Neuro-Logic Learning for Relation Reasoning over Event Knowledge Graph
2023 IEEE International Conference on Intelligence and Security Informatics (ISI)(2023)
摘要
Security-related events are continuously emerging around the world, such as social unrest, armed conflicts and natural disasters. Although event knowledge graphs provide a concise and intuitive way to organize event information, they often suffer from the incompleteness problem. To solve this problem, the knowledge graph reasoning technique is usually adopted to infer new facts based on existing relations of entities. However, existing reasoning methods often concentrate on the entity relations, without considering the attributes of nodes for event knowledge graphs, such as event descriptions and event time, which contain rich information of events and are vital for event relation reasoning. Existing methods are also deficient in interpretability, which significantly benefits security-related decision-making scenarios. In this paper, we propose a Neuro-Logic Learning (NLL) method for relation reasoning over event knowledge graph. Our method first adopts different event attributes to learn the semantic representations of events, and ranks candidate event relation triples according to their similarity scores. The proposed method then develops the neural logic rule leaner to obtain interpretable reasoning rules. We construct a new large-scale event relation dataset EventKG-R based on EventKG to evaluate the effectiveness of our proposed method. Experimental results show that our proposed method achieves superior performances compared to the state-of-the-art baselines.
更多查看译文
关键词
event knowledge graph,event relation reasoning,event representation learning,neural logic rules
AI 理解论文
溯源树
样例
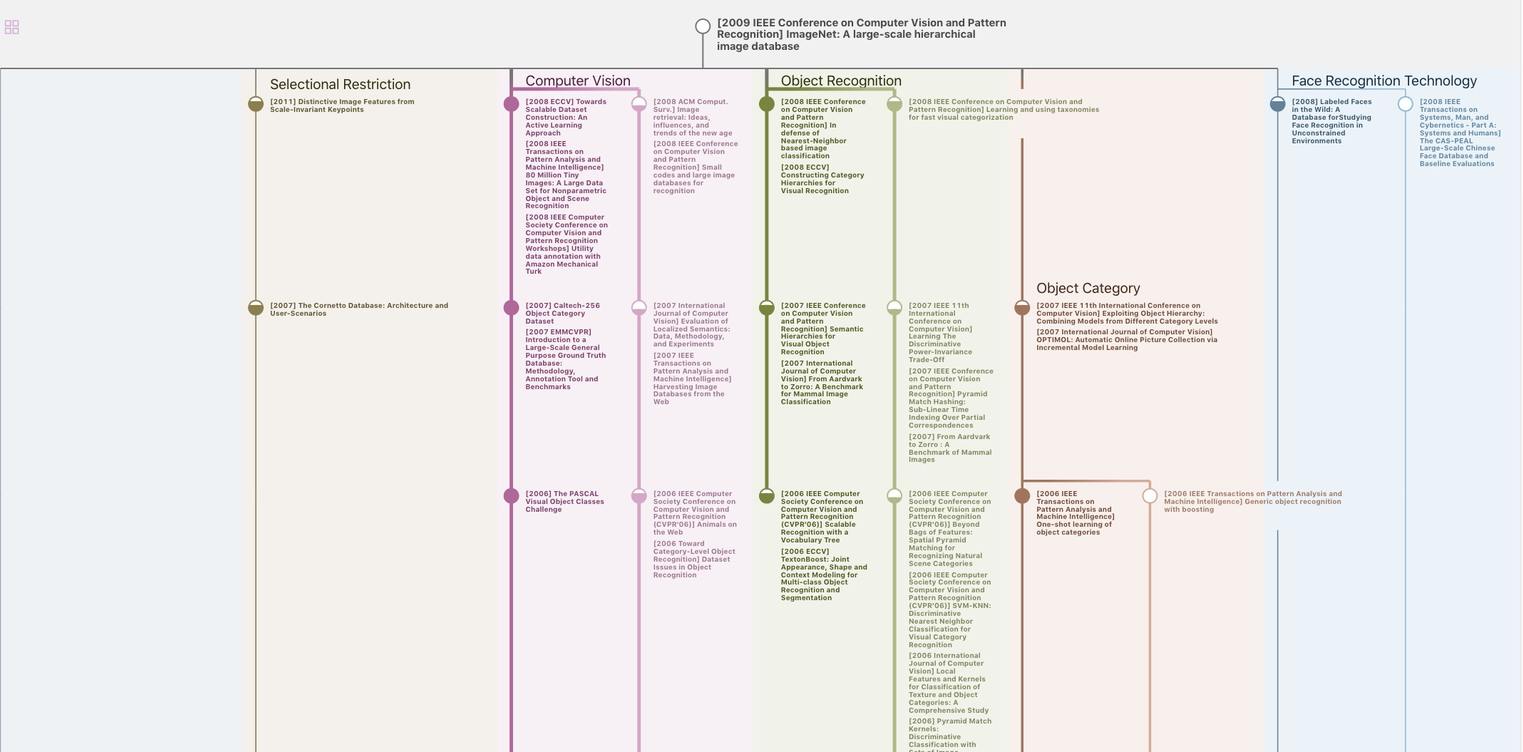
生成溯源树,研究论文发展脉络
Chat Paper
正在生成论文摘要