Ego-Network Transformer for Subsequence Classification in Time Series Data.
CoRR(2023)
摘要
Time series classification is a widely studied problem in the field of time series data mining. Previous research has predominantly focused on scenarios where relevant or foreground subsequences have already been extracted, with each subsequence corresponding to a single label. However, real-world time series data often contain foreground subsequences that are intertwined with background subsequences. Successfully classifying these relevant subsequences requires not only distinguishing between different classes but also accurately identifying the foreground subsequences amidst the background. To address this challenge, we propose a novel subsequence classification method that represents each subsequence as an ego-network, providing crucial nearest neighbor information to the model. The ego-networks of all subsequences collectively form a time series subsequence graph, and we introduce an algorithm to efficiently construct this graph. Furthermore, we have demonstrated the significance of enforcing temporal consistency in the prediction of adjacent subsequences for the subsequence classification problem. To evaluate the effectiveness of our approach, we conducted experiments using 128 univariate and 30 multivariate time series datasets. The experimental results demonstrate the superior performance of our method compared to alternative approaches. Specifically, our method outperforms the baseline on 104 out of 158 datasets.
更多查看译文
关键词
time series,graph,subsequence classification
AI 理解论文
溯源树
样例
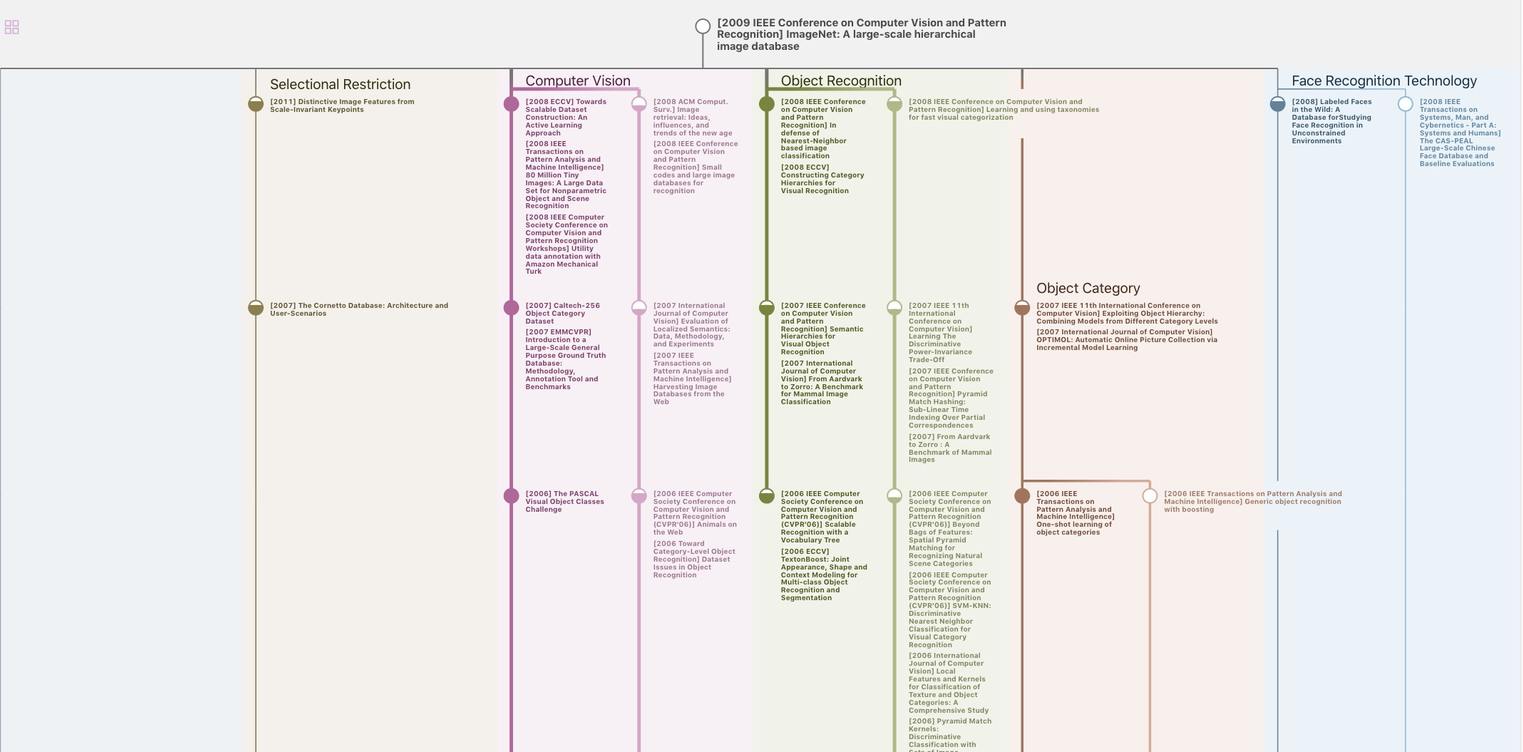
生成溯源树,研究论文发展脉络
Chat Paper
正在生成论文摘要