Text Steganalysis Based on Hierarchical Supervised Learning and Dual Attention Mechanism.
IEEE ACM Trans. Audio Speech Lang. Process.(2023)
摘要
Recent methods with deep neural networks for text steganalysis have succeeded in mining various feature representations. However, a limited number of studies have explicitly analyzed potential security issues of generative text steganography. Furthermore, current text steganalysis approaches lack detailed consideration in the intricate design of deep learning architectures tailored to these challenges. In this article, in order to tackle these problems, we first theoretically and empirically analyze the inevitable embedding distortions of generative text steganography at a semantic and statistical levels. In light of this, we then propose an innovative text steganalysis method based on hierarchical supervised learning and a dual attention mechanism. Concretely, to extract highly effective semantic features, the proposed method involves fine-tuning a BERT extractor through the hierarchical supervised learning that combines signals from multiple softmax classifiers, rather than relying solely on the final one. The mean and standard deviation values in the Gaussian distribution of cover and stego texts are then estimated using an encoder of variational autoencoders and used to capture features representing the statistical distortion of generative text steganography. Subsequently, we introduce a dual attention mechanism that dynamically fuses the semantic and statistical features, thereby creating discriminative feature representations essential for text steganalysis. The experimental results demonstrate that our proposed text steganalysis method surpasses the current state-of-the-art techniques across three distinct text steganalysis scenarios: specific text steganalysis, semi-blind text steganalysis, and blind text steganalysis.
更多查看译文
关键词
Text steganalysis,hierarchical supervised learning,dual attention mechanism
AI 理解论文
溯源树
样例
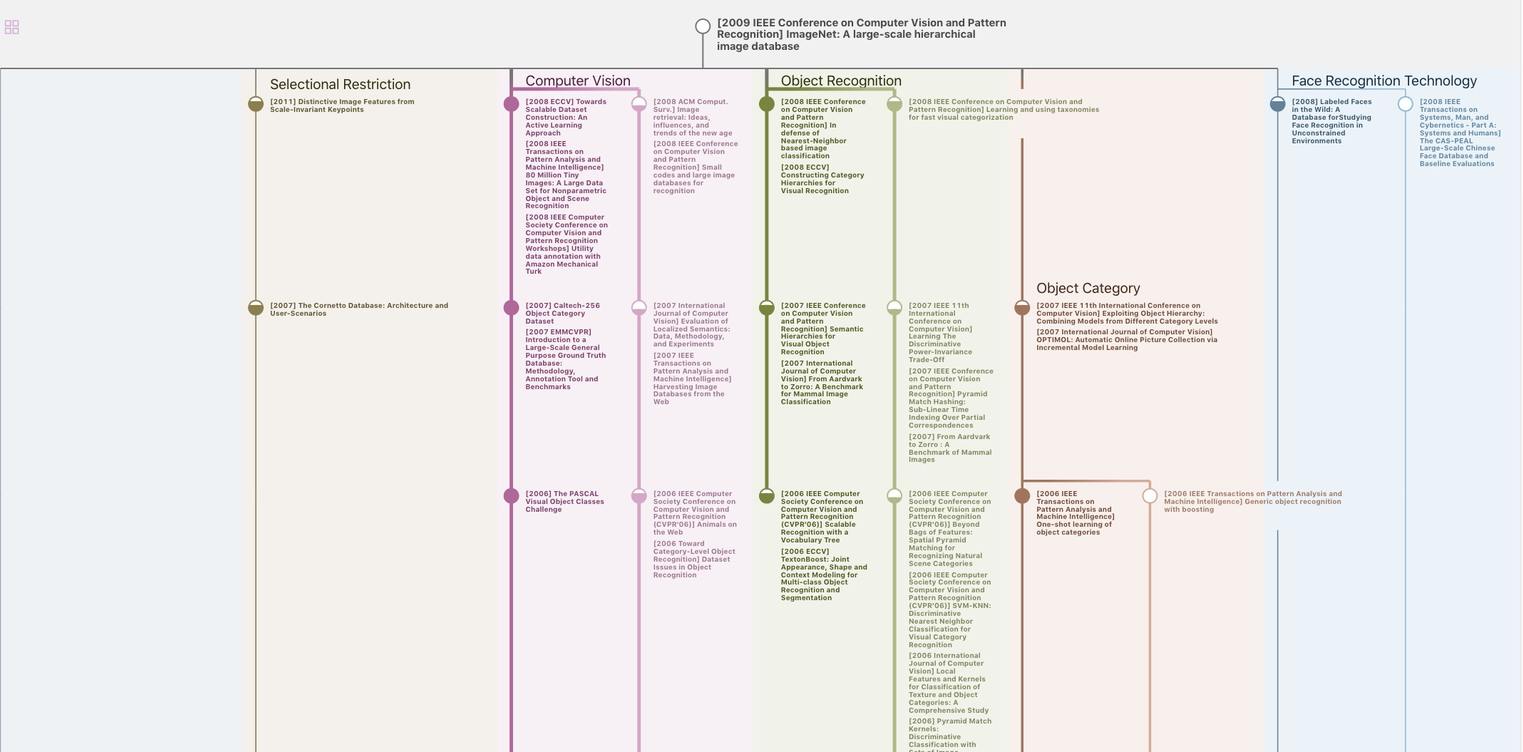
生成溯源树,研究论文发展脉络
Chat Paper
正在生成论文摘要