Multi-view clustering with Laplacian rank constraint based on symmetric and nonnegative low-rank representation.
Comput. Vis. Image Underst.(2023)
摘要
Multi-view spectral clustering has attracted more and more attention due to its promising advantages in data clustering, and many related methods have been proposed, among which the method based on low -rank representation have become increasingly popular. However, the similarity matrices learned by the existing methods inevitably contain some negative entries and are generally nonsymmetric. In order to guarantee weight consistency for each pair of data points and highly correlated data points to be together, we propose a new multi-view spectral clustering model based on the symmetric and nonnegative low -rank representation. This model can learn the view-specific consistent similarity matrix and also capture the local structure and discriminative information among data by imposing symmetric and nonnegative constraints on the representation matrix. By employing the compatible and complementary information from multi-view data points, it can also learn the consensus similarity matrix for all views and perform the spectral clustering simultaneously. By the augmented Lagrangian multiplier method, an alternating iterative optimization algorithm is designed for solving this model and its computational complexity and convergence is analyzed. Comprehensive experiments on multiple multi-view data sets also verify the effectiveness and superiority of the proposed approach.
更多查看译文
关键词
Multi-view clustering,Low-rank representation,Symmetry,Nonnegativeness,Similarity matrix
AI 理解论文
溯源树
样例
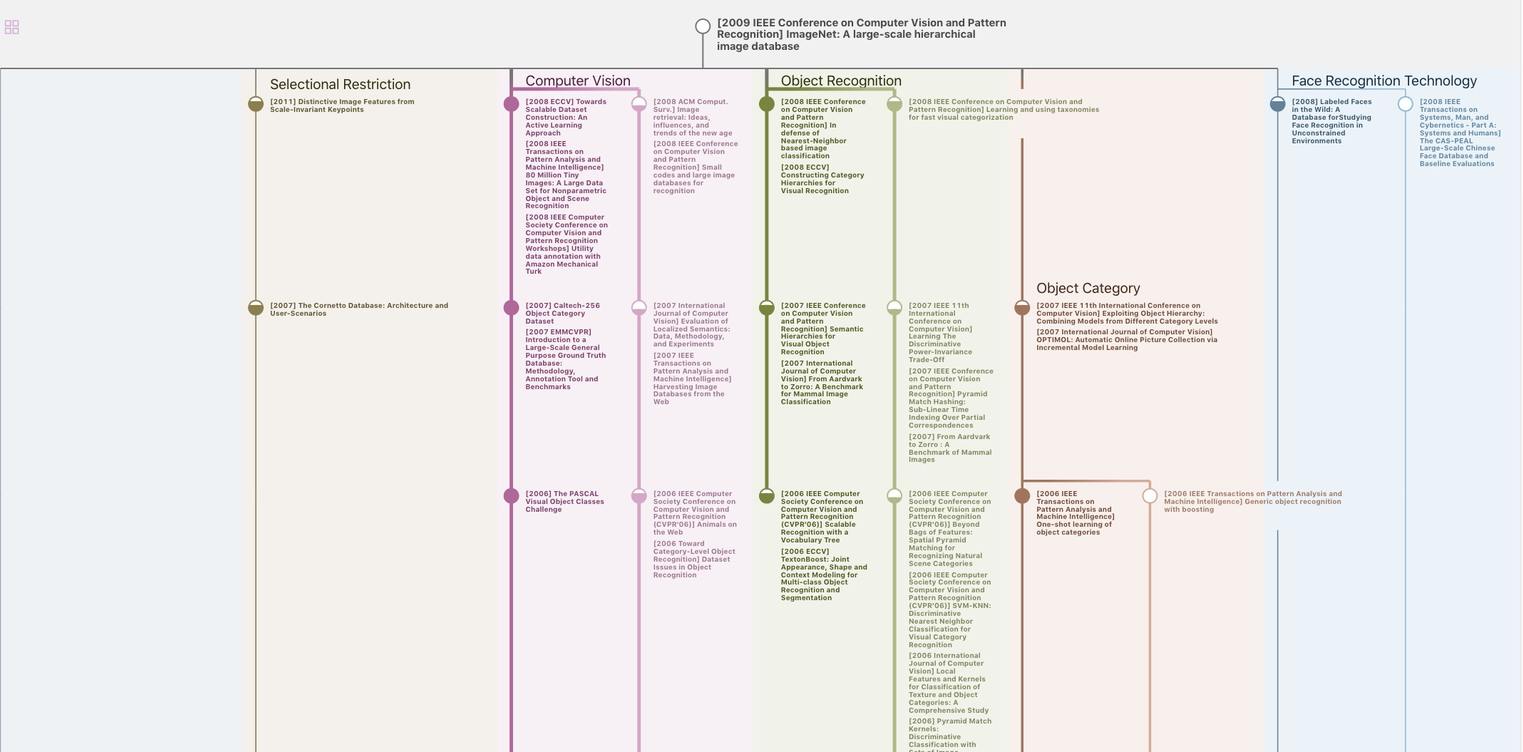
生成溯源树,研究论文发展脉络
Chat Paper
正在生成论文摘要