A knowledge-constrained CNN-BiLSTM model for lithium-ion batteries state-of-charge estimation
Microelectronics Reliability(2023)
摘要
Deep learning (DL) has shown good performance in state-of-charge (SOC) estimation for lithium-ion batteries. However, the requirement for massive data and the instability of results hinder the application of DL in SOC estimation. In this paper, we propose a novel Knowledge-Constrained CNN-BiLSTM model (KCCL), which is more robust and performs better when the amount of training data is not sufficient. In this model, we incorporate knowledge-based constraints into the model training process, by which both statistical knowledge and physical knowledge are integrated. By doing so, we guide the model to focus not only on the accuracy of individual data points, but also on the physical relationships between data points, resulting in more accurate and reliable SOC estimation. We evaluate the performance of proposed KCCL model using a public dataset and a dataset collected from an energy storage battery used in real practice, and demonstrate that it outperforms model without knowledge-constraint, particularly when the amount of training data is limited.
更多查看译文
关键词
batteries,knowledge-constrained,cnn-bilstm,lithium-ion,state-of-charge
AI 理解论文
溯源树
样例
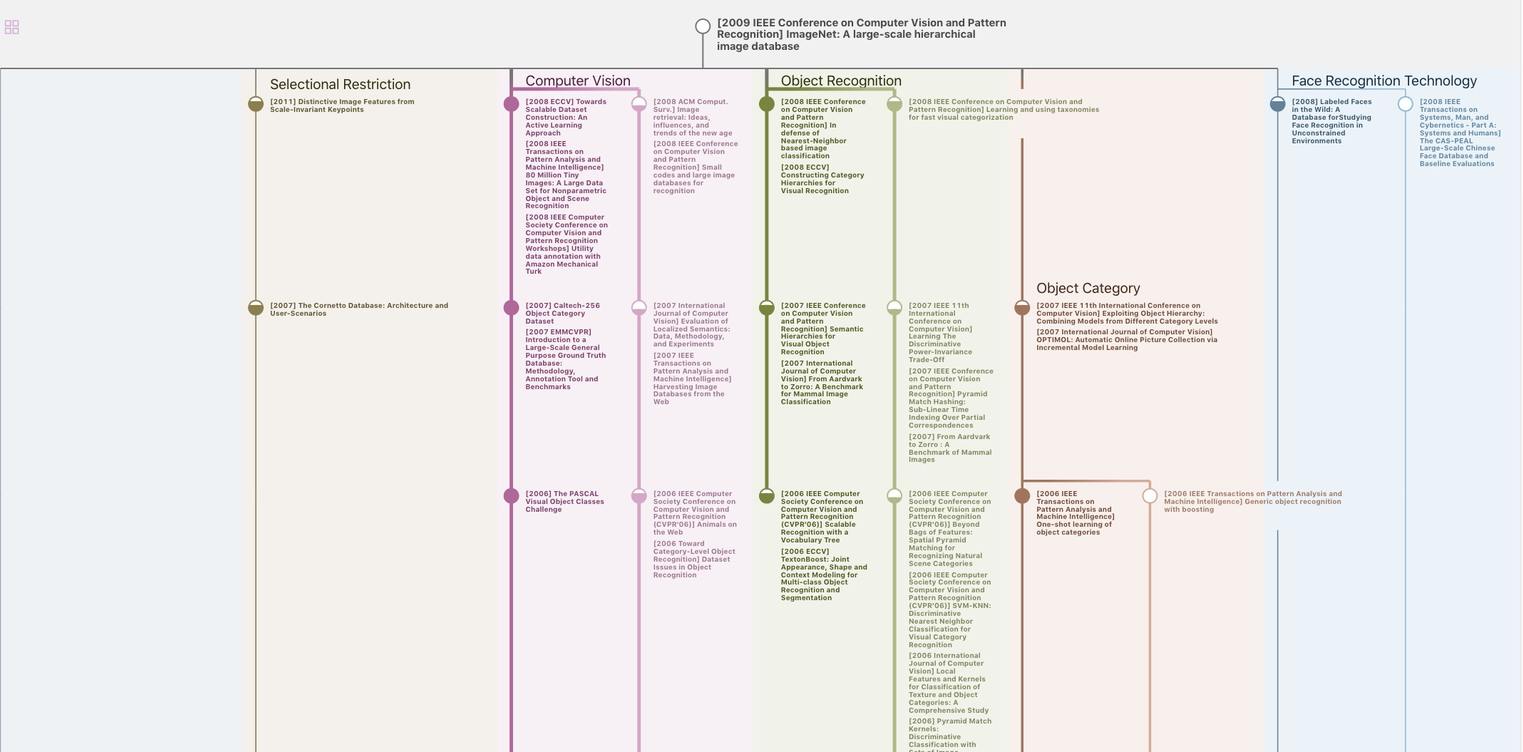
生成溯源树,研究论文发展脉络
Chat Paper
正在生成论文摘要