Power Minimization in Federated Learning with Over-the-air Aggregation and Receiver Beamforming
PROCEEDINGS OF THE INT'L ACM CONFERENCE ON MODELING, ANALYSIS AND SIMULATION OF WIRELESS AND MOBILE SYSTEMS, MSWIM 2023(2023)
摘要
Combining over-the-air uplink transmission and multi-antenna beamforming can improve the efficiency of federated learning (FL). However, to mitigate the significant aggregation error due to communication noise and signal distortion, pre-processing of device signals and post-processing at the server are required. In this paper, we study the optimization of receiver beamforming and device transmit weights in over-the-air FL, to minimize the total transmit power in each communication round while guaranteeing the convergence of FL. We establish sufficient convergence conditions based on the analysis of gradient descent with error and formulate a power minimization problem. An alternating optimization approach is then employed to decompose the problem into tractable subproblems, and efficient solutions are developed for these subproblems. Our proposed method is evaluated through simulation on standard image classification tasks, demonstrating its effectiveness in achieving substantial reductions in transmit power compared with existing alternatives.
更多查看译文
关键词
Federated Learning,Over-the-air Computation,Power Consumption,Multi-antenna Beamforming
AI 理解论文
溯源树
样例
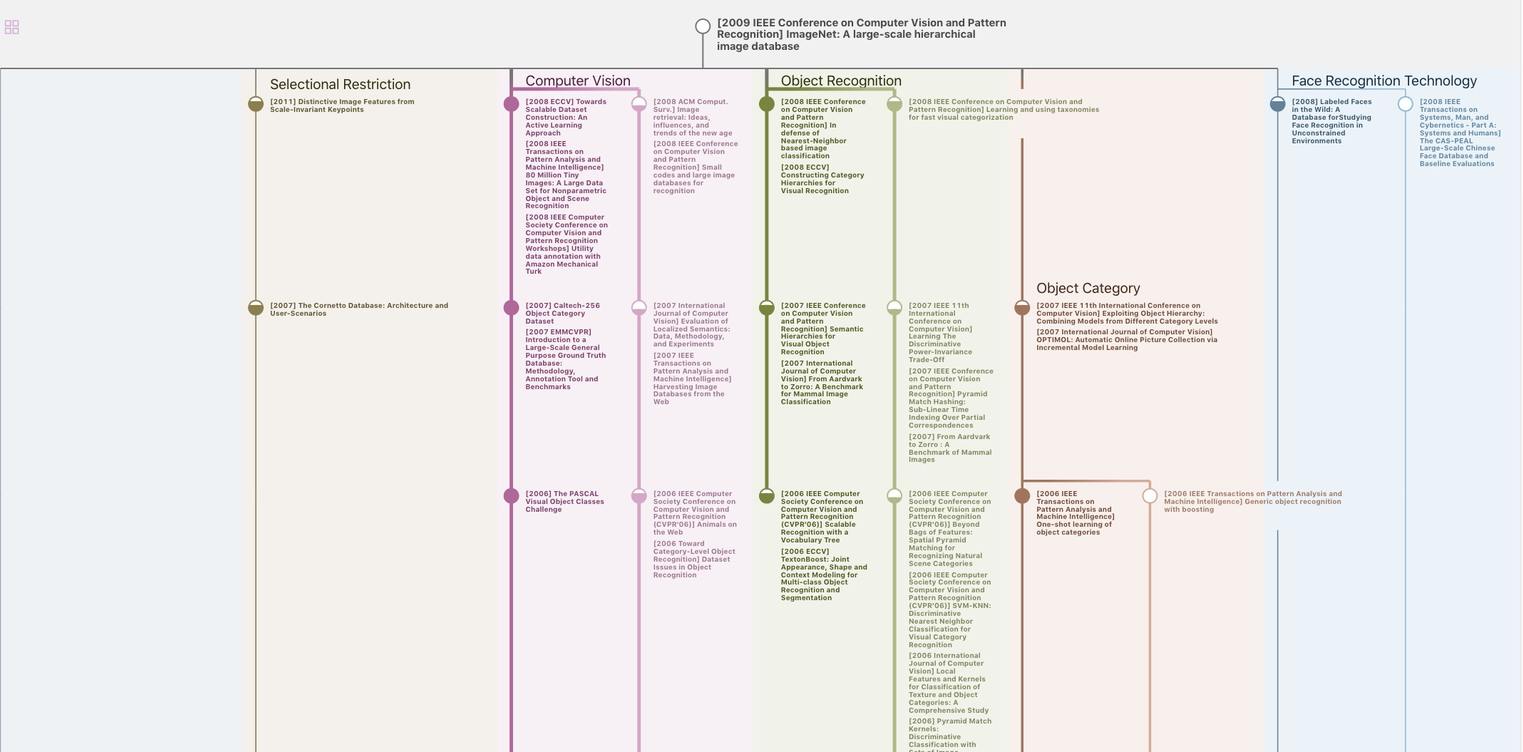
生成溯源树,研究论文发展脉络
Chat Paper
正在生成论文摘要