Boosting Short Text Classification by Solving the OOV Problem.
IEEE ACM Trans. Audio Speech Lang. Process.(2023)
摘要
In the field of natural language processing, text classification has received a lot of attention. Compared with long texts, short texts have fewer words and lack contextual semantic information. Existing approaches enrich short text information by linking the external knowledge graph, but they ignore the out-of-vocabulary (OOV) problem during entity linking, especially when dealing with domain-oriented data, which has some rare words or domain-specific nouns. In this article, to alleviate the OOV problem caused by linking the external knowledge graph(KG), we propose a domain knowledge graph and entity complementation strategy to improve the performance of short text classification. Specifically, the external knowledge graph is used to enrich the information of short texts. The self-build domain knowledge graph is used to solve the problem of entities failing to link to the external knowledge graph. Finally, we conduct experiments on various datasets: 1. a labeled Chinese electronic domain dataset; 2. an open-source dataset to test the performance of our algorithm in different data distribution scenarios. The results demonstrate our dual knowledge graph model outperforms the state-of-the-art short text classification methods, especially when the OOV problem is severe.
更多查看译文
关键词
Dual knowledge graph,knowledge enhancement,out of vocabulary problem,short text classification
AI 理解论文
溯源树
样例
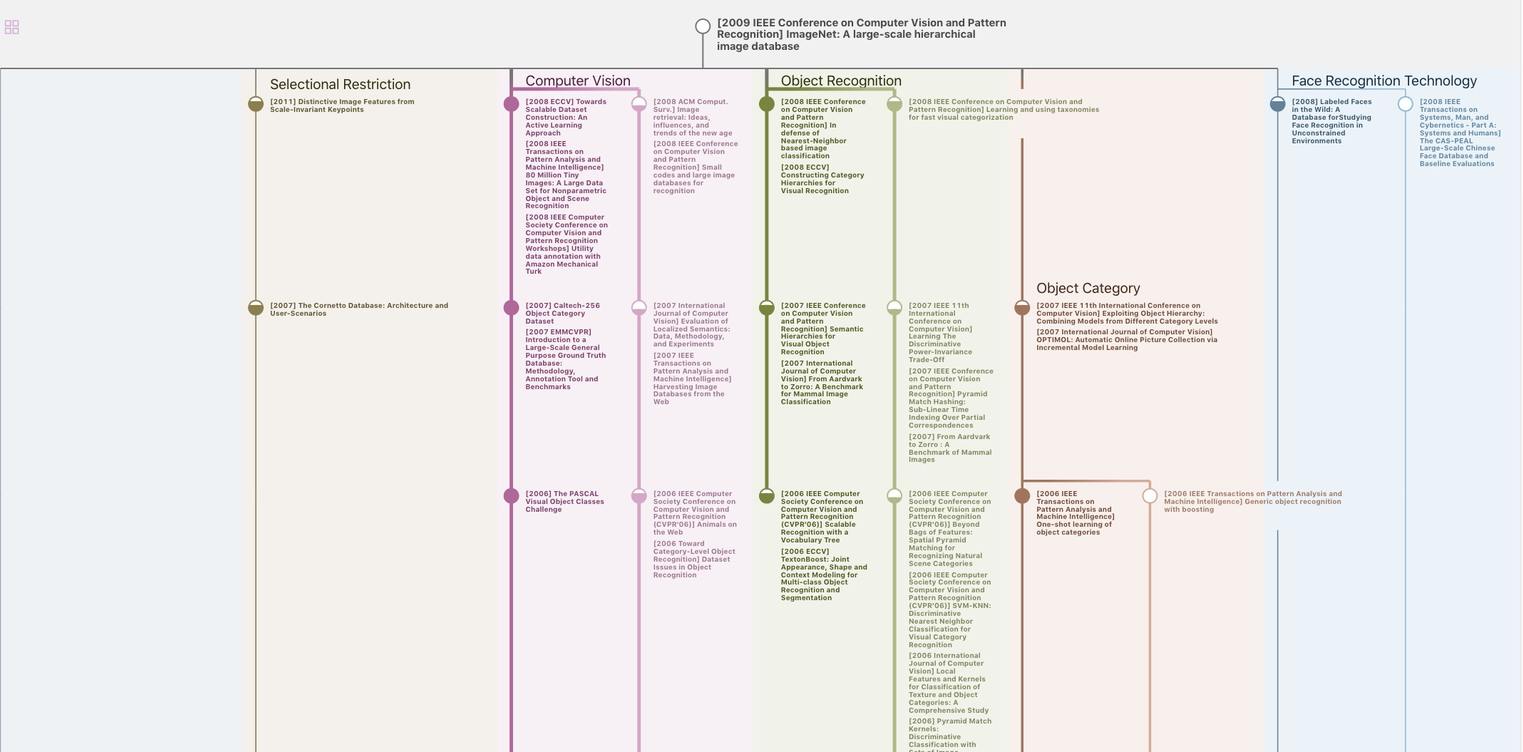
生成溯源树,研究论文发展脉络
Chat Paper
正在生成论文摘要