Eliciting knowledge from language models with automatically generated continuous prompts
EXPERT SYSTEMS WITH APPLICATIONS(2024)
摘要
Pre-trained Language Models (PLMs) have demonstrated remarkable performance in Natural Language Under-standing (NLU) tasks, with continuous prompt-based fine-tuning further enhancing their capabilities. However, current methods rely on hand-crafted discrete prompts to initialize continuous prompts, which are sensitive to subtle changes and inherently limited by the constraints of natural language. To address these limitations, this study introduces an innovative AutoPrompt-based Prompt Tuning (APT) approach. APT optimizes the initialization of continuous prompts by employing a gradient-guided automatic search to generate ideal discrete templates and identify trigger tokens. As the semantic features are already captured from the target task dataset, the continuous parameters initialized by trigger tokens are highly relevant, providing a superior starting point for prompt-tuning. APT searches for optimal prompts across various NLU tasks, enabling the PLM to learn task-related knowledge effectively. The APT method significantly improves PLM performance in both few-shot and fully supervised settings, eliminating the need for extensive prompt engineering. In the knowledge exploration (Language Model Analysis (LAMA)) benchmark, APT achieved a remarkable 58.6% (P@1) performance without additional text, representing a 3.6% improvement over the previous best result. Additionally, APT outperformed state-of-the-art methods in the SuperGLUE benchmark.
更多查看译文
关键词
Prompt learning,Initialization,Trigger token,Continuous parameters
AI 理解论文
溯源树
样例
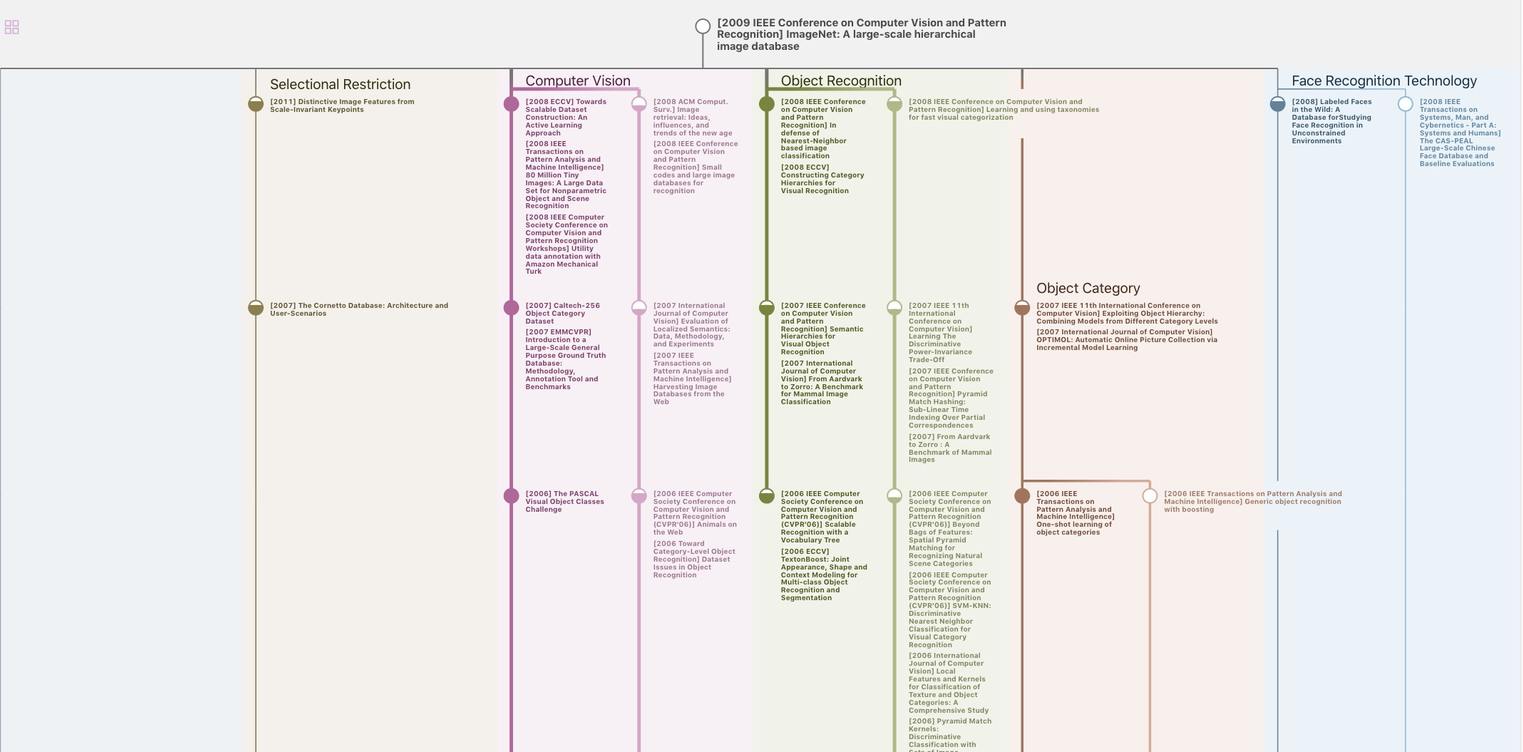
生成溯源树,研究论文发展脉络
Chat Paper
正在生成论文摘要