Event-triggered learning synchronization of coupled heterogeneous recurrent neural networks
KNOWLEDGE-BASED SYSTEMS(2023)
摘要
This paper investigates the synchronization of coupled heterogeneous recurrent neural networks. Based on the assumption of the existence of a spanning tree in the communication digraph, an effective event-triggered iterative learning control applicable to continuous nonlinear dynamical systems is proposed, under which some sufficient criteria for guaranteeing the synchronization of coupled heterogeneous recurrent neural networks are rigorously derived in virtue of contracting mapping principle. Moreover, the exclusion of the Zeno behaviors is analyzed. In contrast with relevant existing results, the control presented herein is applicable to both continuous and nonlinear dynamical systems, and the designed control involves the directed topology with a spanning tree, which includes the existing controls that based on the strongly connected topologies as special cases. Finally, the validity of theoretical results is substantiated by a numerical example. (c) 2023 Elsevier B.V. All rights reserved.
更多查看译文
关键词
Synchronization,Heterogeneous,Recurrent neural networks,Event-triggered control,Iterative learning control
AI 理解论文
溯源树
样例
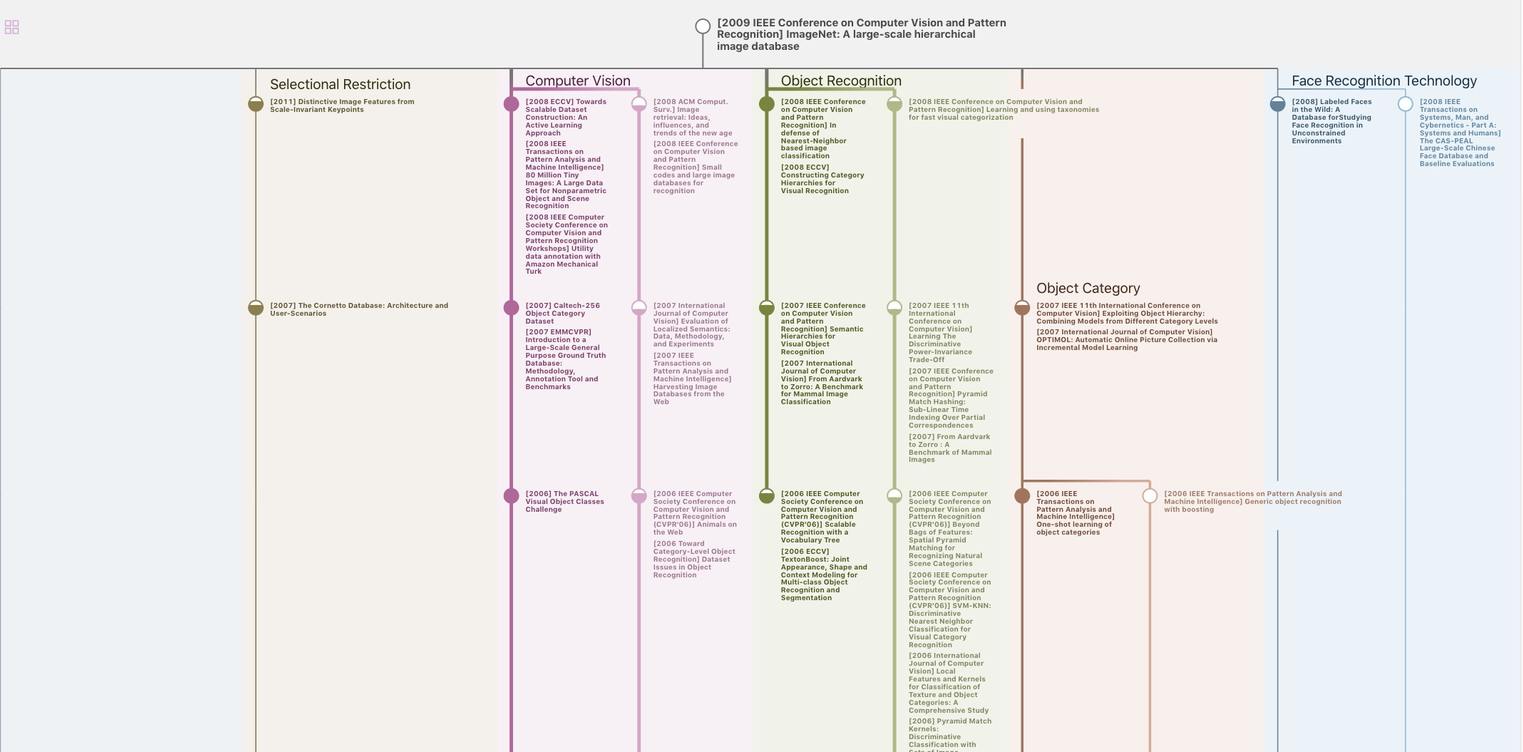
生成溯源树,研究论文发展脉络
Chat Paper
正在生成论文摘要