Fast and Accurate Approximations of the Optimal Transport in Semi-Discrete and Discrete Settings
ACM-SIAM Symposium on Discrete Algorithms(2023)
摘要
Given a $d$-dimensional continuous (resp. discrete) probability distribution $\mu$ and a discrete distribution $\nu$, the semi-discrete (resp. discrete) Optimal Transport (OT) problem asks for computing a minimum-cost plan to transport mass from $\mu$ to $\nu$; we assume $n$ to be the size of the support of the discrete distributions, and we assume we have access to an oracle outputting the mass of $\mu$ inside a constant-complexity region in $O(1)$ time. In this paper, we present three approximation algorithms for the OT problem. (i) Semi-discrete additive approximation: For any $\epsilon>0$, we present an algorithm that computes a semi-discrete transport plan with $\epsilon$-additive error in $n^{O(d)}\log\frac{C_{\max}}{\epsilon}$ time; here, $C_{\max}$ is the diameter of the supports of $\mu$ and $\nu$. (ii) Semi-discrete relative approximation: For any $\epsilon>0$, we present an algorithm that computes a $(1+\epsilon)$-approximate semi-discrete transport plan in $n\epsilon^{-O(d)}\log(n)\log^{O(d)}(\log n)$ time; here, we assume the ground distance is any $L_p$ norm. (iii) Discrete relative approximation: For any $\epsilon>0$, we present a Monte-Carlo $(1+\epsilon)$-approximation algorithm that computes a transport plan under any $L_p$ norm in $n\epsilon^{-O(d)}\log(n)\log^{O(d)}(\log n)$ time; here, we assume that the spread of the supports of $\mu$ and $\nu$ is polynomially bounded.
更多查看译文
AI 理解论文
溯源树
样例
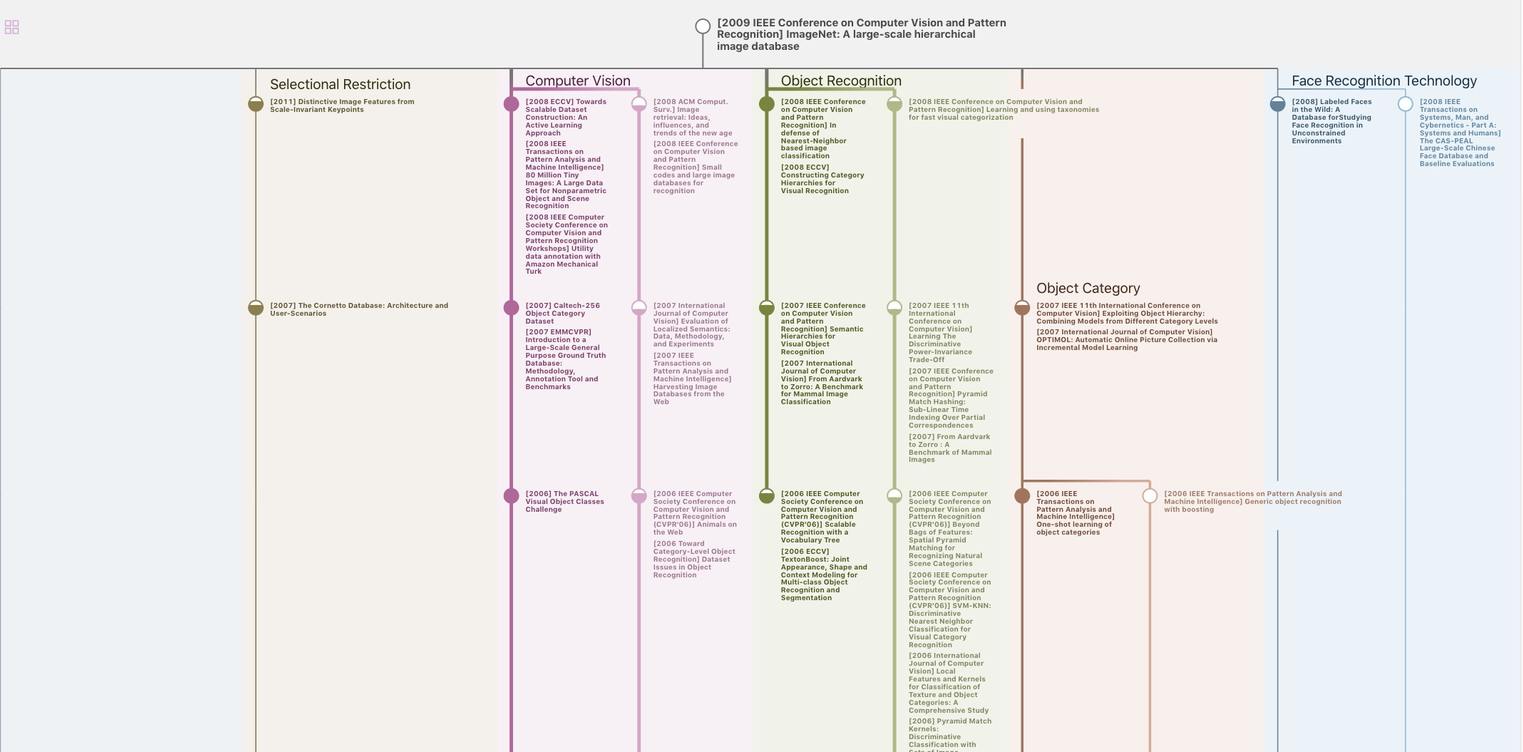
生成溯源树,研究论文发展脉络
Chat Paper
正在生成论文摘要