Accurate E-CID Framework for Indoor Positioning in 5G using Path Tracing and Machine Learning
PROCEEDINGS OF THE INT'L ACM CONFERENCE ON MODELING, ANALYSIS AND SIMULATION OF WIRELESS AND MOBILE SYSTEMS, MSWIM 2023(2023)
摘要
Locating at-risk workers in hospitals using legacy private 5G networks is a daunting task that involves solving the problem of indoor localization using commercial off-the-shelf proprietary hardware. Currently, no full-stack schemes or realistic indoor positioning experiments have been conducted using 5G. In this study, we present the first comprehensive 5G framework that combines fingerprinting with the 3GPP Enhanced Cell ID (E-CID) approach. Our methodology consists of a machine-learning model to deduce the user's position by comparing the signal strength received from the User Equipment (UE) with a reference radio power map. This challenging method has four main contributions. First, the 3GPP protocols and functions are extended to provide open, secure, and universal core network-based localization functions. Second, to generate a reference map, the first paradigm of Optical Radio Power Estimation using Light Analysis (ORPELA) is introduced. Real-world experiments prove that it is reproducible and more accurate than state-of-the-art radio-planning software. Third, machine-learning models are designed, trained, and optimized for an ultra-challenging radio context. Finally, an extensive experimental campaign is conducted to demonstrate the expected indoor localization performance of realistic 5G private networks.
更多查看译文
关键词
Indoor Positioning,5G,Path Tracing,Real-Word Experiment
AI 理解论文
溯源树
样例
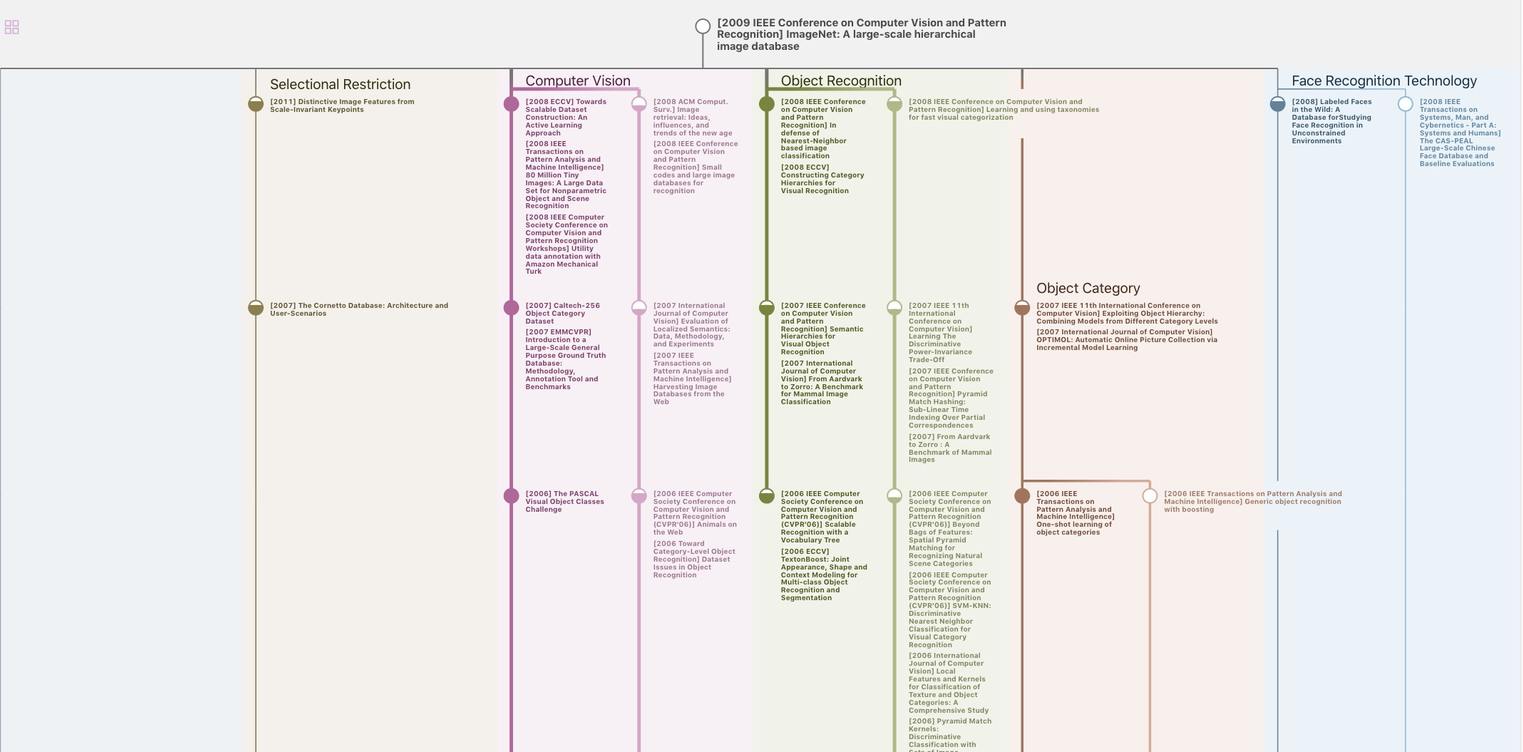
生成溯源树,研究论文发展脉络
Chat Paper
正在生成论文摘要