Let's Discover More API Relations: A Large Language Model-based AI Chain for Unsupervised API Relation Inference.
CoRR(2023)
摘要
APIs have intricate relations that can be described in text and represented
as knowledge graphs to aid software engineering tasks. Existing relation
extraction methods have limitations, such as limited API text corpus and
affected by the characteristics of the input text.To address these limitations,
we propose utilizing large language models (LLMs) (e.g., GPT-3.5) as a neural
knowledge base for API relation inference. This approach leverages the entire
Web used to pre-train LLMs as a knowledge base and is insensitive to the
context and complexity of input texts. To ensure accurate inference, we design
our analytic flow as an AI Chain with three AI modules: API FQN Parser, API
Knowledge Extractor, and API Relation Decider. The accuracy of the API FQN
parser and API Relation Decider module are 0.81 and 0.83, respectively. Using
the generative capacity of the LLM and our approach's inference capability, we
achieve an average F1 value of 0.76 under the three datasets, significantly
higher than the state-of-the-art method's average F1 value of 0.40. Compared to
CoT-based method, our AI Chain design improves the inference reliability by
67%, and the AI-crowd-intelligence strategy enhances the robustness of our
approach by 26%.
更多查看译文
关键词
more api relations,ai chain
AI 理解论文
溯源树
样例
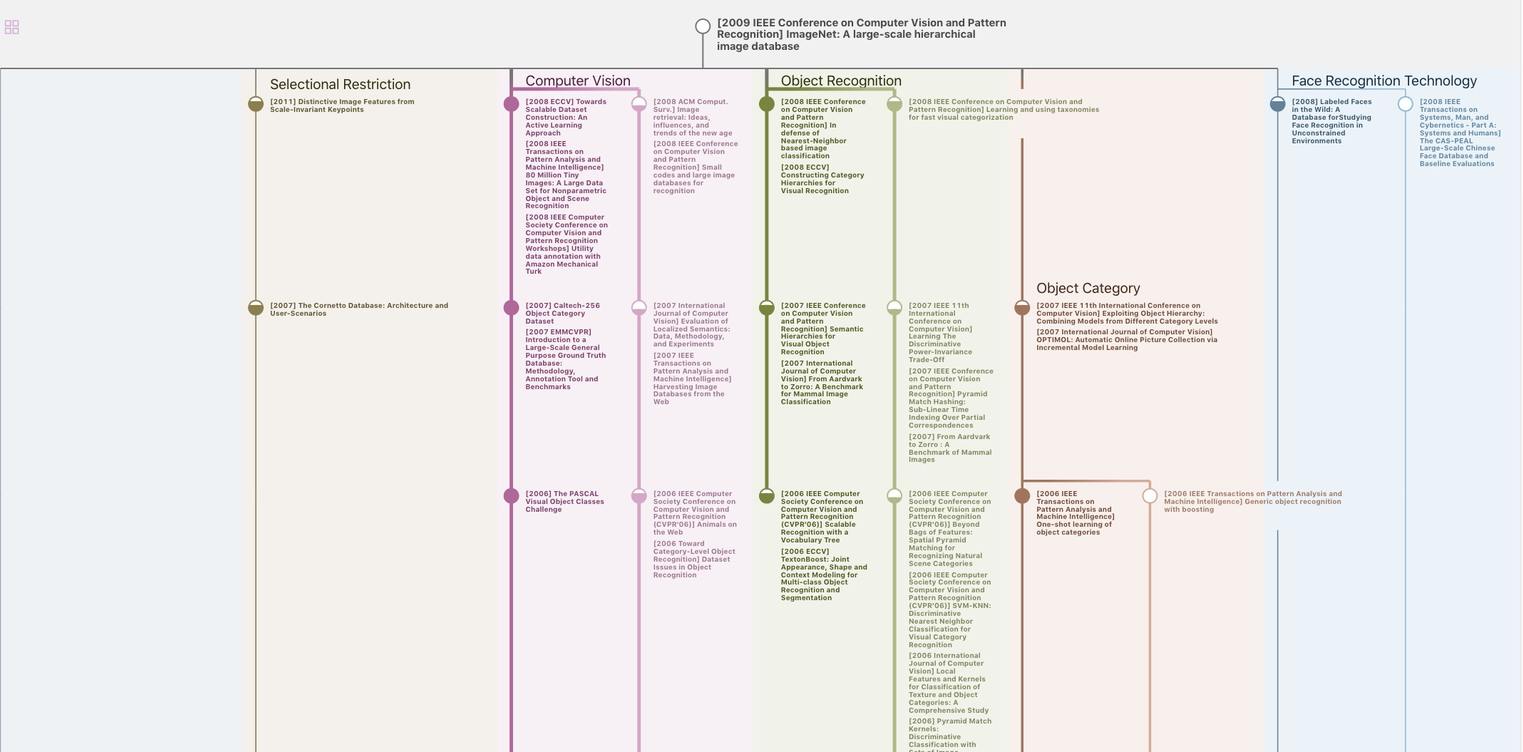
生成溯源树,研究论文发展脉络
Chat Paper
正在生成论文摘要