On the performance of screening invalid jobs using the policy gradient method in deadline-aware data transfer scheduling
2023 14th International Conference on Network of the Future (NoF)(2023)
摘要
When transferring large amounts of data between data centers using residual bandwidth, some transfers must be completed before the deadline. Such data transfers are called deadline-aware jobs. Scheduling deadline-aware jobs is not easy, and existing methods such as Earliest Deadline First (EDF) are not optimal because they do not consider jobs that will arrive in the future. In this paper, we apply a policy-gradient deep reinforcement learning algorithm to schedule the deadline-aware job scheduling. We set conditions under which EDF cannot achieve optimal job scheduling, and show the proposed policy-gradient deep reinforcement learning algorithm outperforms the EDF method in this scenario. By varying the number of jobs and job size, we increased the job difficulty and investigated how the proposed method behaved. The results show that as the job difficulty is increased, the proposed method performs worse than EDF, although it still performs better. There is still room for improvement when applying reinforcement learning to this task.
更多查看译文
关键词
deadline-aware data transfer,policy-gradient,reinforcement learning,earliest-due-first
AI 理解论文
溯源树
样例
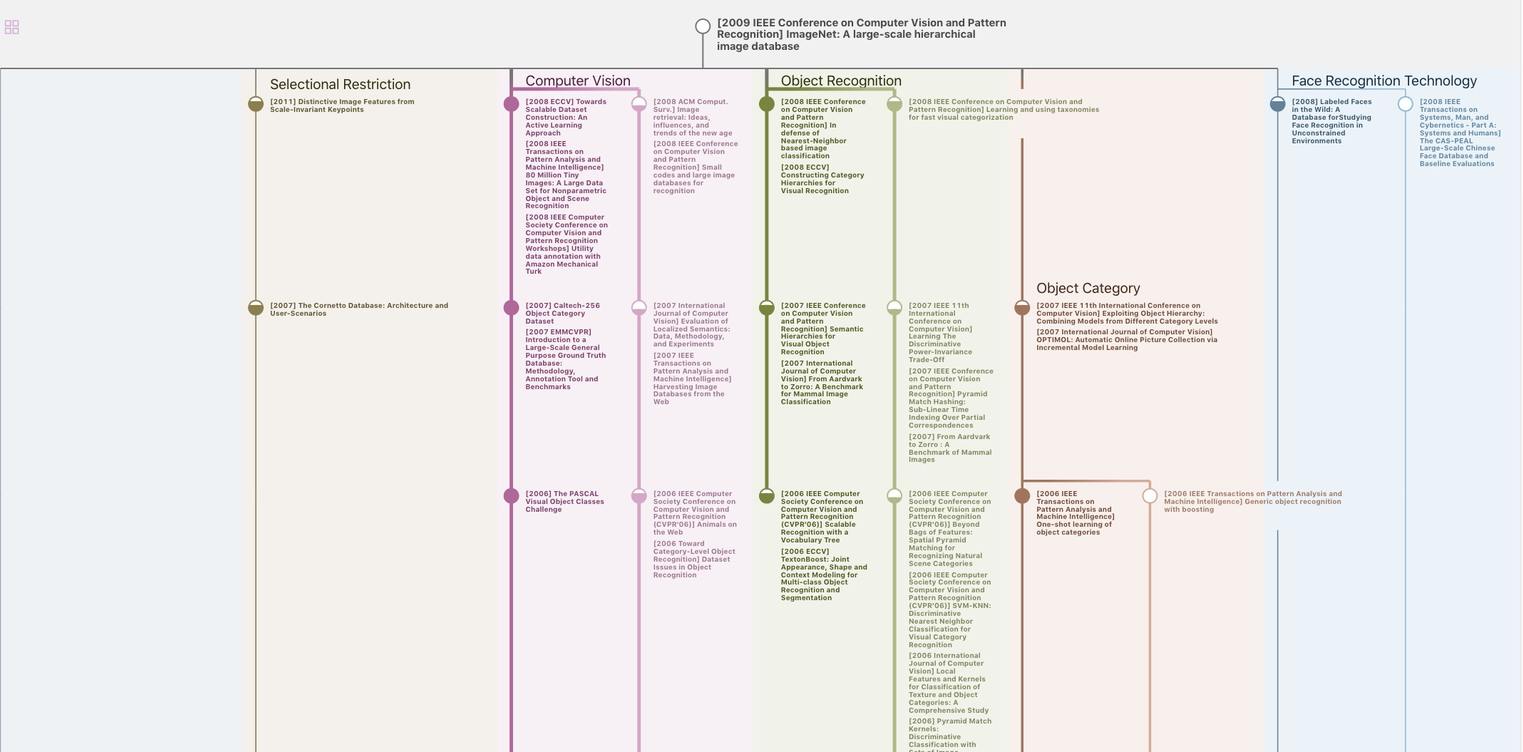
生成溯源树,研究论文发展脉络
Chat Paper
正在生成论文摘要