Deep Learning-Enhanced Parameter Extraction for Equivalent Circuit Modeling in Electrochemical Impedance Spectroscopy
2023 IEEE NORDIC CIRCUITS AND SYSTEMS CONFERENCE, NORCAS(2023)
摘要
Reliable and automatic parameter extraction in equivalent circuit modeling of electrochemical impedance spectroscopy (EIS) could be a challenge as the common circuit fitting method, complex nonlinear least-squares (CNLS), heavily depends on the initial guesses. To prevent the adjustment of the initial guess that demands extra time and experience, we propose employing a deep learning-based convolutional neural network (CNN) to perform the pre-fitting of the measured impedance spectrum. This approach not only facilitates the convergence dynamics of CNLS but also manifests a notable enhancement in parameter extraction fidelity, especially when benchmarked against conventional methodologies. The improvement of 25% in fitting success rate is demonstrated on an open-source impedance dataset by comparing to CNLS with random initials and the traditional stochastic methods including differential evolution and simulated annealing. Thus, we believe the proposed prefitting method can provide a useful tool for reliable parameter extraction with the uncertainty minimized to explore the underlying mechanism from EIS and automate this process for the analysis of a large amount of data.
更多查看译文
关键词
Equivalent Circuit,Electrochemical Impedance Spectroscopy,Deep Learning,Convolutional Neural Network.
AI 理解论文
溯源树
样例
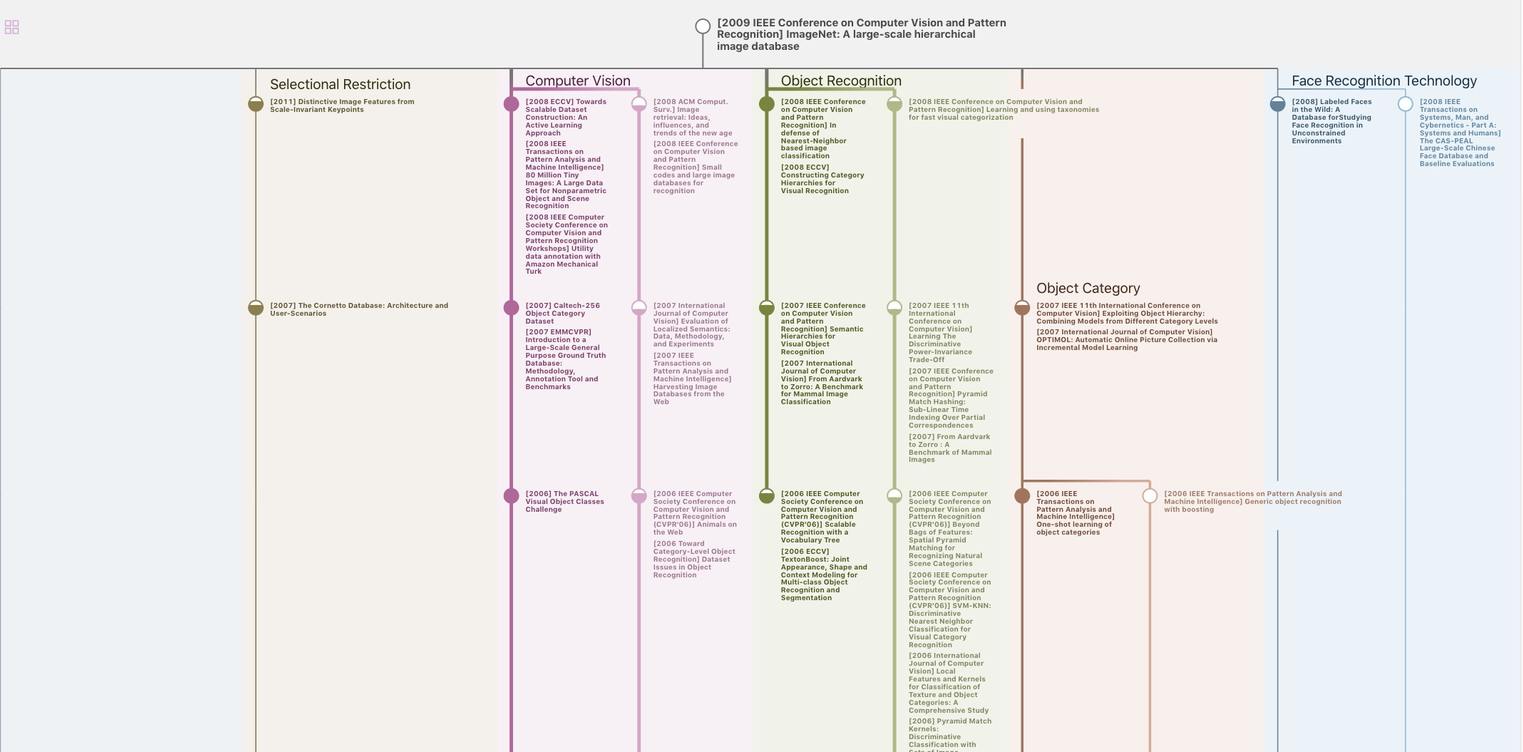
生成溯源树,研究论文发展脉络
Chat Paper
正在生成论文摘要