Mask Again: Masked Knowledge Distillation for Masked Video Modeling
MM '23: Proceedings of the 31st ACM International Conference on Multimedia(2023)
摘要
Masked video modeling has shown remarkable performance in downstream tasks by predicting masked video tokens from visible ones. However, training models from scratch on large-scale unlabeled data remains computationally challenging and time-consuming. Moreover, the commonly used random-based sampling techniques may lead to the selection of redundant or low-information regions, hindering the model from learning discriminative representations within the limited training epochs. To achieve efficient pre-training, we propose MaskAgain, an efficient feature-based knowledge distillation framework for masked video pre-training that facilitates knowledge transfer from a pre-trained teacher model to a student model. In contrast to previous approaches that align all visible token features with the teacher model at output layers, MaskAgain adopts a selective approach by masking visible tokens again at both the hidden and output layers of the transformer block. Attention mechanisms are utilized for informative feature selection. At the hidden level, attention maps generated by the transformer's multi-head attention structure are utilized to select crucial token information at both temporally-global and temporally-local levels. Additionally, at the output level, an activation-based attention map is generated using token features, enabling us to focus on important tokens while preserving feature similarity and the relationship matrix similarity between patches. Extensive experimental results show that MaskAgain achieves comparable or even better performance than existing methods on benchmark datasets with much fewer training epochs and much less memory, which demonstrates that MaskAgain allows for efficient pre-training of accurate video models, reducing computational resources and training time significantly. Code is released at https://github.com/xiaojieli0903/MaskAgain.
更多查看译文
AI 理解论文
溯源树
样例
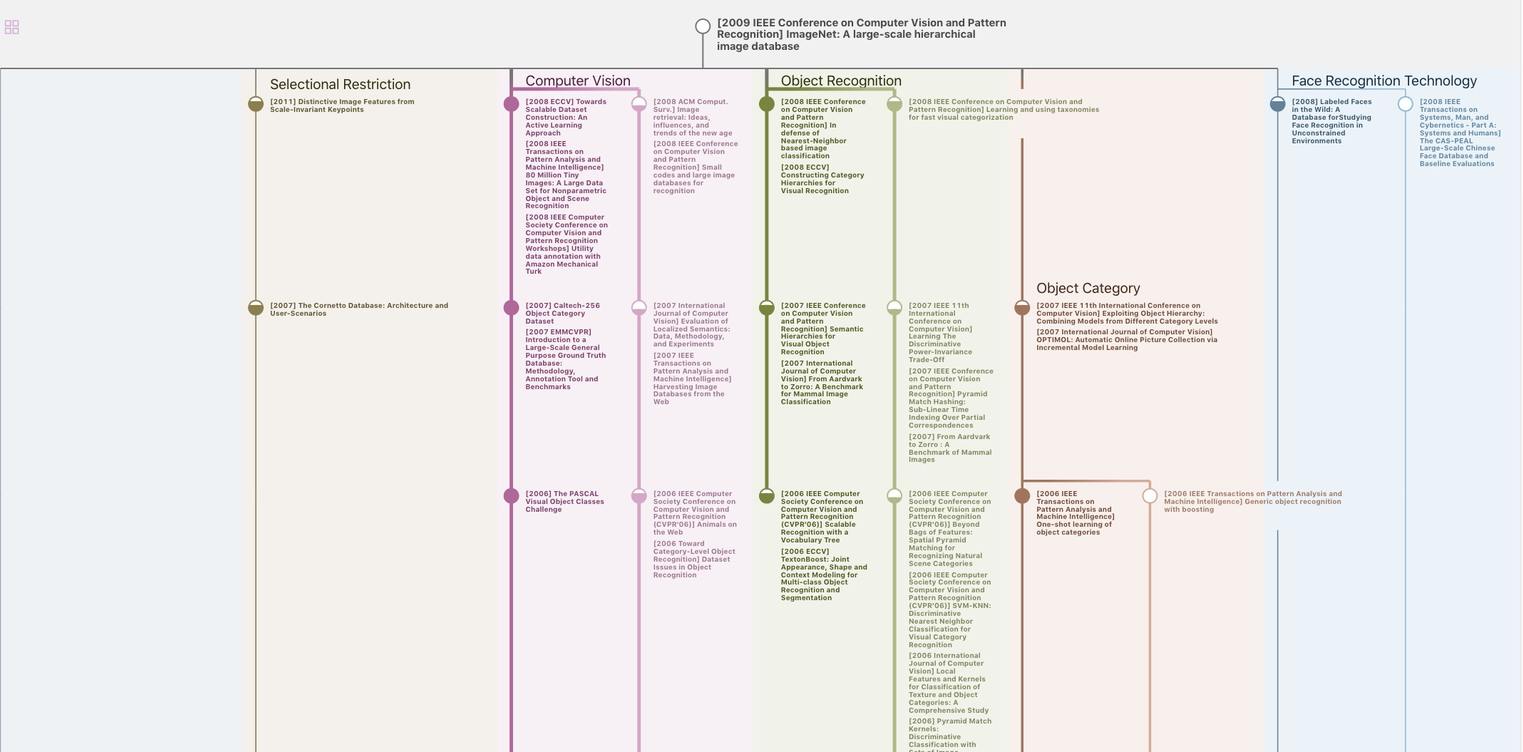
生成溯源树,研究论文发展脉络
Chat Paper
正在生成论文摘要