V2V-Assisted Timely Hierarchical Federated Learning
2023 IEEE 24th International Workshop on Signal Processing Advances in Wireless Communications (SPAWC)(2023)
摘要
To meet the timeliness and data privacy requirements of vehicular applications, federated learning (FL) has been applied in edge training for connected vehicles. However, FL in vehicular networks needs to meet stringent latency requirements due to the high mobility of vehicles. This paper proposes a vehicle-to-vehicle (V2V)-assisted hierarchical federated learning (VAHFL) architecture to reduce the communication latency for model uploading. We formulate the communication latency minimization problem as a sequential decision-making problem. This problem is too complex and we divide it into three sub-problems: V2V link scheduling, bandwidth allocation, and sequential node classification. The first two problems can be solved using optimization methods, while the third problem is still hard to solve due to unavailable noncausal information. We develop a graph neural network (GNN)-reinforcement learning (RL) based framework to solve the third problem in an online manner. Experimental results show that our proposed scheme can reduce the latency by at least 41.99% compared with the benchmarks. Additionally, the convergence speed and the testing accuracy of our proposed scheme exceed that of the benchmarks under both i.i.d. and non-i.i.d. settings.
更多查看译文
AI 理解论文
溯源树
样例
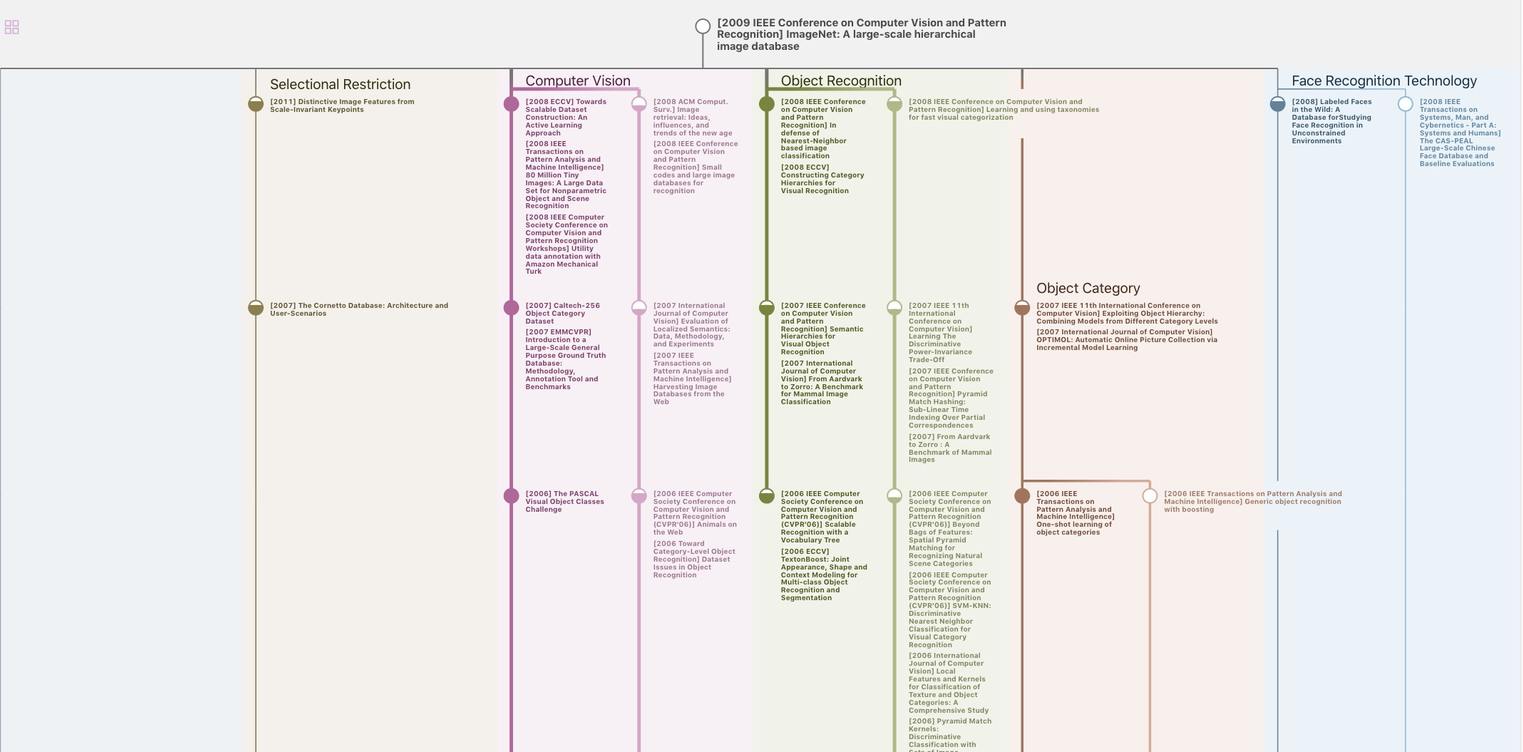
生成溯源树,研究论文发展脉络
Chat Paper
正在生成论文摘要