Are ML Models Scenario-Independent in Enhancing Routing Efficiency for Smart Grid Networks?
PROCEEDINGS OF THE INT'L ACM SYMPOSIUM ON PERFORMANCE EVALUATION OF WIRELESS AD HOC, SENSOR, & UBIQUITOUS NETWORKS, PE-WASUN 2023(2023)
摘要
This study aims to achieve two objectives. Firstly, it evaluates the performance of various traditional machine learning (ML) techniques using two datasets containing Quality of Service (QoS) metrics obtained from real-world and synthetic Grid scenarios, both utilizing the Routing Protocol for Low-Power and Lossy Networks (RPL) in Wireless Smart Grid Networks (WSGNs). Secondly, it investigates how different scenarios impact the performance of ML models, considering their potential integration within the widely adopted RPL. Initially, the performance of multiple traditional ML techniques was assessed to determine the most effective one. Subsequently, two distinct models were created, one for each generated dataset, using the most effective technique. The findings highlight that the Long Short-Term Memory (LSTM) technique outperforms all other techniques, achieving an AUC (Area Under the Curve) value of >=0.98. Furthermore, an important discovery emerged from this research, indicating that the performance of the ML model diminishes when applied to a scenario different from the one it was trained on. This effect is particularly notable when transitioning from a real-world context to a simplified grid scenario.
更多查看译文
关键词
Wireless smart grid networks,routing protocols,machine learning
AI 理解论文
溯源树
样例
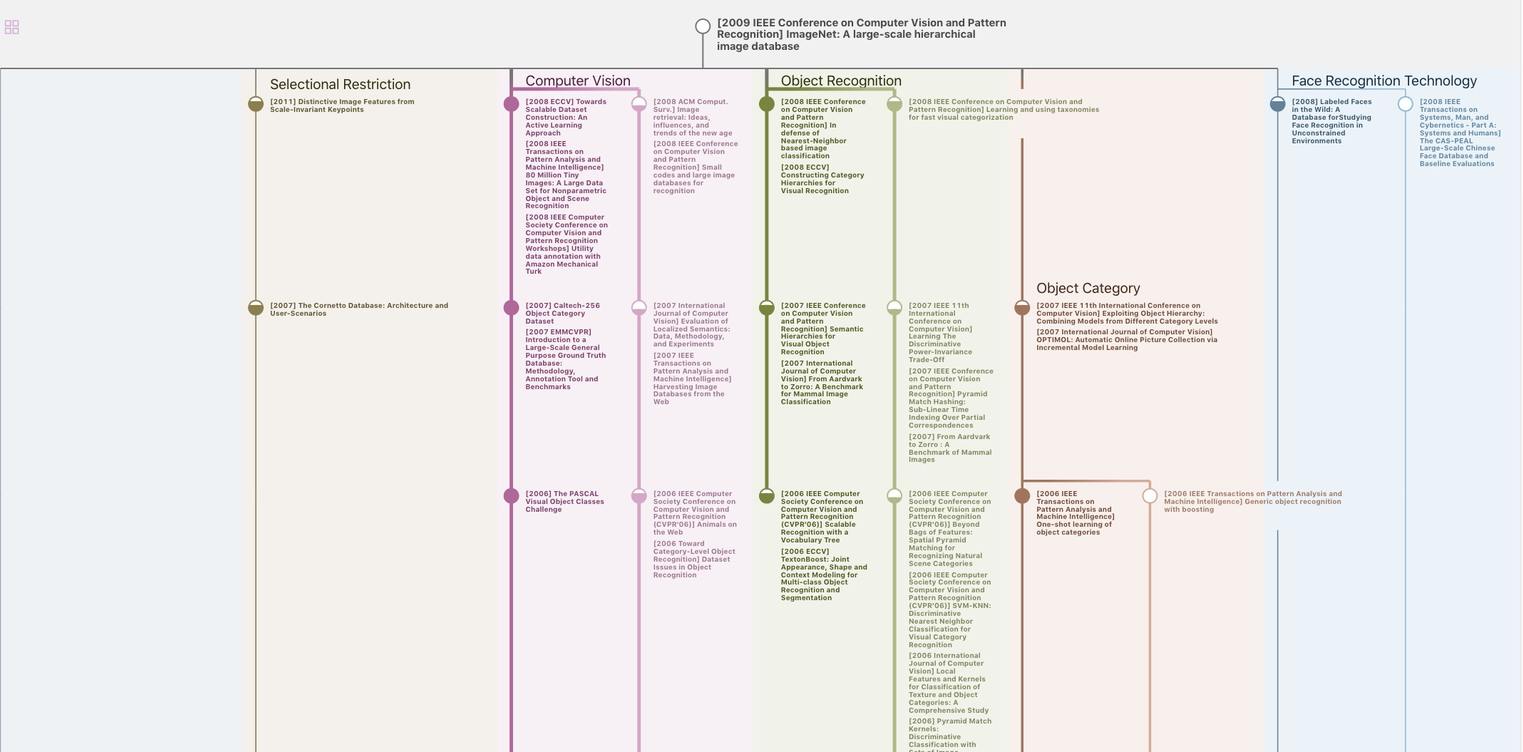
生成溯源树,研究论文发展脉络
Chat Paper
正在生成论文摘要