Differentially Private Sparse Mapping for Privacy-Preserving Cross Domain Recommendation
MM '23: Proceedings of the 31st ACM International Conference on Multimedia(2023)
摘要
Cross-Domain Recommendation (CDR) has been popularly studied for solving the data sparsity problem via leveraging rich knowledge from the auxiliary domain. Most of the current CDR models assume that user-item ratings/reviews are both accessible during the training procedure. However, it is impractical nowadays due to the strict data privacy protection policy. In this paper, we focus on the Privacy-Preserving Cross-Domain Recommendation problem (PPCDR). Although some previous approaches have investigated the problems, they always fail to effectively utilize the rating and review for user preference modeling. What is worse, they separate the processes of privacy data modeling and user-item collaborative filtering, leading to suboptimal solutions. To fill this gap, we propose the Differentially Private Sparse Mapping Recommendation model (DPSMRec), an end-to-end cross-domain recommendation framework for solving the PPCDR problem. DPSMRec includes three main modules, i.e., source embedding generation module, target rating prediction module, and differentially private sparse mapping module. Specifically, the source embedding generation module and target rating prediction module are set to exploit the ratings and review in each domain. The differentially private sparse mapping module aims to transfer knowledge among the overlapped users via Wasserstein distance privately. To obtain more accurate mapping solutions, we further propose differentially private enhanced sparse optimal transport via fused Gromov-Wasserstein distance which can consider both structure and semantic information among the users across domains. Our empirical study on Amazon and Douban datasets demonstrates that DPSMRec significantly outperforms the state-of-the-art models under the PPCDR setting.
更多查看译文
AI 理解论文
溯源树
样例
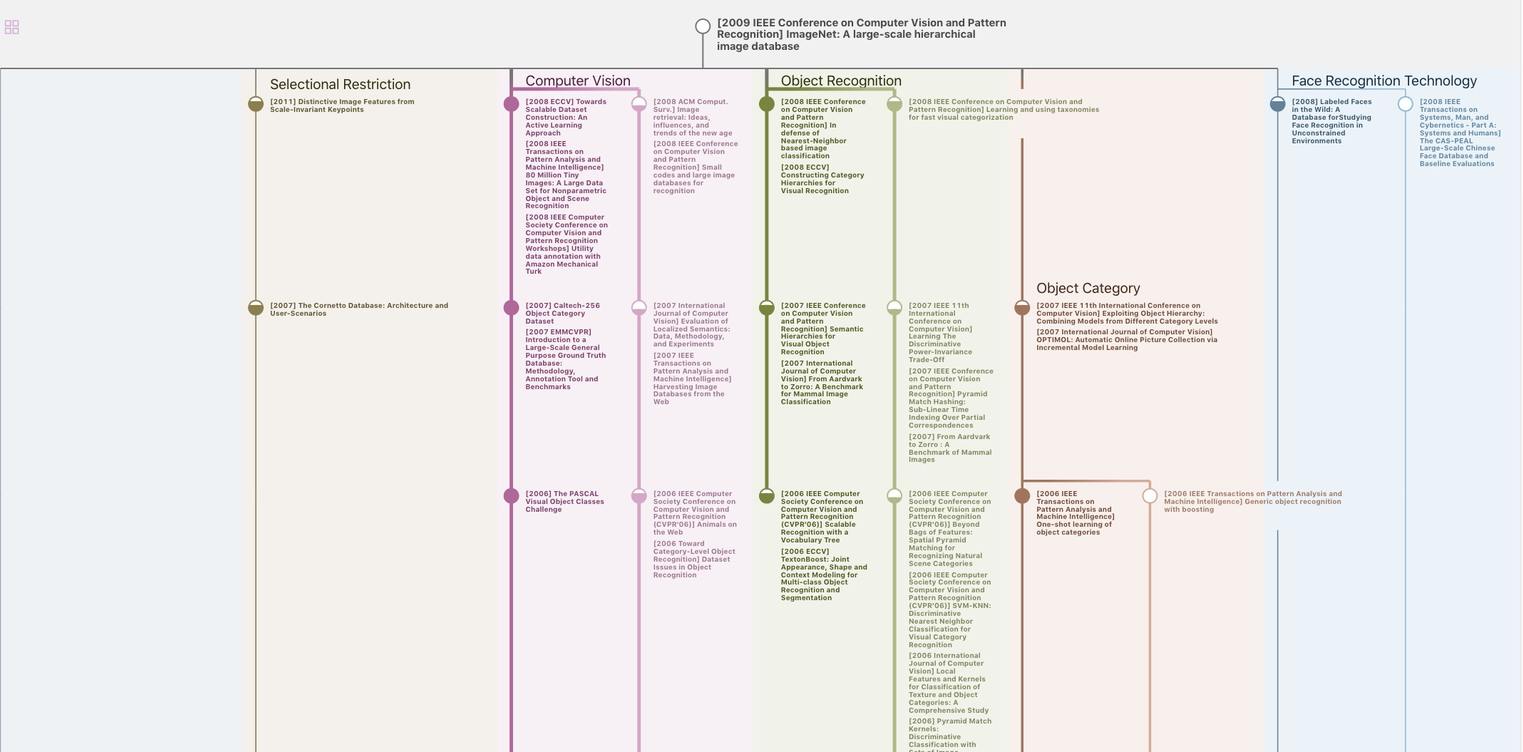
生成溯源树,研究论文发展脉络
Chat Paper
正在生成论文摘要