When Masked Image Modeling Meets Source-free Unsupervised Domain Adaptation: Dual-Level Masked Network for Semantic Segmentation
MM '23: Proceedings of the 31st ACM International Conference on Multimedia(2023)
摘要
Source-Free domain adaptive Semantic Segmentation (SFSS) aims to transfer knowledge from source domain to the target domain with only pre-trained source segmentation model and the unlabeled target dataset. Only a few works have been researched for SFSS, relying on entropy minimization, pseudo-labeling. Nevertheless, due to the domain bias, these methods tend to suffering from the confusion of classes with a similar visual appearance in different domains. To address the above issue, we propose to enhance discriminability towards target samples with masked image modeling to model spatial context relations as additional recognition clues. Specifically, we design a novel Dual-Level Masked Consistency method, which explicitly encourages the model to learn comprehensive context relations, i.e. patch-wise context and channel-wise context, on the target domain. By randomly masking target images and forcing the model to reconstruct predictions of the entire image with left unmasked part, the model has to make full use of spatially contextual information. To take a step further, we propose a novel masking strategy considering both local context and global context information by applying patch-wise masking on image patches and channel-wise masking on latent features. Notably, patch-wise context learning and channel-wise context learning can complement each other. Extensive experiments demonstrate the effectiveness of our proposed method and our method achieves state-of-the-art performance on two synthetic-to-real benchmarks: GTA5→Cityscapes and SYNTHIA→Cityscapes.
更多查看译文
AI 理解论文
溯源树
样例
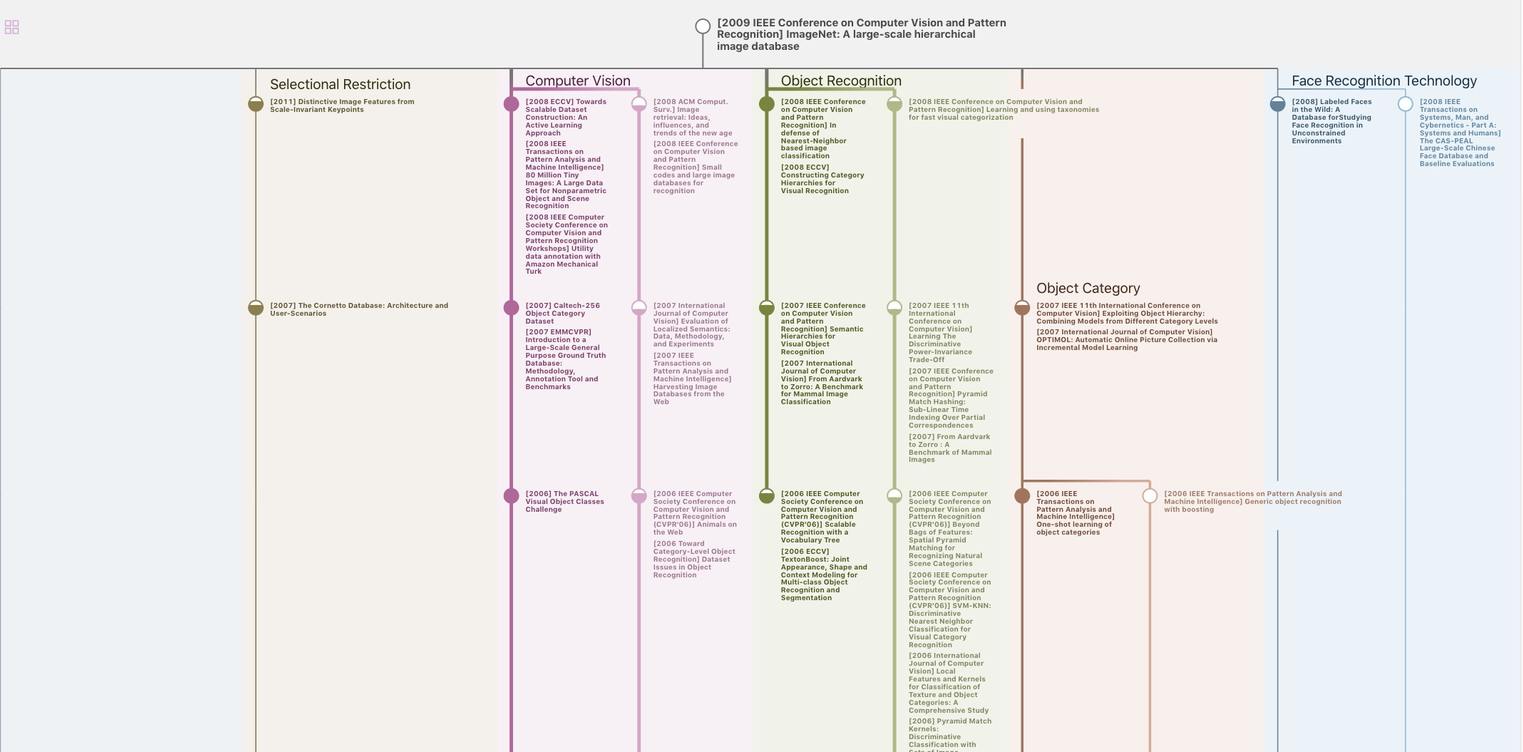
生成溯源树,研究论文发展脉络
Chat Paper
正在生成论文摘要