Deep learning algorithms in predicting Cr(VI) removal performance of S-ZVI: Models building and optimal parameters prediction
Separation and Purification Technology(2024)
摘要
The Cr(VI) removal by sulfidated zero-valent iron (S-ZVI) is a complex process, and reaction conditions can greatly affect Cr(VI) removal performance. To obtain the optimal experimental conditions for removing Cr(VI) by S-ZVI, a significant amount of experimental data is needed which is very laborious. Deep learning is an effective method for predicting unknown or unobservable data and reducing experimental workload. It can build highly complex data models based on existing experimental data through multiple layers of nonlinear transformations. In this study, deep learning was used to establish regression models based on experimental data to predict Cr(VI) removal performance of S-ZVI. Deep neural network (DNN) and long short-term memory (LSTM) algorithms were selected, initial pH and reaction time were input features, and C/C0 of Cr(VI) removal performance was output value. The performance of models built by DNN and LSTM algorithms was investigated by comparing R2 and mean square error. Both models built by DNN and LSTM algorithms exhibited accurate predictive ability. Then DNN and LSTM models were used to predict Cr(VI) removal trend by S-ZVI at different pH and determine optimal pH. As predicted by DNN and LSTM, the optimal removal of Cr(VI) by S-ZVI was achieved when pH was 5.2 and 5.1, respectively. This study provides an effective and promising method for using deep learning modeling to predict Cr(VI) removal performance of S-ZVI. It is expected to simplify the preliminary experiments and reduce labor, time and chemical costs.
更多查看译文
关键词
Sulfidated zero-valent iron,Deep neural network,Long short-term memory,Hexavalent chromium Cr(VI),pH
AI 理解论文
溯源树
样例
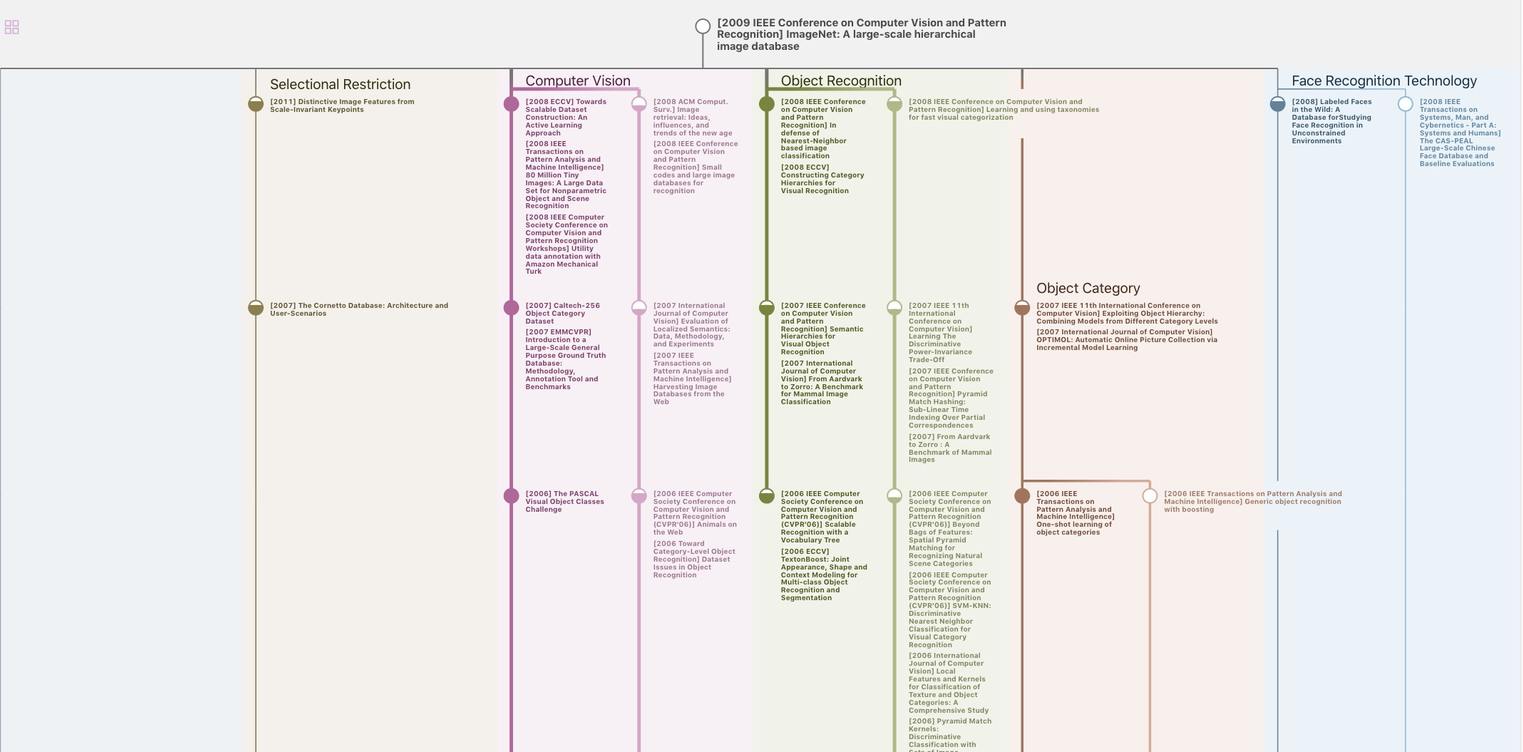
生成溯源树,研究论文发展脉络
Chat Paper
正在生成论文摘要