Active Sensing for Transformer Model in Sparse Mobile CrowdSensing
2023 IEEE 20th International Conference on Mobile Ad Hoc and Smart Systems (MASS)(2023)
摘要
With the popularization of mobile devices and the development of 5G networks, Mobile Crowd-Sensing (MCS) has emerged as a new paradigm for data collection. As its variant, Sparse MCS is favored by researchers because of its practicality and low costs, which only needs to select a few sub-areas and then infer the entire map. To achieve good performance on the complex sensing tasks, Sparse MCS has to utilize the powerful deep learning methods, e.g., Transformer, which actually has high requirements for training data sets. However, most existing works randomly select spatiotemporal positions to sense training data, which not only ignores the importance of the spatiotemporal positions but also may lead to unbalance sensing data distribution and affect the training of the model. Consequently, in this paper, we propose an active sensing method in Sparse MCS for the Transformer-like models. First, we consider the data correlation at different spatiotemporal positions and use it to evaluate the representativeness of each spatiotemporal position. Secondly, we assess the informativeness of each spatiotemporal position by using the newly proposed spatiotemporal attention mechanism. Then we use these two aspects to evaluate the importance of each spatiotemporal position. Finally, we evaluate the performance of our proposed method through two typical urban sensing tasks with three real-world datasets.
更多查看译文
关键词
Mobile crowdsensing,Active Learning,Transformer
AI 理解论文
溯源树
样例
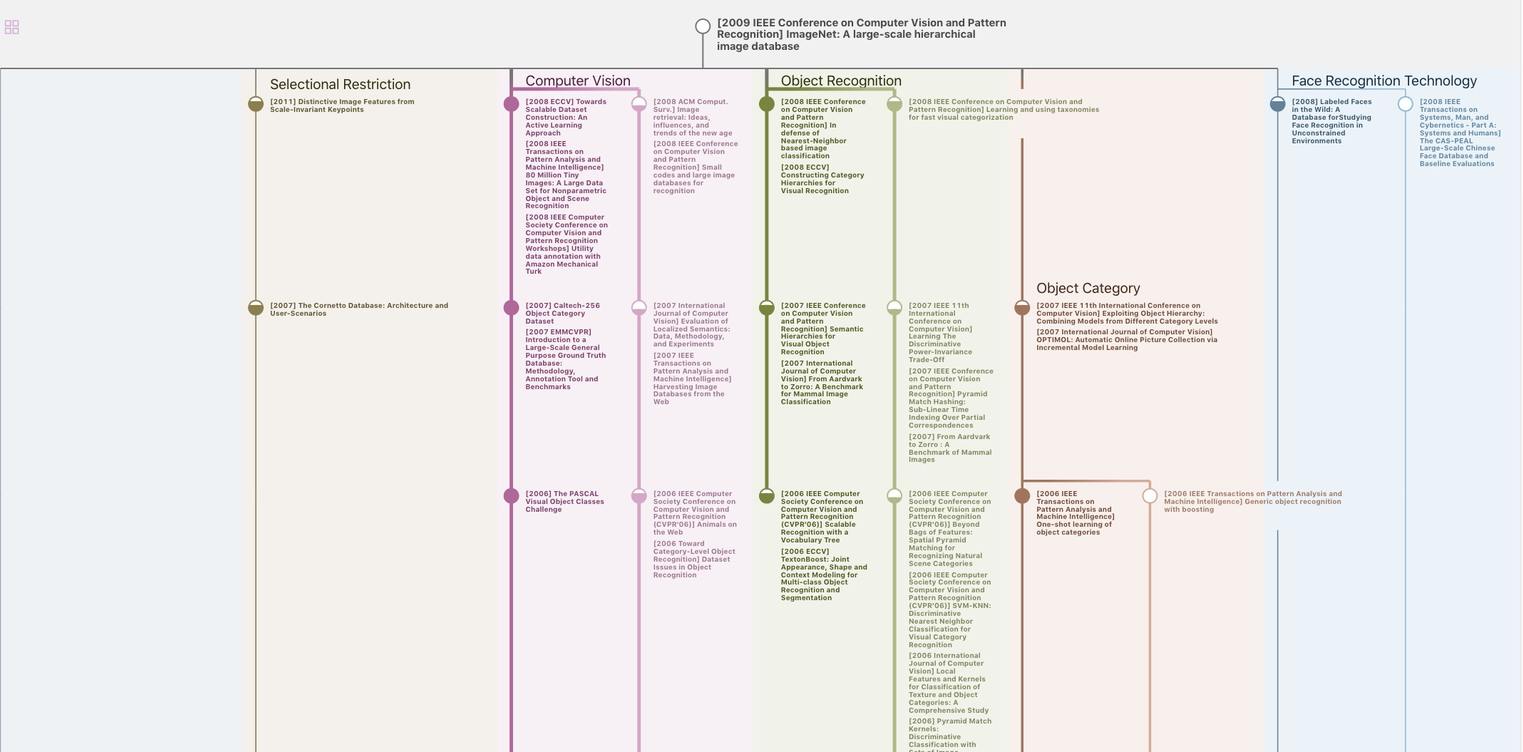
生成溯源树,研究论文发展脉络
Chat Paper
正在生成论文摘要