fKPISelect: Fault-Injection Based Automated KPI Selection for Practical Multivariate Anomaly Detection
2023 IEEE 34th International Symposium on Software Reliability Engineering (ISSRE)(2023)
摘要
IT services are now popularly hosted in cloud systems. In order to enhance the availability of cloud services, an emerging approach for detecting failures of cloud components is to monitor Key Performance Indicators (KPIs) of the components and apply Neural Network based AI technologies to detect KPI anomalies. Multivariate Time Series Anomaly Detection (TSAD) models have been designed for this purpose. However, when applying such models directly to real-world cloud systems the anomaly detection performance is not as good. This is because the number of KPIs in real cloud systems is typically much more than the number of KPIs in the datasets used for model evaluation, and the larger number of KPIs bring about a performance loss of the models’ anomaly detection. Therefore, selecting KPIs properly is essential for applying multivariant KPI data for any practical anomaly detection. This paper studies this performance loss issue when TSAD models are applied onto real-world cloud systems, and proposes fKPISelect, a mechanism of automated KPI selection based on fault injection. We implemented fKPISelect, deployed it to a real cloud system, and created a real-world KPI dataset. We conducted extensive experiments, and the experimental results show the effectiveness and practicality of fKPISelect: it improves the F1 score of anomaly detection from 0.68 to 0.91 for real-world KPI data.
更多查看译文
关键词
anomaly detection,cloud reliability,unsupervised learning,KPI,multivariant analysis
AI 理解论文
溯源树
样例
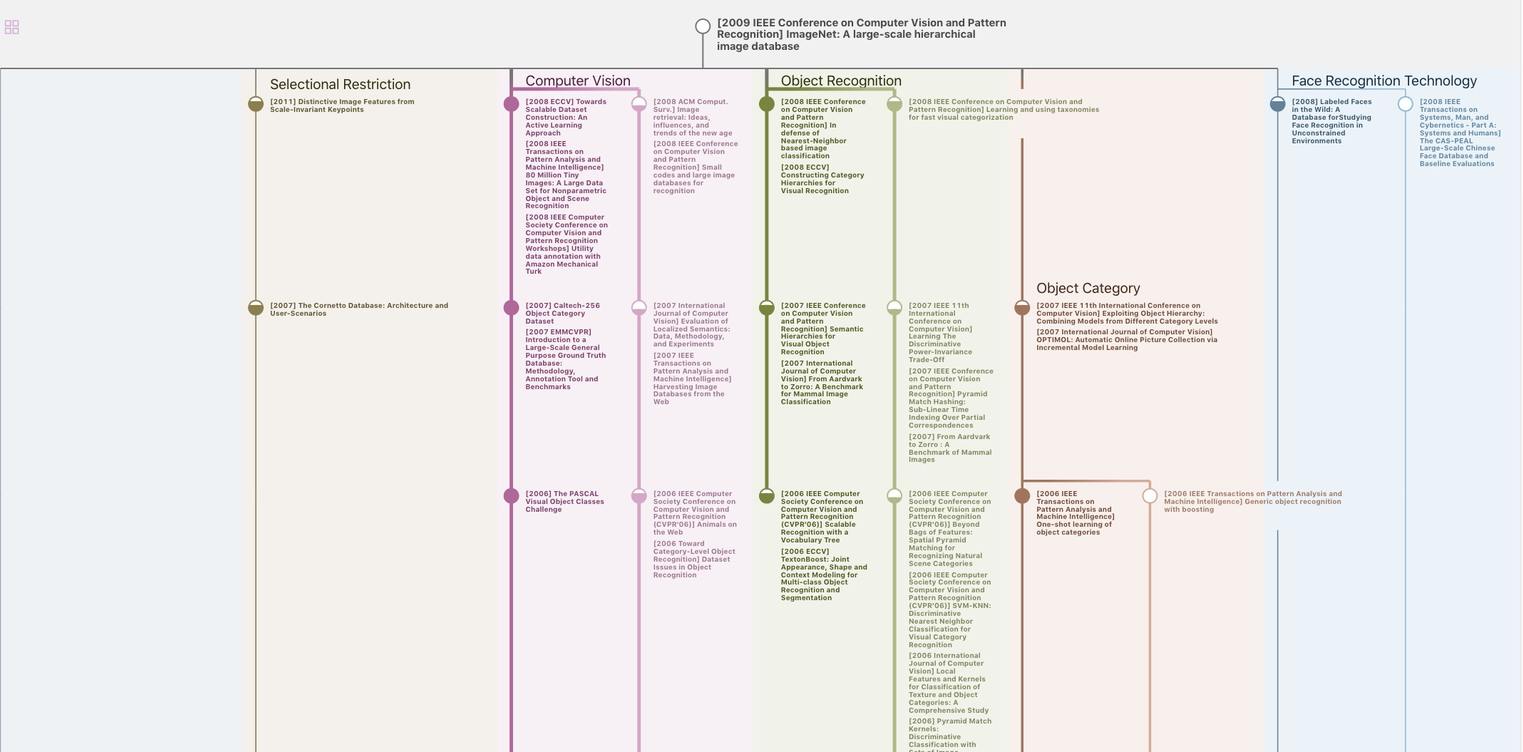
生成溯源树,研究论文发展脉络
Chat Paper
正在生成论文摘要