How Fresh is the Data? An Optimal Learning-Based End-to-End Pull-Based Forwarding Framework for NDNoTs
PROCEEDINGS OF THE INT'L ACM CONFERENCE ON MODELING, ANALYSIS AND SIMULATION OF WIRELESS AND MOBILE SYSTEMS, MSWIM 2023(2023)
摘要
The Named Data Networks (NDNs) are considered as a suitable architectural paradigm for collecting the sensory data generated by the Internet of Things (IoTs). However, towards retrieving fresh ephemeral sensory data, we show that the naive integration of NDN and IoT/sensor, without using learning-based forwarding methods, is energy-demanding and sub-optimal in retrieving fresh data. To this end, a novel learning-based NDNoT data forwarding framework is proposed. We analytically show that a Reinforcement Learning (RL) based NDN forwarding strategy can optimally retrieve fresh sensory data from IoTs in an energy-efficient manner. The proposed NDN forwarding strategy is modelled as an optimal-stopping problem using Multi-Armed Bandit (MABs). The structural result of the MAB is investigated to yield optimal policy in terms of both energy efficiency and fresh data delivery. The resultant optimal policy implemented in the form of an NDNoT forwarding algorithm namely, FRESH is validated and compared against the state-of-the-art NDN forwarding strategies using extensive simulation.
更多查看译文
关键词
NDN,IoT,MAB,RL,performance modeling,data freshness,energy efficiency,learning based forwarding
AI 理解论文
溯源树
样例
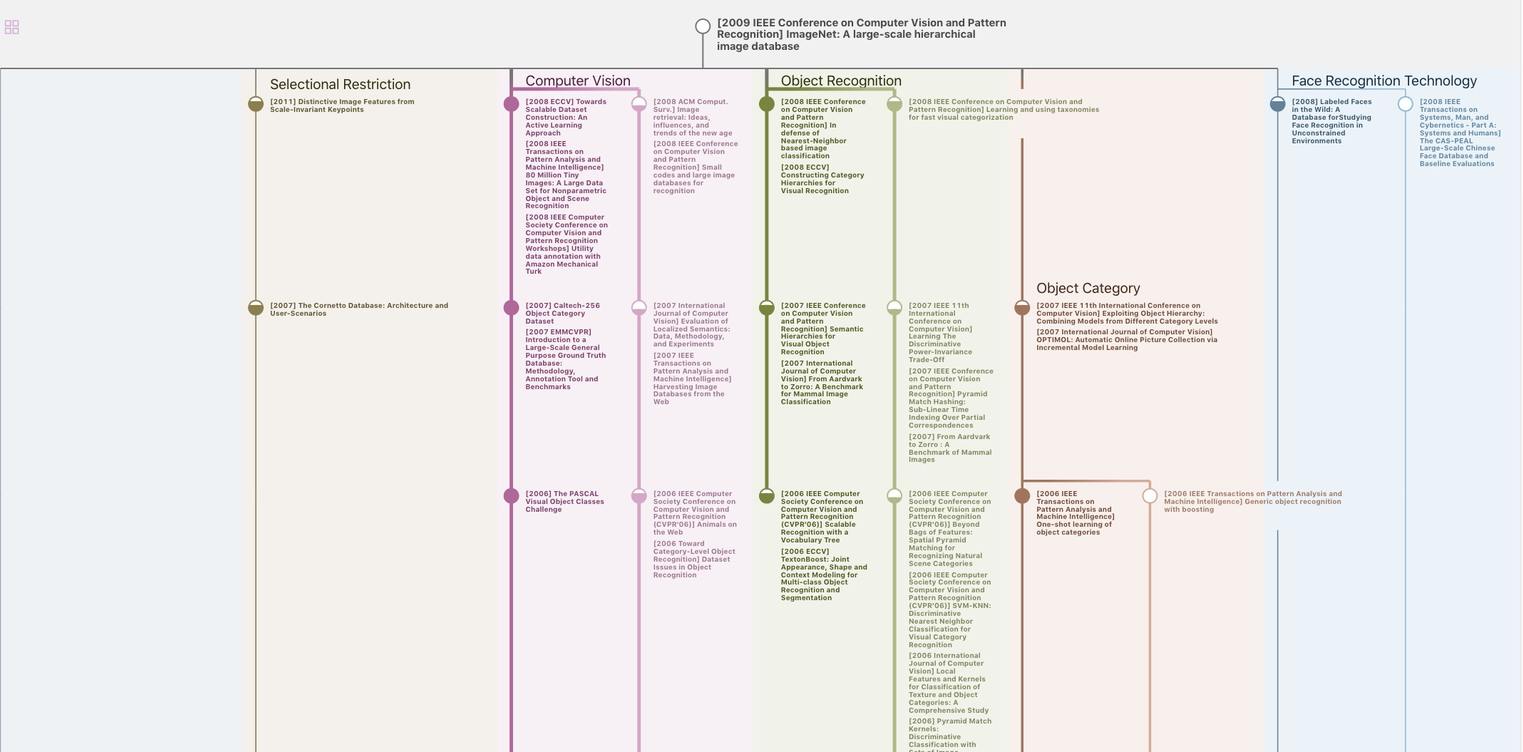
生成溯源树,研究论文发展脉络
Chat Paper
正在生成论文摘要