Designing Concept Drift Detection Ensembles: A Survey
2023 IEEE 10th International Conference on Data Science and Advanced Analytics (DSAA)(2023)
摘要
Data streams represent real-world concepts that typically cannot be assumed to be static. Instead, sensors monitoring industrial resources deteriorate, network intrusion attacks exhibit new patterns, consumer behavior or public interest in news topics change. These are examples of a phenomenon commonly known as concept drift. It threatens the performance of estimation models conducting inference on associated data. Explicitly employed concept drift detection is among the most promising approaches to maintain a stable and robust performance of productively used estimators, as it identifies the times when adaptation to new concepts becomes actually necessary. Combining concept drift detectors to form ensembles can additionally increase detection performance as it aggregates their individual specializations and allows advanced processing of concept drift evidence. This survey is the first to systematically retrieve and overview research relevant in the field of concept drift detection ensembles. It identifies the research as being in its initial stages and reveals numerous gaps, which should be addressed to further exploit the substantial performance potential ensembles have over individually applied detectors. Furthermore, it contributes to the literature by discussing and describing key characteristics and principles of their design in a structured manner.
更多查看译文
关键词
concept drift detection ensembles,ensemble methods,data stream mining
AI 理解论文
溯源树
样例
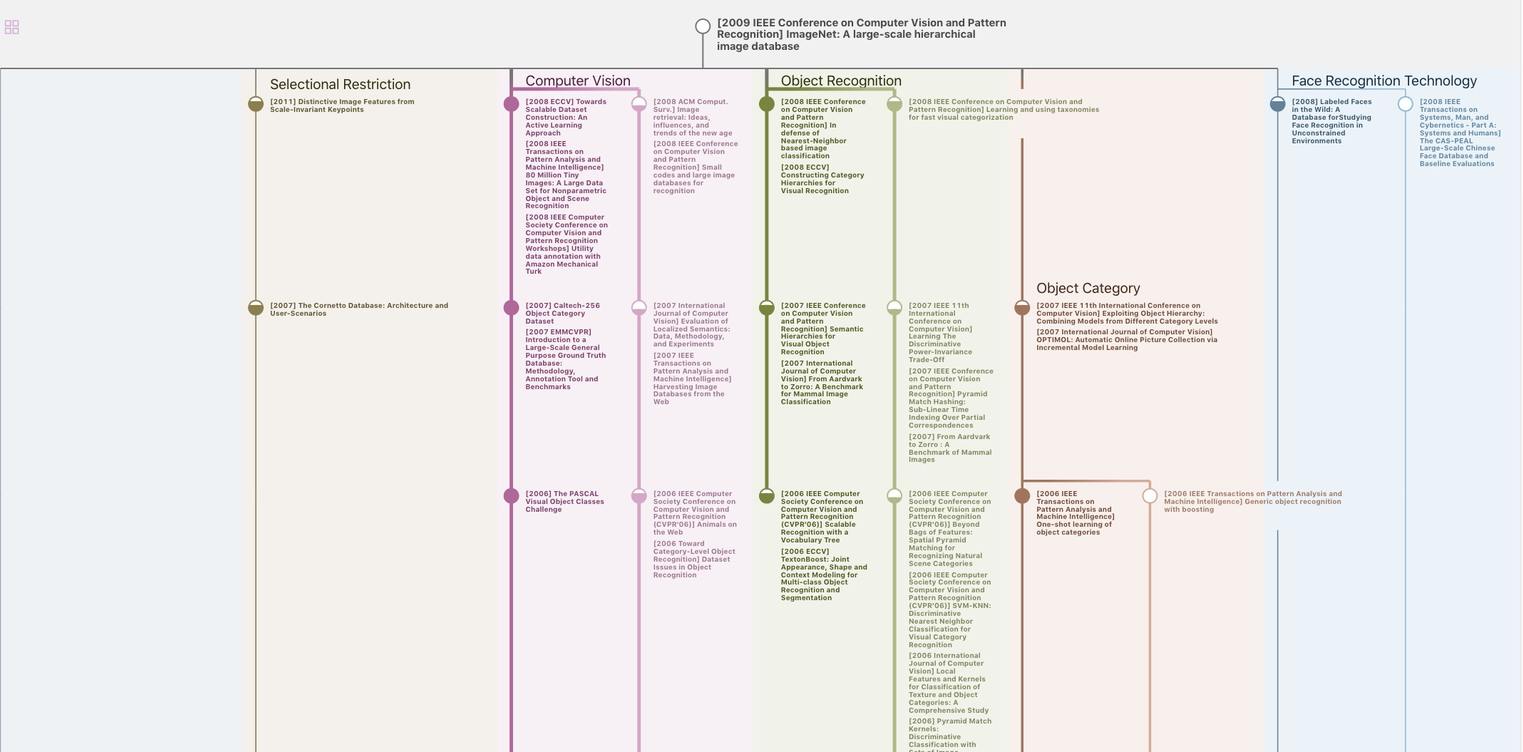
生成溯源树,研究论文发展脉络
Chat Paper
正在生成论文摘要