Fine-grained Key-Value Memory Enhanced Predictor for Video Representation Learning
MM '23: Proceedings of the 31st ACM International Conference on Multimedia(2023)
摘要
Self-supervised learning methods have shown significant promise in acquiring robust spatiotemporal representations from unlabeled videos. In this work, we address three critical limitations in existing self-supervised video representation learning: 1) insufficient utilization of contextual information and lifelong memory, 2) lack of fine-grained visual concept alignment, and 3) neglect of the feature distribution gap between encoders. To overcome these limitations, we propose a novel memory-enhanced predictor that leverages key-value memory networks with separate memories for the online and target encoders. This design enables the effective storage and retrieval of contextual knowledge, facilitating informed predictions and enhancing overall performance. Additionally, we introduce a visual concept alignment module that ensures fine-grained alignment of shared semantic information across segments of the same video. By employing coupled dictionary learning, we effectively decouple visual concepts, enriching the semantic representation stored in the memory networks. Our proposed approach is extensively evaluated on widely recognized benchmarks for action recognition and retrieval tasks, demonstrating its superiority in learning generalized video representations with significantly improved performance compared to existing state-of-the-art self-supervised learning methods. Code is released at https://github.com/xiaojieli0903/FGKVMemPred_video.
更多查看译文
AI 理解论文
溯源树
样例
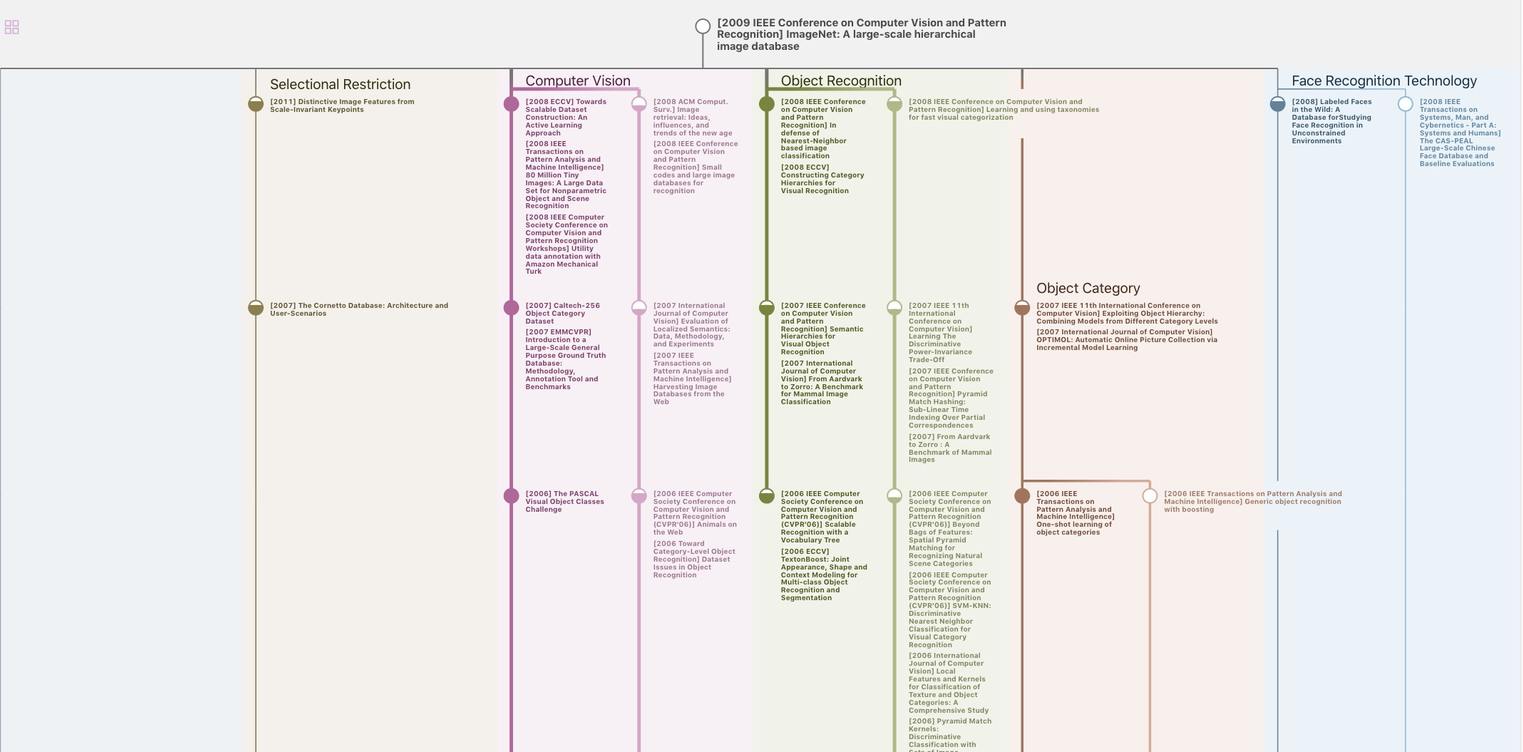
生成溯源树,研究论文发展脉络
Chat Paper
正在生成论文摘要