Exploring Motion Cues for Video Test-Time Adaptation
MM '23: Proceedings of the 31st ACM International Conference on Multimedia(2023)
摘要
Test-time adaptation (TTA) aims at boosting the generalization capability of a trained model by conducting self-/un-supervised learning during testing in real-world applications. Though TTA on image-based tasks has seen significant progress, TTA techniques for video remain scarce. Naively introducing image-based TTA methods into video tasks may achieve limited performance, since these methods do not consider the special nature of video tasks, e.g., the motion information. In this paper, we propose leveraging motion cues in videos to design a new test-time learning scheme for video classification. We extract spatial appearance and dynamic motion clip features using two sampling rates (i.e., slow and fast) and propose a fast-to-slow unidirectional alignment scheme to align fast motion and slow appearance features, thereby enhancing the motion encoding ability. Additionally, we propose a slow-fast dual contrastive learning strategy to learn a joint feature space for fastly and slowly sampled clips, guiding the model to extract discriminative video features. Lastly, we introduce a stochastic pseudo-negative sampling scheme to provide better adaptation supervision by selecting a more reliable pseudo-negative label compared to the pseudo-positive label used in prior TTA methods. This technique reduces the adaptation difficulty often caused by poor performance on out-of-distribution test data before adaptation. Our approach significantly improves performance on various video classification backbones, as demonstrated through extensive experiments on two benchmark datasets.
更多查看译文
AI 理解论文
溯源树
样例
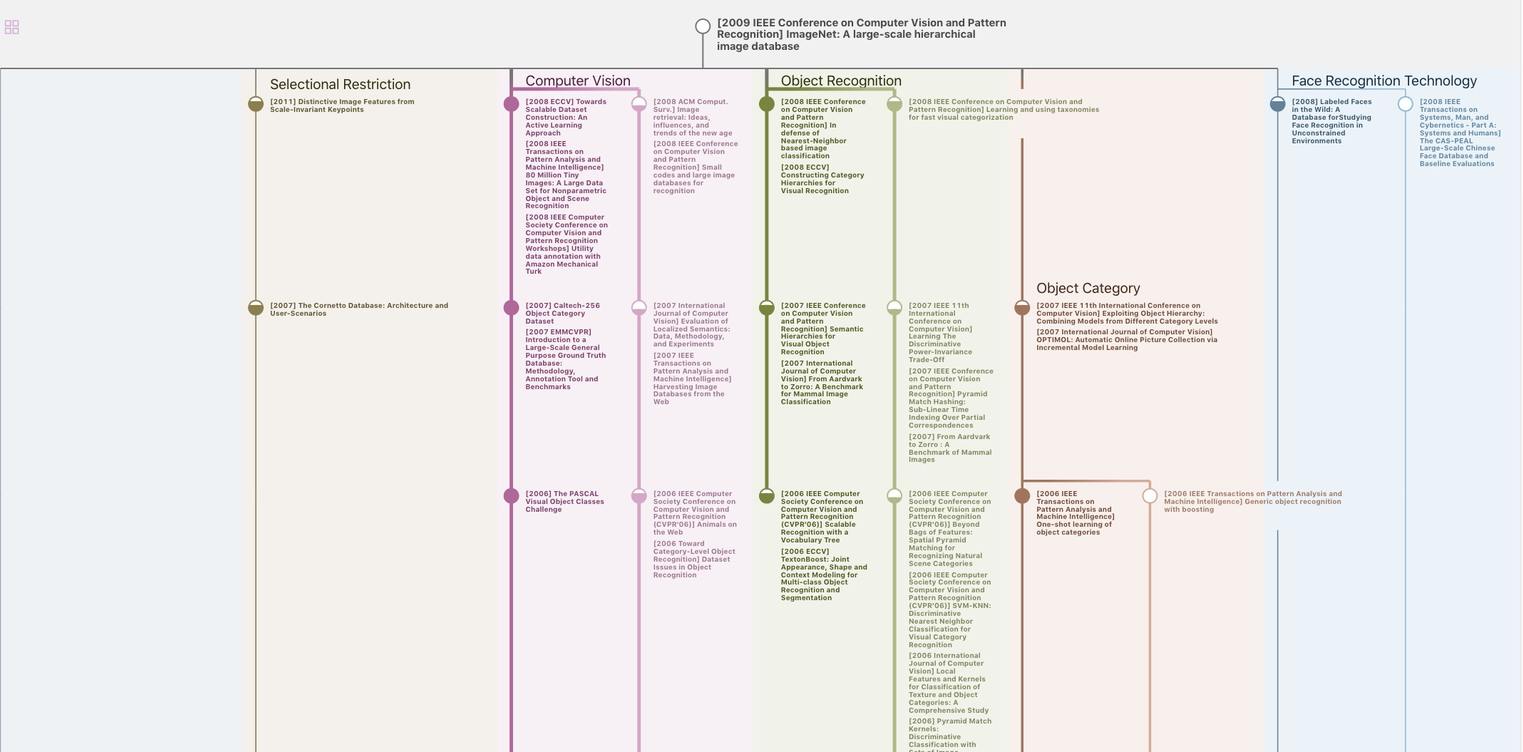
生成溯源树,研究论文发展脉络
Chat Paper
正在生成论文摘要