Efficiency-optimized Video Diffusion Models
MM '23: Proceedings of the 31st ACM International Conference on Multimedia(2023)
摘要
Video diffusion models have recently shown strong capability in synthesizing high-fidelity videos in various ways, including prediction, interpolation, and unconditional generation. However, their synthesis ability credits a lot to leveraging large denoising models to reverse the long noise-adding process, which also brings extremely expansive sampling and training costs. After examining the source of the computation cost, we confirm that the main calculation comes from the redundancy of the convolution. To address this issue, we propose Efficiency-optimized Video Diffusion Models to reduce the network's computation cost by minimizing the input and output channels of the convolution. First, a bottleneck residual pathway is proposed to conduct a channel-wise downsample to the convolution pathways, which extracts crucial information from the input and reduces computation cost. Second, a three-path channel split strategy is proposed to reduce channel redundancy by handling part of the input channels with more efficient pointwise convolution and skip-connection pathways. Furthermore, a mixed self-attention mechanism is proposed to optimize the computation cost of the self-attention in the network by adaptively choosing the algorithm with lower time complexity according to the input token lengths and hidden dimensions. Extensive experiments on three downstream tasks show that our Efficiency-optimized Video Diffusion Models can achieve a 10x speed-up while achieving comparable or even better results in the performance of fidelity compared with the state-of-the-art methods. The code is available at https://github.com/PKU-ICST-MIPL/EVDM_ACMMM2023.
更多查看译文
AI 理解论文
溯源树
样例
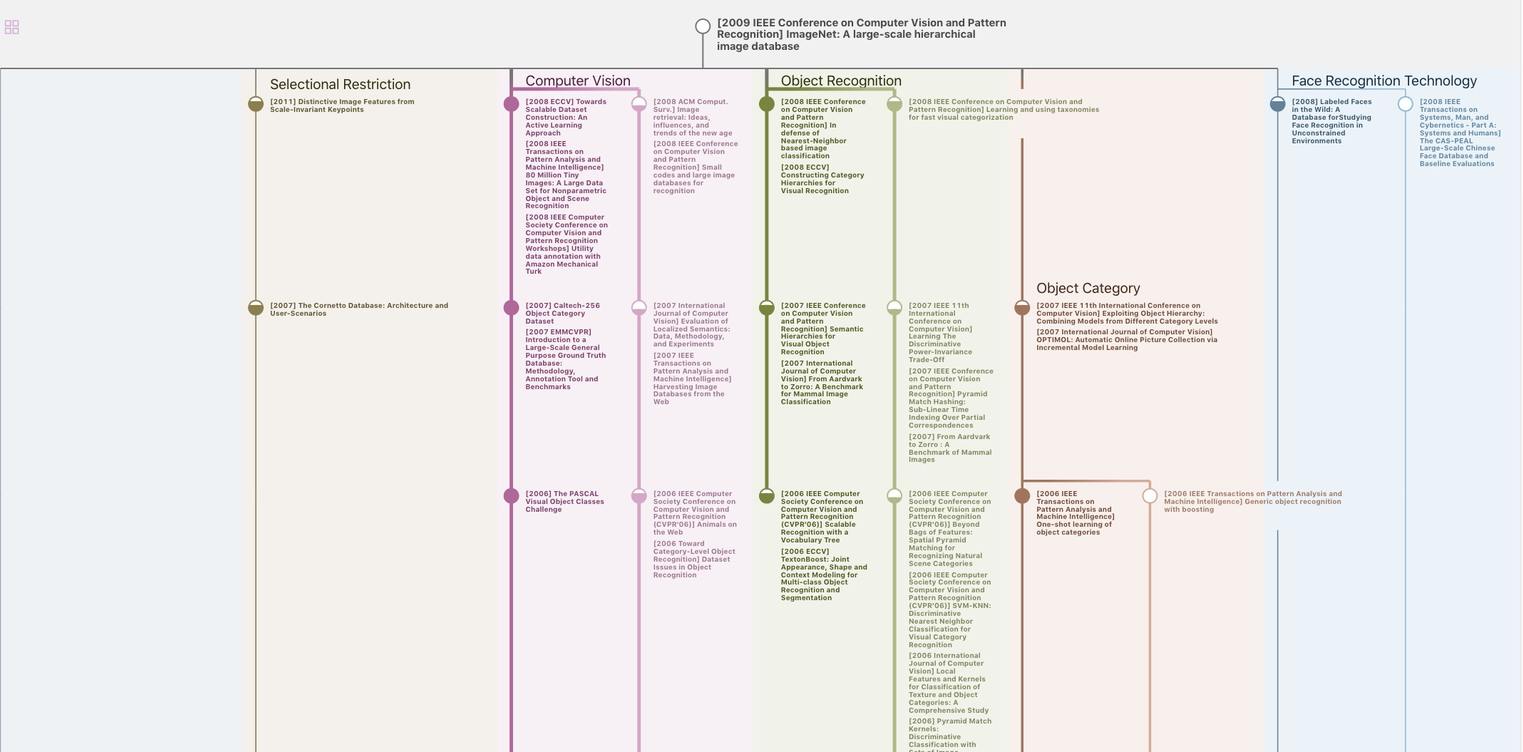
生成溯源树,研究论文发展脉络
Chat Paper
正在生成论文摘要