SoftDECA: Computationally Efficient Physics-Based Facial Animations
15TH ANNUAL ACM SIGGRAPH CONFERENCE ON MOTION, INTERACTION AND GAMES, MIG 2023(2023)
摘要
Facial animation on computationally weak systems is still mostly dependent on linear blendshape models. However, these models suffer from typical artifacts such as loss of volume, self-collisions, or erroneous soft tissue elasticity. In addition, while extensive effort is required to personalize blendshapes, there are limited options to simulate or manipulate physical and anatomical properties once a model has been crafted. Finally, second-order dynamics can only be represented to a limited extent. For decades, physics-based facial animation has been investigated as an alternative to linear blendshapes but is still cumbersome to deploy and results in high computational cost at runtime. We propose SoftDECA, an approach that provides the benefits of physics-based simulation while being as effortless and fast to use as linear blendshapes. SoftDECA is a novel hypernetwork that efficiently approximates a FEM-based facial simulation while generalizing over the comprehensive DECA model of human identities, facial expressions, and a wide range of material properties that can be locally adjusted without re-training. Along with SoftDECA, we introduce a pipeline for creating the needed high-resolution training data. Part of this pipeline is a novel layered head model that densely positions the biomechanical anatomy within a skin surface while avoiding self-intersections.
更多查看译文
关键词
Facial Animation,Physics-Based Simulation,Deep Learning
AI 理解论文
溯源树
样例
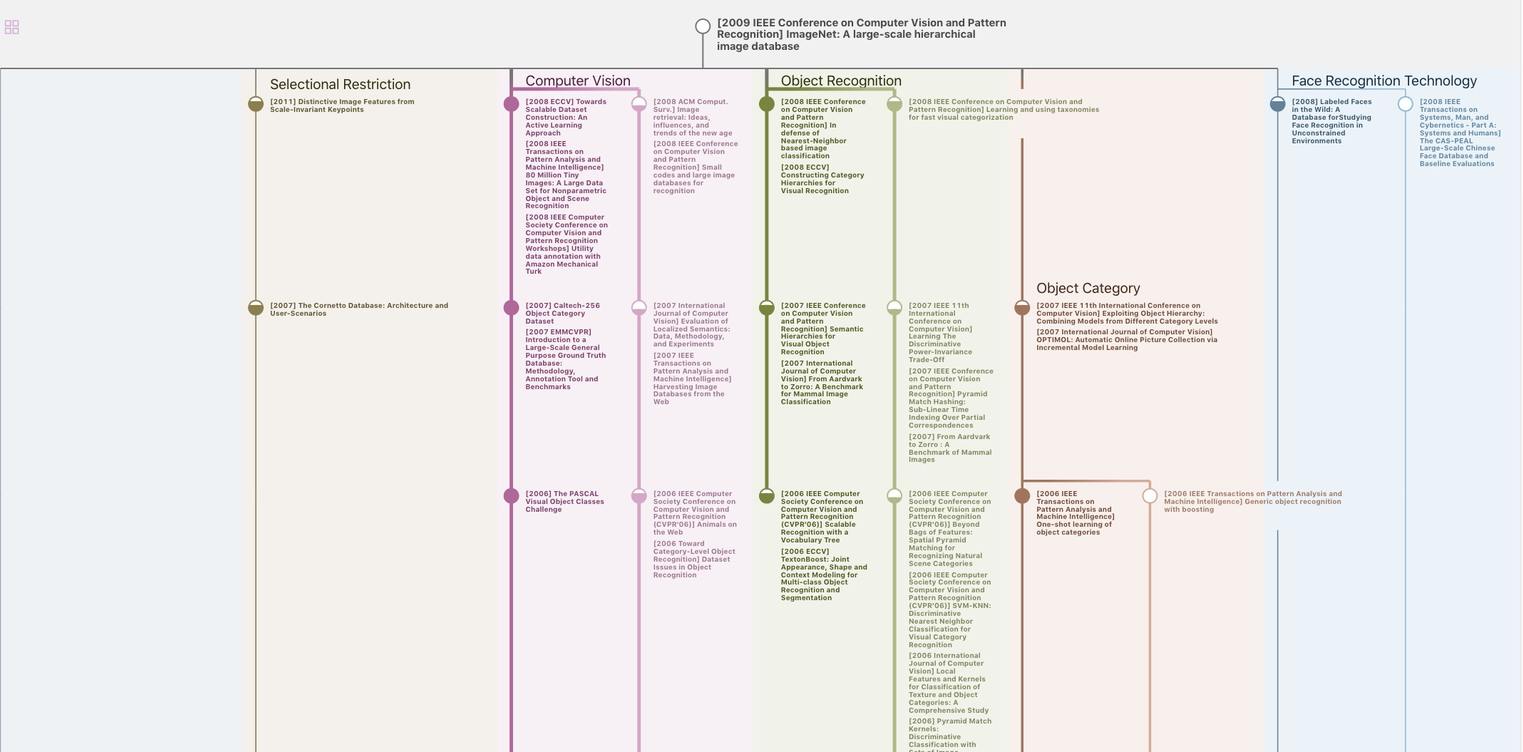
生成溯源树,研究论文发展脉络
Chat Paper
正在生成论文摘要