Failure probability function estimation in augmented sample space combined active learning Kriging and adaptive sampling by Voronoi cells
MECHANICAL SYSTEMS AND SIGNAL PROCESSING(2024)
摘要
Due to the epistemic uncertainty in engineering practice, both the random variables and their distribution parameters should be simultaneously considered uncertain. Therefore, the failure probability function (FPF) is represented as a function of distribution parameters, which can be estimated based on Bayes' rule with sampling approaches, but the accuracy and the computational burden still need to be improved. Thereafter, the calculation of FPF with an active learning Kriging model is preferable, but it needs to build one with good fitness in the entire space with the variation of sample space. To balance the global and local accuracy of the Kriging model under uncertain distribution parameters, the variation of sample space is first transformed into an augmented sample space for random variables and further divided into Voronoi cells, then the most sensitive cell is tracked adaptively to update the Kriging model. The beneficial information and a corresponding stopping condition ensure the global and local accuracy of the Kriging model. Finally, due to the significant error of the probability density function approximation methods, the FPF is estimated by point-wise prediction and interpolation technique after discretizing the distribution parameters. Two numerical examples and two engineering examples for an automotive front axle and a turbine blade demonstrate the efficiency and accuracy of the proposed method for FPF estimation. However, due to the Kriging model and Voronoi cells themselves, the method is limited to high-dimensional problems.
更多查看译文
关键词
Failure probability function,Augmented sample space,Distribution parameter,Global Kriging model,Voronoi cells
AI 理解论文
溯源树
样例
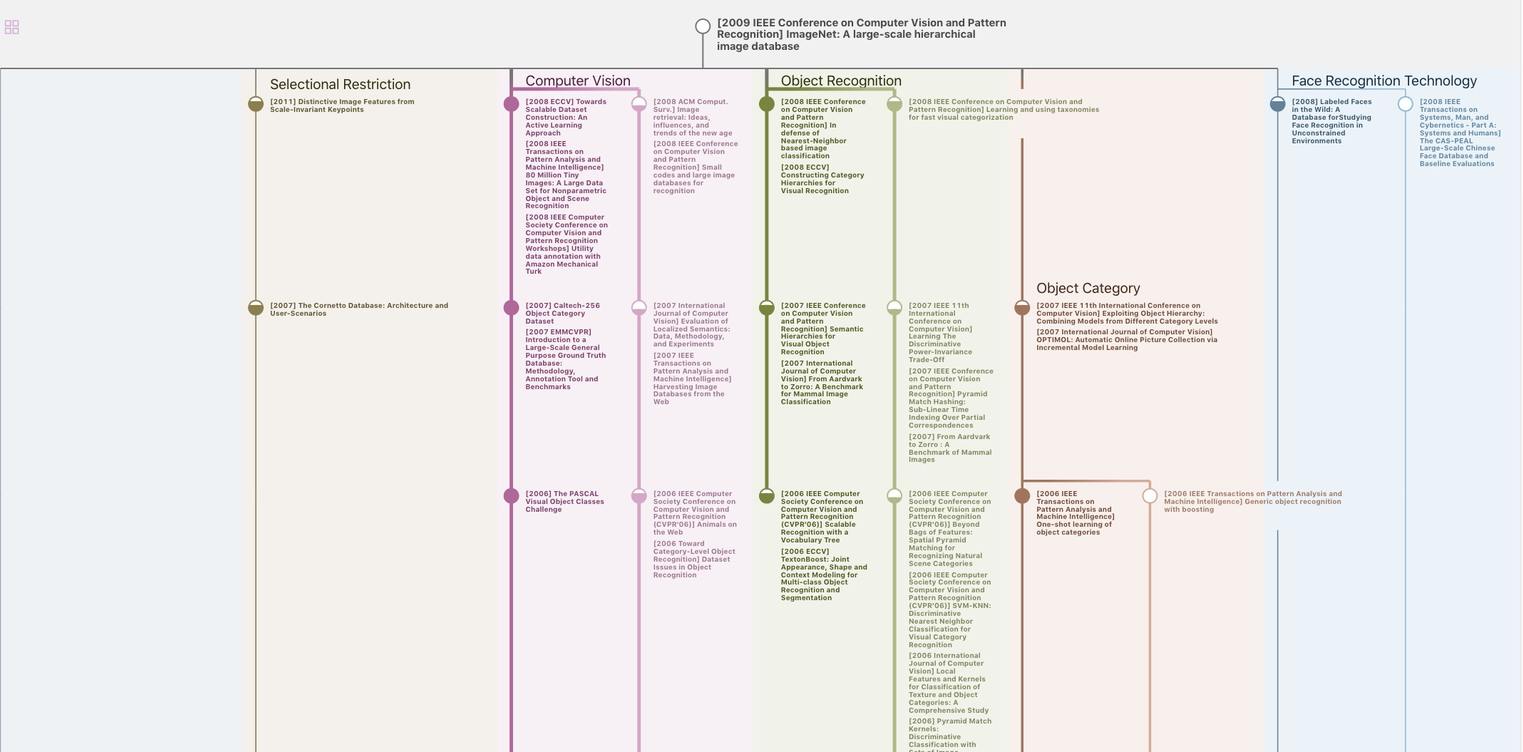
生成溯源树,研究论文发展脉络
Chat Paper
正在生成论文摘要