Application Performance Modeling via Tensor Completion
SC '23: Proceedings of the International Conference for High Performance Computing, Networking, Storage and Analysis(2023)
摘要
Performance tuning, software/hardware co-design, and job scheduling are among the many tasks that rely on models to predict application performance. We propose and evaluate low-rank tensor decomposition for modeling application performance. We discretize the input and configuration domains of an application using regular grids. Application execution times mapped within grid-cells are averaged and represented by tensor elements. We show that low-rank canonical-polyadic (CP) tensor decomposition is effective in approximating these tensors. We further show that this decomposition enables accurate extrapolation of unobserved regions of an application's parameter space. We then employ tensor completion to optimize a CP decomposition given a sparse set of observed execution times. We consider alternative piecewise/grid-based models and supervised learning models for six applications and demonstrate that CP decomposition optimized using tensor completion offers higher prediction accuracy and memory-efficiency for high-dimensional performance modeling.
更多查看译文
关键词
performance,application,modeling
AI 理解论文
溯源树
样例
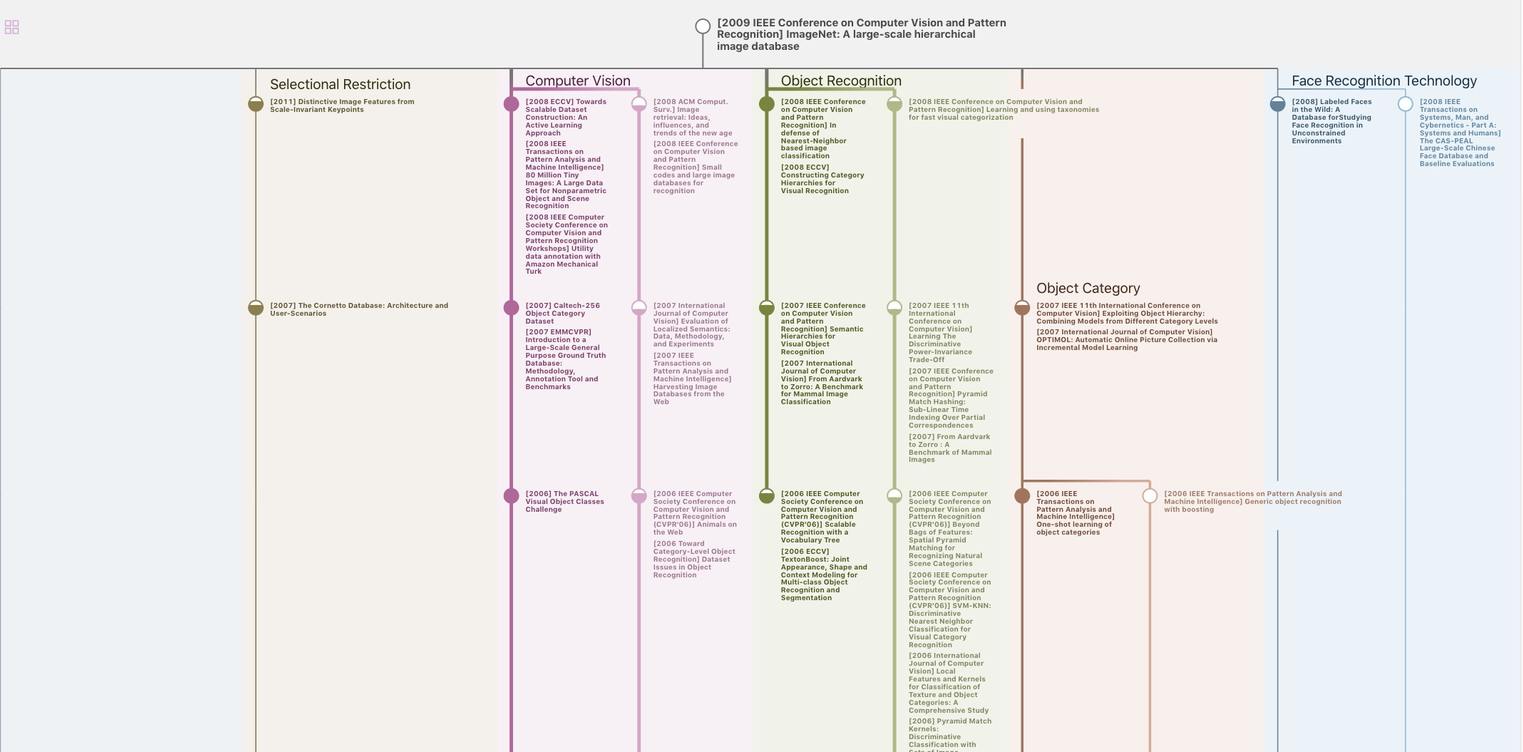
生成溯源树,研究论文发展脉络
Chat Paper
正在生成论文摘要