Toward All-Digital Time-Domain Neural Network Accelerators for In-Sensor Processing Applications
2023 IEEE Nordic Circuits and Systems Conference (NorCAS)(2023)
摘要
Deep Neural Network (DNN) accelerators are increasingly integrated into sensing applications, such as wearables and sensor networks, to provide advanced in-sensor processing capabilities. Given wearables' strict size and power requirements, minimizing the area and energy consumption of DNN accelerators is a critical concern. In that regard, computing DNN models in the time domain is a promising architecture, taking advantage of both technology scaling friendliness and efficiency. Yet, time-domain accelerators are typically not fully digital, limiting the full benefits of time-domain computation. In this work, we propose a time-domain multiply and accumulate (MAC) circuitry enabling an all-digital with a small size and low energy consumption to target in-sensor processing. The proposed MAC circuitry features a simple and efficient architecture without dependencies on analog non-idealities such as leakage and charge errors. It is implemented in 22nm FD-SOI technology, occupying 35 mu m x 35 mu m while supporting multi-bit inputs (8-bit) and weights (4-bit). The power dissipation is 46.61 mu W at 500MHz, and 20.58 mu W at 200MHz. Combining 32 MAC units achieves an average power efficiency, area efficiency and normalized efficiency of 0.45 TOPS/W and 75GOPS/mm(2), and 14.4 1b-TOPS/W.
更多查看译文
关键词
Edge Computing,Human Activity Recognition HAR,Inertial Measurement Unit IMU,In-Sensor Processing,Multiply-and-Accumulate MAC,Neural Network Accelerator,Smart Sensor Interface,Time-Domain Signal Processing
AI 理解论文
溯源树
样例
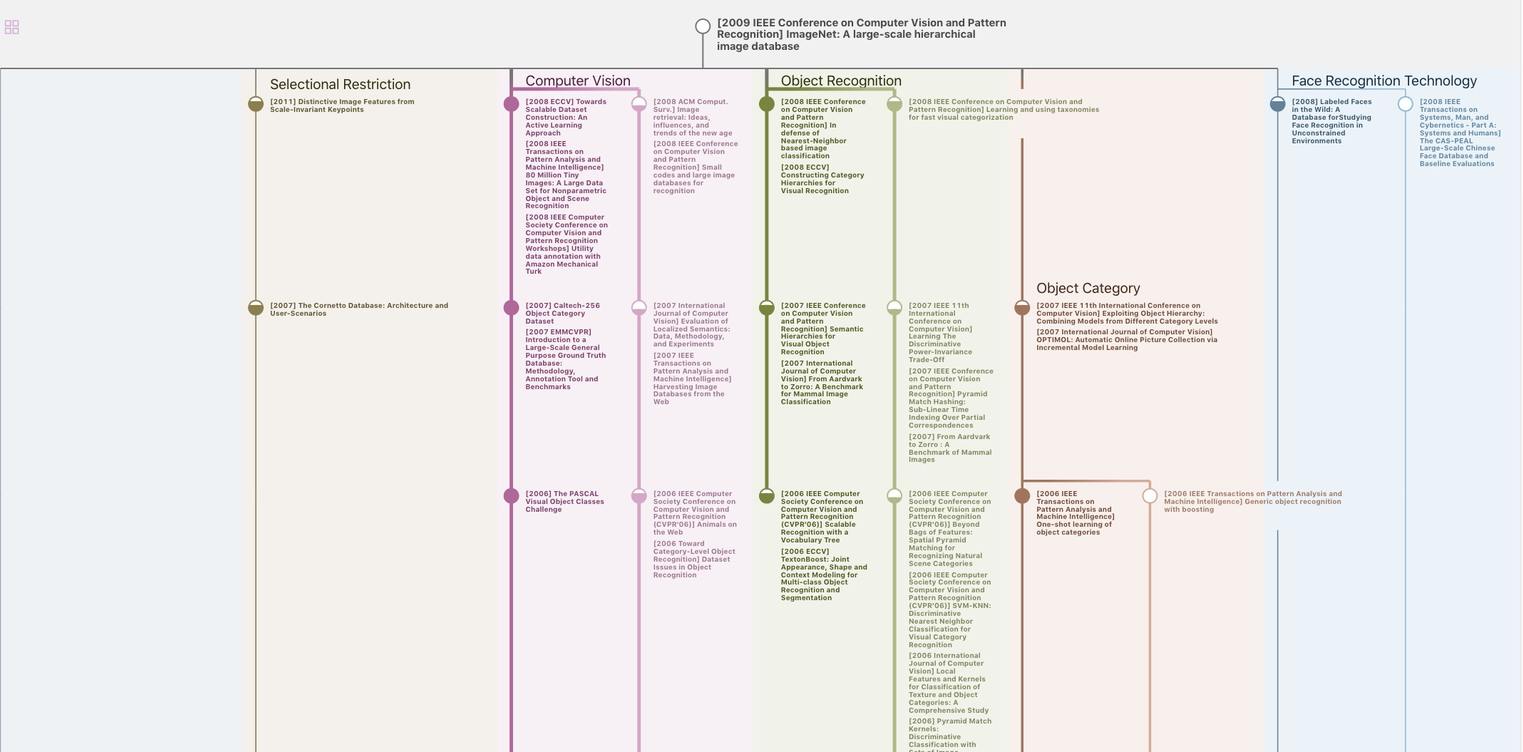
生成溯源树,研究论文发展脉络
Chat Paper
正在生成论文摘要