Autonomous Learning of Generative Models with Chemical Reaction Network Ensembles.
CoRR(2023)
摘要
Can a micron sized sack of interacting molecules autonomously learn an
internal model of a complex and fluctuating environment? We draw insights from
control theory, machine learning theory, chemical reaction network theory, and
statistical physics to develop a general architecture whereby a broad class of
chemical systems can autonomously learn complex distributions. Our construction
takes the form of a chemical implementation of machine learning's optimization
workhorse: gradient descent on the relative entropy cost function. We show how
this method can be applied to optimize any detailed balanced chemical reaction
network and that the construction is capable of using hidden units to learn
complex distributions. This result is then recast as a form of integral
feedback control. Finally, due to our use of an explicit physical model of
learning, we are able to derive thermodynamic costs and trade-offs associated
to this process.
更多查看译文
关键词
chemical reaction network
AI 理解论文
溯源树
样例
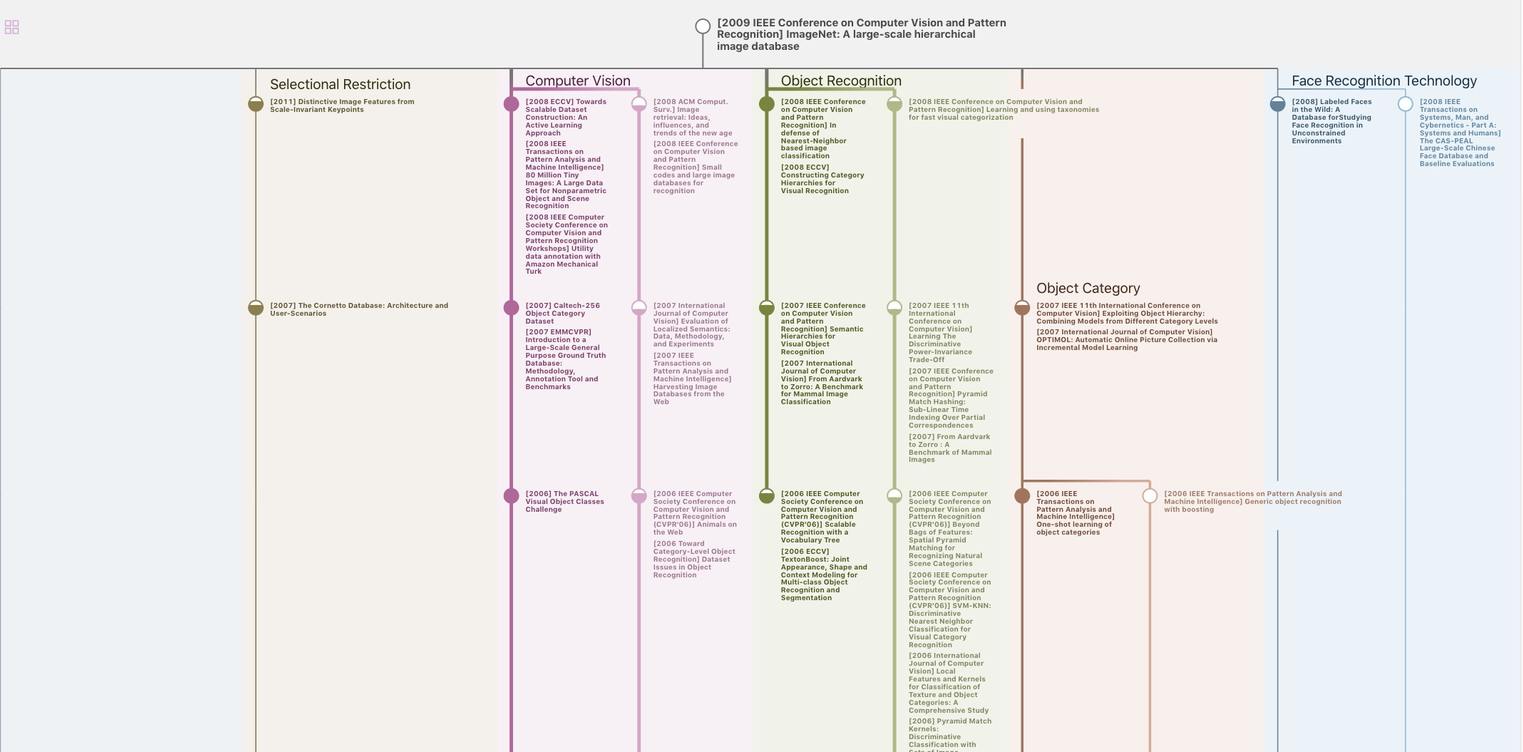
生成溯源树,研究论文发展脉络
Chat Paper
正在生成论文摘要