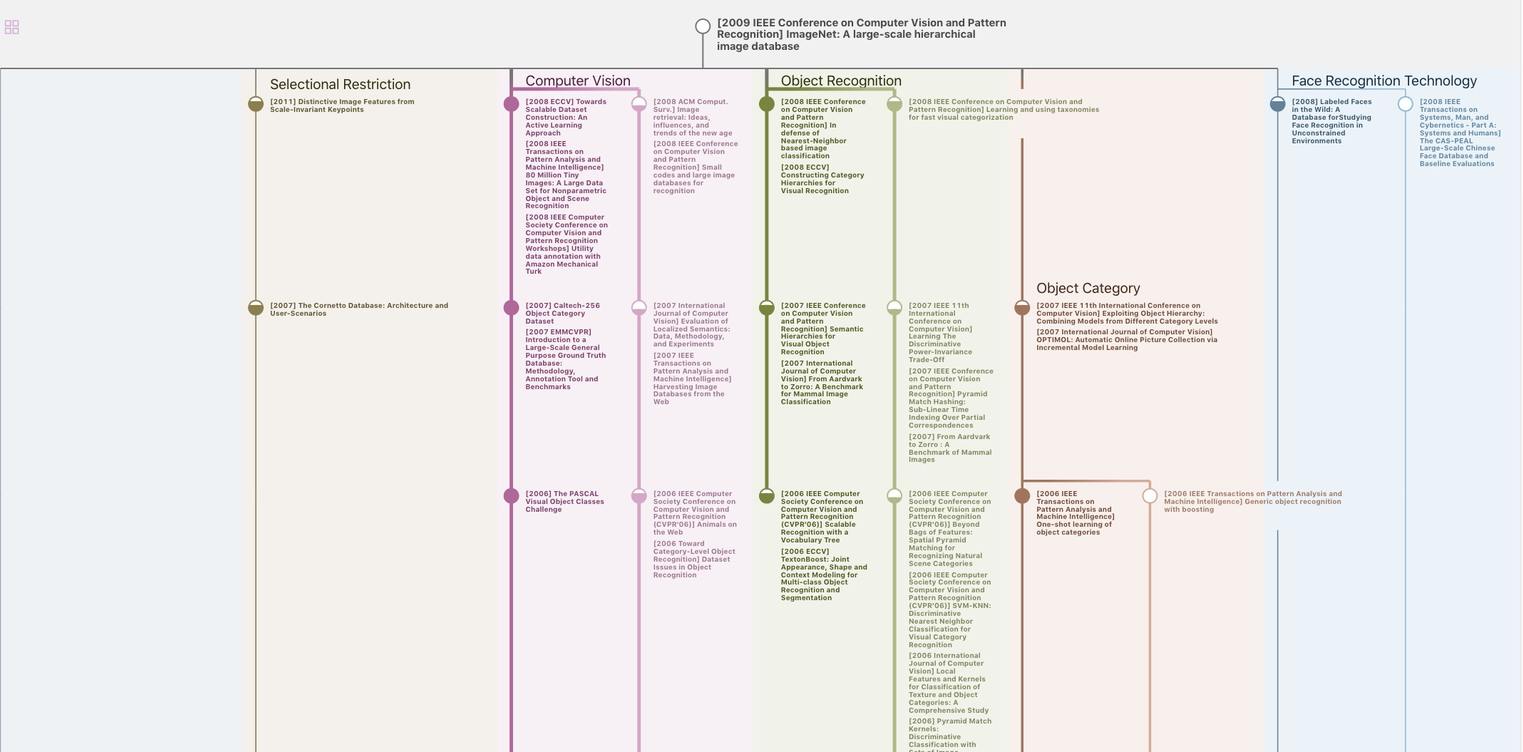
生成溯源树,研究论文发展脉络
Chat Paper
正在生成论文摘要