Comprehensive Dynamic Structure Graph Neural Network for Aero-Engine Remaining Useful Life Prediction.
IEEE Trans. Instrum. Meas.(2023)
摘要
Accurate remaining useful life (RUL) prediction is of great significance to the safe operation of aircraft. Graph neural network (GNN) can describe the spatial structure relationship between variables, which provides a new solution to the problem of RUL prediction. In this article, we propose a new RUL prediction method based on GNN, which is named comprehensive dynamic structure GNN (CDSG). The proposed CDSG not only fully exploits the health information hidden in the condition monitoring data but also takes into account the structural characteristics of the aero-engine. This method uses the designed dynamic graph learning (DGL) module to capture the potential dynamic relationships between time series data and combines them with the structural characteristics of the aero-engine to generate the comprehensive graph structure. In addition, the global temporal features obtained by the visual graph (VG) algorithm and graph convolutional network (GCN) are introduced into the proposed model, which can improve the predictive performance of the method. The validity of CDSG is verified with the commercial modular aero-propulsion system simulation (C-MAPSS) and N-CMAPSS datasets. The experimental results show that the proposed CDSG has a better performance compared with the existing state-of-the-art methods.
更多查看译文
关键词
Feature extraction, Graph neural networks, Aircraft propulsion, Time series analysis, Data mining, Predictive models, Data models, Graph neural network (GNN), graph structure learning, remaining useful life (RUL) prediction, visibility graph
AI 理解论文
溯源树
样例
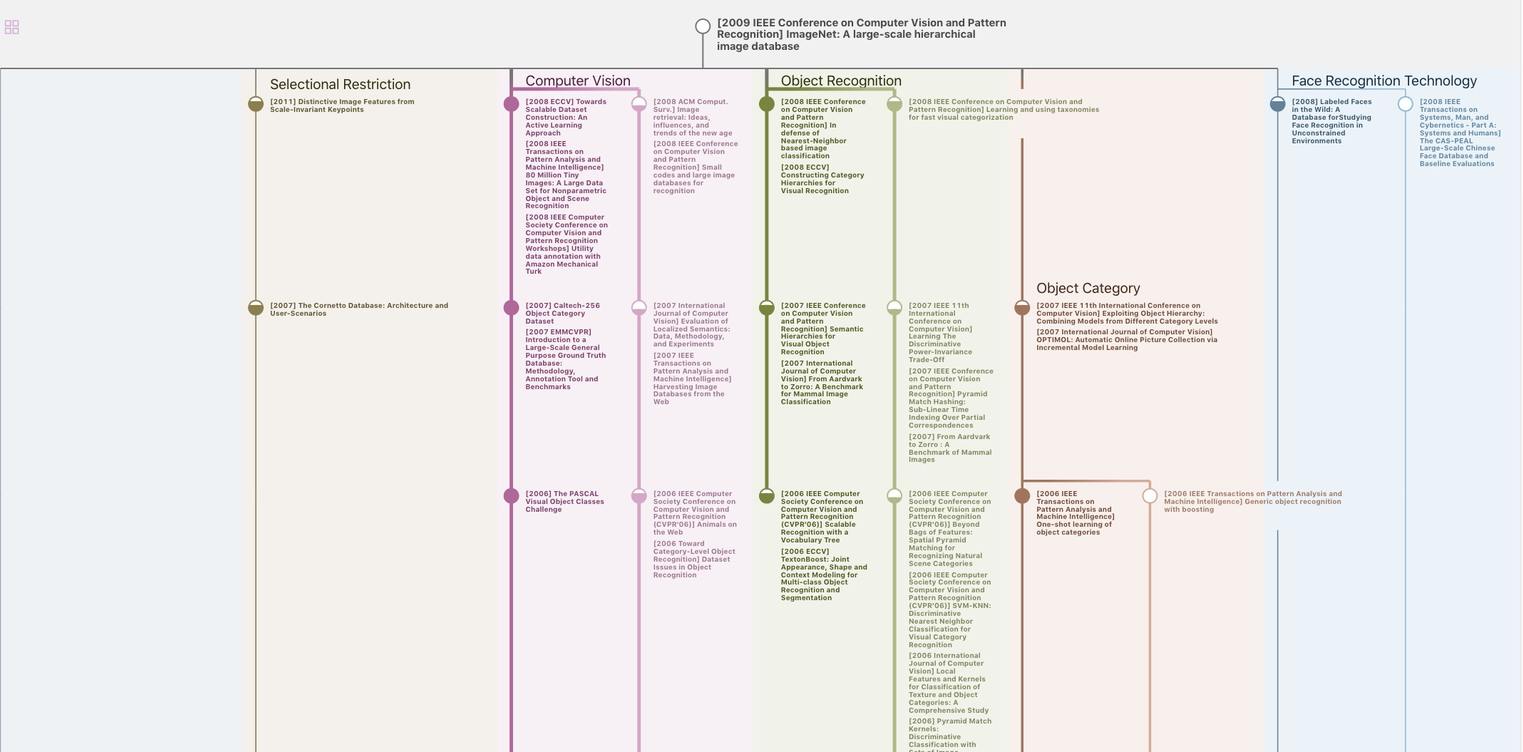
生成溯源树,研究论文发展脉络
Chat Paper
正在生成论文摘要