DPS: Adaptive Power Management for Overprovisioned Systems
SC '23: Proceedings of the International Conference for High Performance Computing, Networking, Storage and Analysis(2023)
摘要
Maximizing performance under a power budget is essential for HPC systems and has inspired the development of many power management frameworks. These can be broadly characterized into two groups: model-based and stateless. Model-based frameworks use machine learning to achieve good performance under a power budget but are highly dependent on the quality of the learned model and the data used to train it. Stateless frameworks are more robust and require no training, but are generally lower performance. In this paper, we propose a new framework that does not require a model, but does track a small amount of state in the form of recent power dynamics. We implement this idea and test it on a public cloud running both Spark and HPC jobs. We find when total power demand is low, our framework achieves equivalent performance to prior work, but when power demand is high it achieves mean 8% performance improvement (with no reliance on a learned model).
更多查看译文
关键词
power-efficient design and power-management strategies,resource management,job scheduling,system interoperations and energy-aware techniques for large-scale systems
AI 理解论文
溯源树
样例
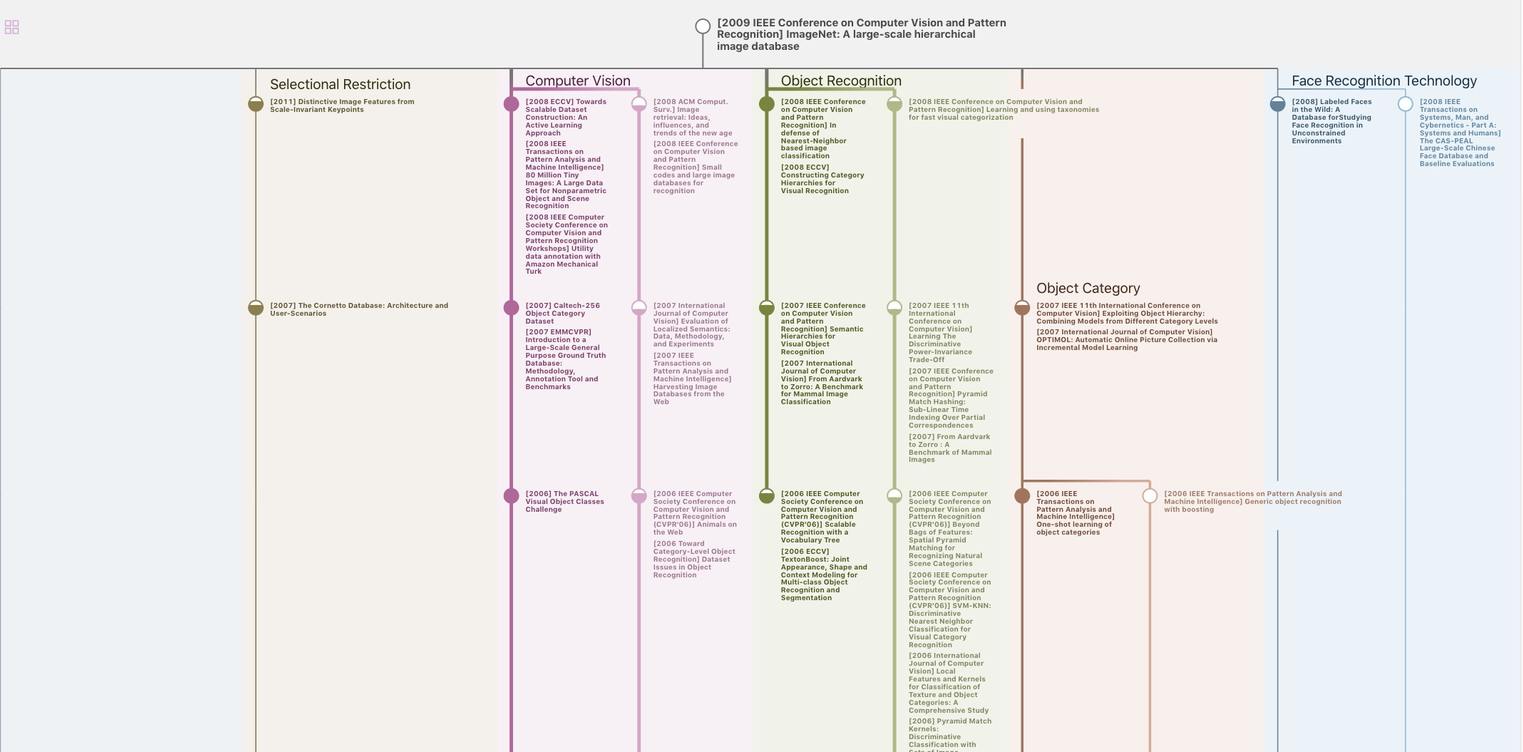
生成溯源树,研究论文发展脉络
Chat Paper
正在生成论文摘要