COLA: COarse-LAbel multi-source LiDAR semantic segmentation for autonomous driving.
CoRR(2023)
摘要
LiDAR semantic segmentation for autonomous driving has been a growing field of interest in the past few years. Datasets and methods have appeared and expanded very quickly, but methods have not been updated to exploit this new availability of data and continue to rely on the same classical datasets. Different ways of performing LIDAR semantic segmentation training and inference can be divided into several subfields, which include the following: domain generalization, the ability to segment data coming from unseen domains ; source-to-source segmentation, the ability to segment data coming from the training domain; and pre-training, the ability to create re-usable geometric primitives. In this work, we aim to improve results in all of these subfields with the novel approach of multi-source training. Multi-source training relies on the availability of various datasets at training time and uses them together rather than relying on only one dataset. To overcome the common obstacles found for multi-source training, we introduce the coarse labels and call the newly created multi-source dataset COLA. We propose three applications of this new dataset that display systematic improvement over single-source strategies: COLA-DG for domain generalization (up to +10%), COLA-S2S for source-to-source segmentation (up to +5.3%), and COLA-PT for pre-training (up to +12%).
更多查看译文
关键词
semantic segmentation,lidar,autonomous driving,coarse-label,multi-source
AI 理解论文
溯源树
样例
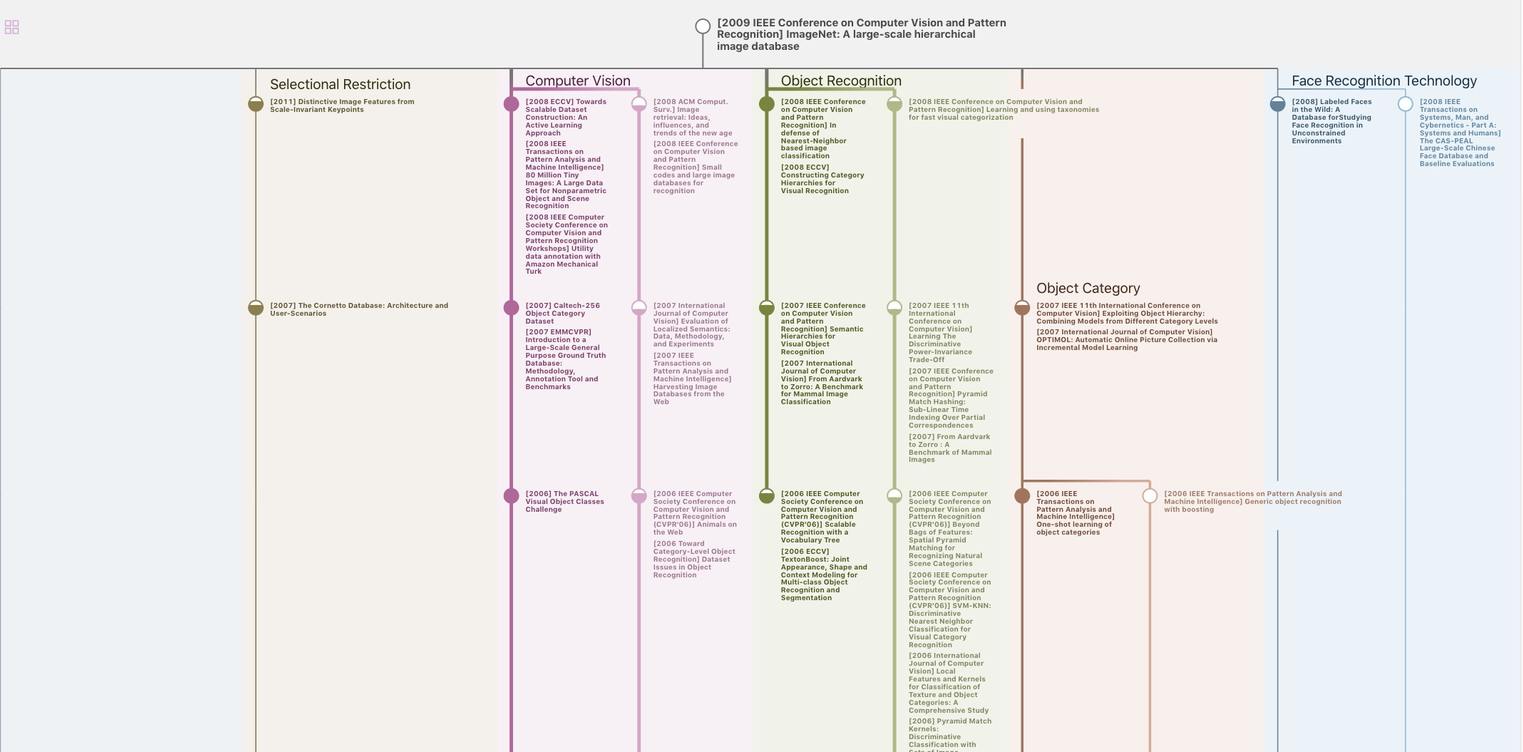
生成溯源树,研究论文发展脉络
Chat Paper
正在生成论文摘要