Neural Field Dynamics Model for Granular Object Piles Manipulation.
CoRR(2023)
摘要
We present a learning-based dynamics model for granular material
manipulation. Inspired by the Eulerian approach commonly used in fluid
dynamics, our method adopts a fully convolutional neural network that operates
on a density field-based representation of object piles and pushers, allowing
it to exploit the spatial locality of inter-object interactions as well as the
translation equivariance through convolution operations. Furthermore, our
differentiable action rendering module makes the model fully differentiable and
can be directly integrated with a gradient-based trajectory optimization
algorithm. We evaluate our model with a wide array of piles manipulation tasks
both in simulation and real-world experiments and demonstrate that it
significantly exceeds existing latent or particle-based methods in both
accuracy and computation efficiency, and exhibits zero-shot generalization
capabilities across various environments and tasks.
更多查看译文
关键词
neural field dynamics model,object
AI 理解论文
溯源树
样例
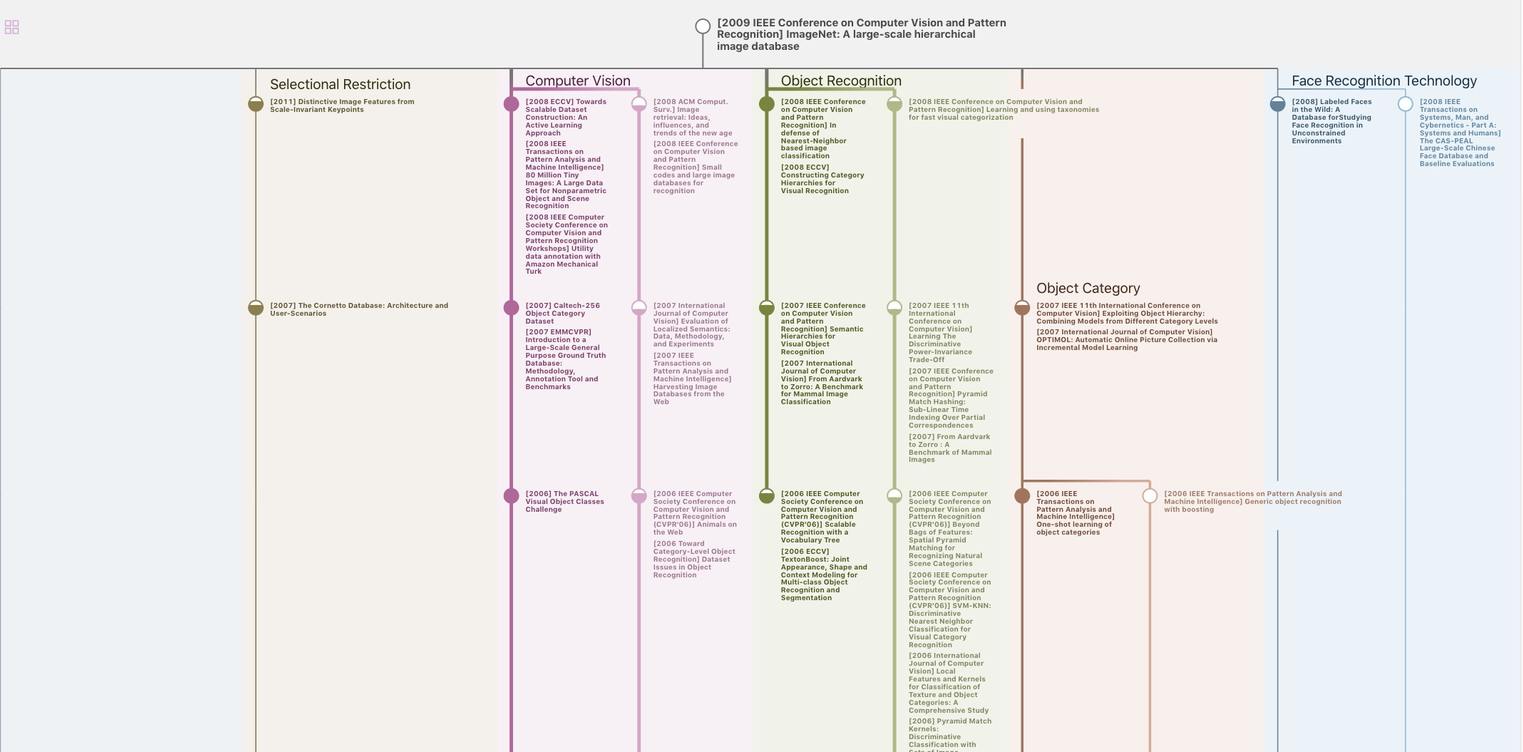
生成溯源树,研究论文发展脉络
Chat Paper
正在生成论文摘要