Segmentation-Guided Semantic-Aware Self-Supervised Denoising for SAR Image.
IEEE Trans. Geosci. Remote. Sens.(2023)
摘要
Synthetic aperture radar (SAR) images often suffer from speckle noise, which can degrade their visual quality and affect downstream applications. Recently, supervised and self-supervised methods have been proposed by virtue of deep learning with synthetic “noisy–clean” image pairs or only real noisy images as training data, respectively. Among these methods, by avoiding artifact problems in real SAR image denoising, self-supervised methods solve the domain gap problem of supervised methods and hence have attracted significant attention. However, existing self-supervised denoising methods essentially rely on pixel information of images and ignore the corresponding semantic information, which makes them challenging to remove speckle noise while retaining the detailed features. To this end, we propose a segmentation-guided semantic-aware self-supervised denoising method for SAR images, namely, SARDeSeg, where a segmentation network is incorporated with a denoising network and guides it to learn and be aware of the semantic information of the input noisy SAR images. In addition, a wavelet transform-based connector is introduced to efficiently transmit semantic information between the denoising network and the segmentation network, together with an edge-aware smoothing loss to improve speckle noise suppression while preserving edge features. The experimental results demonstrate that the proposed SARDeSeg outperforms state-of-the-art (SOTA) denoising methods for SAR images, particularly in preserving detailed edge features.
更多查看译文
关键词
Edge feature preservation, image denoising, self-supervised learning, speckle noise, synthetic aperture radar (SAR)
AI 理解论文
溯源树
样例
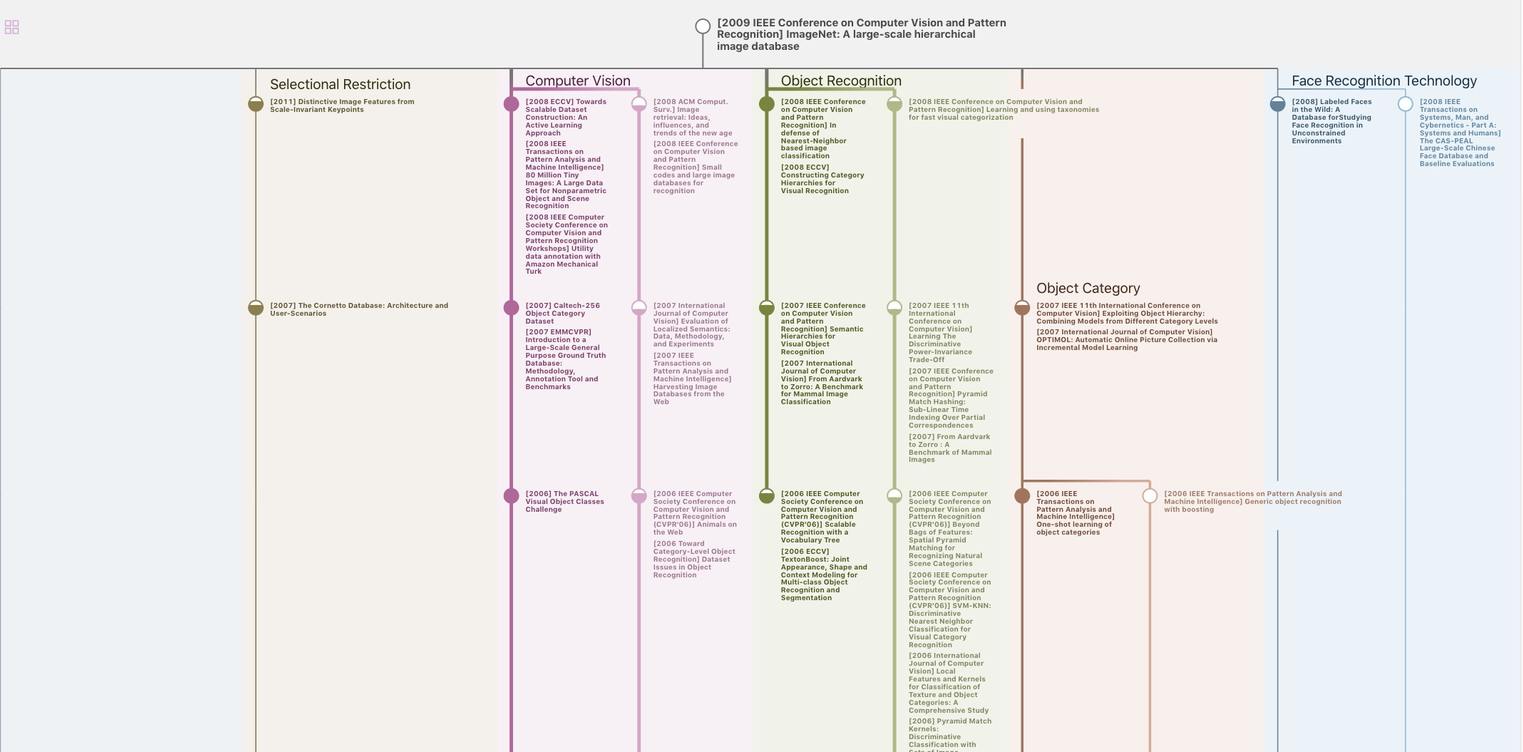
生成溯源树,研究论文发展脉络
Chat Paper
正在生成论文摘要